Mitigating the missing-fragmentation problem in de novo peptide sequencing with a two-stage graph-based deep learning model
Nature Machine Intelligence(2023)
Abstract
Novel protein discovery and immunopeptidomics depend on highly sensitive de novo peptide sequencing with tandem mass spectrometry. Despite notable improvement using deep learning models, the missing-fragmentation problem remains an important hurdle that severely degrades the performance of de novo peptide sequencing. Here we reveal that in the process of peptide prediction, missing fragmentation results in the generation of incorrect amino acids within those regions and causes error accumulation thereafter. To tackle this problem, we propose GraphNovo, a two-stage de novo peptide-sequencing algorithm based on a graph neural network. GraphNovo focuses on finding the optimal path in the first stage to guide the sequence prediction in the second stage. Our experiments demonstrate that GraphNovo mitigates the effects of missing fragmentation and outperforms the state-of-the-art de novo peptide-sequencing algorithms.
MoreTranslated text
Key words
de novo,sequencing,peptide,deep learning,deep learning model,missing-fragmentation,two-stage,graph-based
AI Read Science
Must-Reading Tree
Example
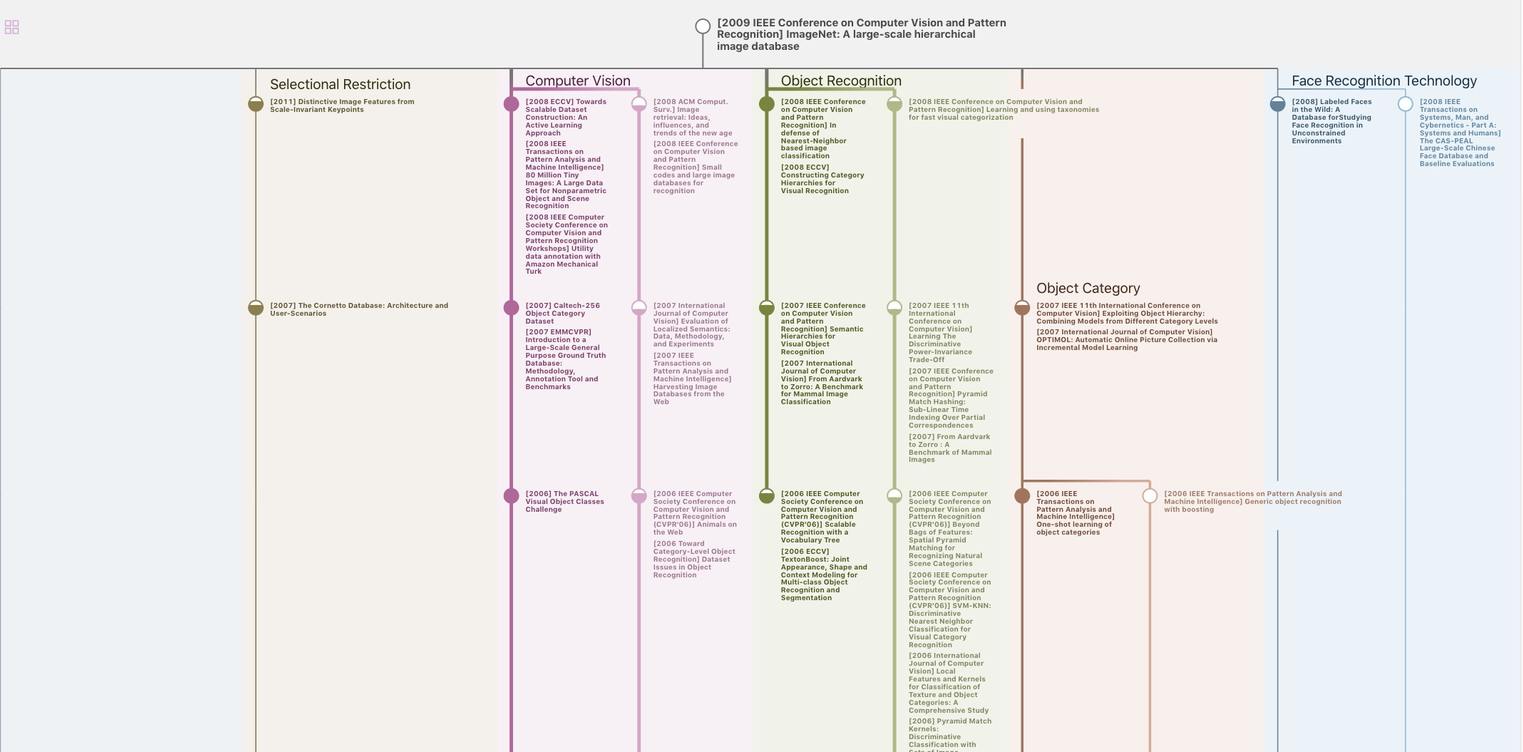
Generate MRT to find the research sequence of this paper
Chat Paper
Summary is being generated by the instructions you defined