Protein–protein contact prediction by geometric triangle-aware protein language models
Nature Machine Intelligence(2023)
摘要
Information regarding the residue–residue distance between interacting proteins is important for modelling the structures of protein complexes, as well as being valuable for understanding the molecular mechanism of protein–protein interactions. With the advent of deep learning, many methods have been developed to accurately predict the intra-protein residue–residue contacts of monomers. However, it is still challenging to accurately predict inter-protein residue–residue contacts for protein complexes, especially hetero-protein complexes. Here we develop a protein language model-based deep learning method to predict the inter-protein residue–residue contacts of protein complexes—named DeepInter—by introducing a triangle-aware mechanism of triangle update and triangle self-attention into the deep neural network. We extensively validate DeepInter on diverse test sets of 300 homodimeric, 28 CASP-CAPRI homodimeric and 99 heterodimeric complexes and compare it with state-of-the-art methods including CDPred, DeepHomo2.0, GLINTER and DeepHomo. The results demonstrate the accuracy and robustness of DeepInter.
更多查看译文
关键词
models,protein-protein,triangle-aware
AI 理解论文
溯源树
样例
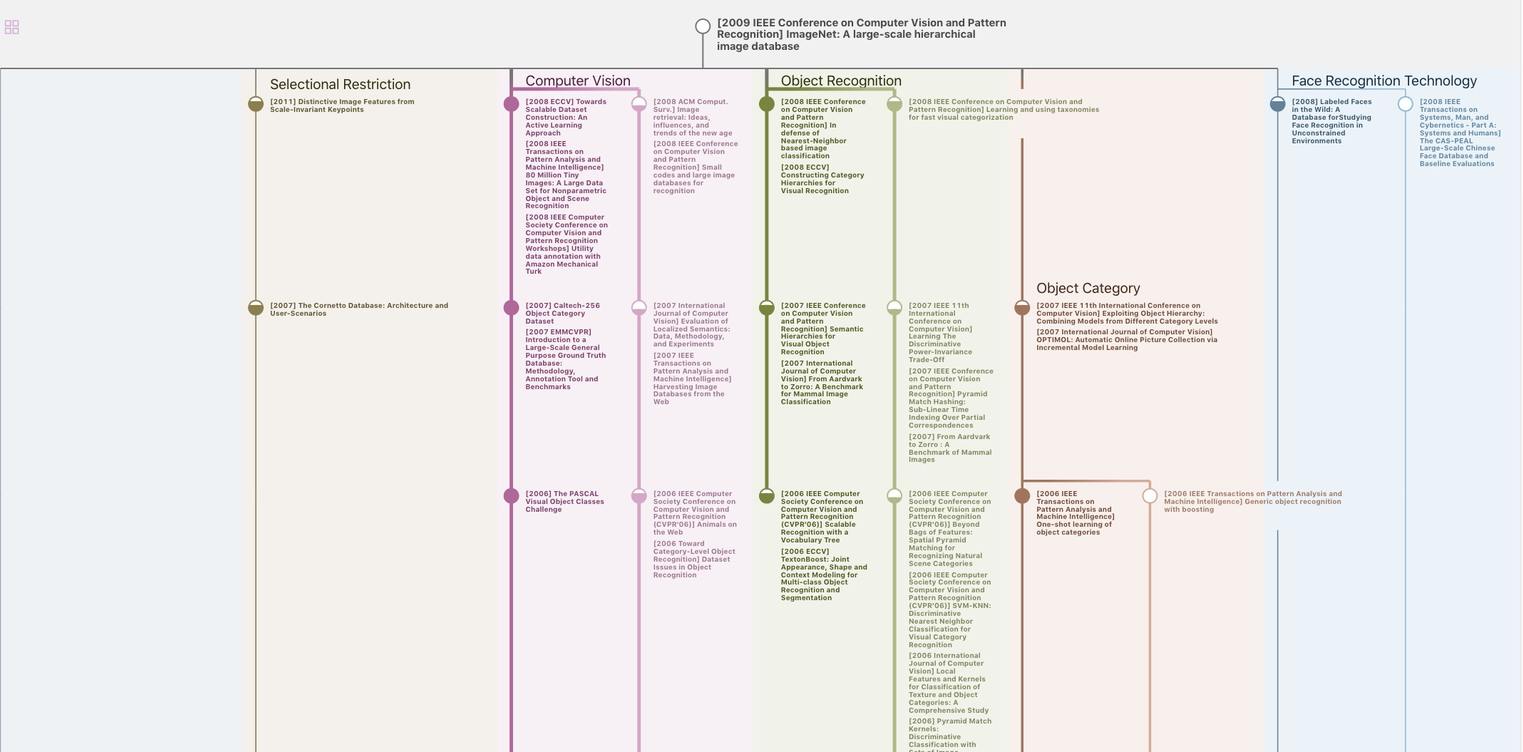
生成溯源树,研究论文发展脉络
Chat Paper
正在生成论文摘要