Machine learning reveals the effects of drivers on PM2.5 and CO2 based on ensemble source apportionment method
Atmospheric Research(2023)
摘要
Air pollution and climate change are currently common global concern issues. Understanding the main pollution sources of fine particulate matter (PM2.5) and carbon dioxide (CO2), and the drivers that accelerate their concentrations can provide novel insights into the co-benefits of air-quality improvement and climate change mitigation. Hence, in this study, we conducted source apportionment of PM2.5 and CO2, and obtained impacts of common sources on PM2.5-CO2 using an ensemble source apportionment method. Then, machine learning (ML) methods were utilized to study the effects of different drivers (including main pollution sources and meteorological factors) on PM2.5 and CO2. The results demonstrate that coal combustion (40%), vehicle exhaust (37%), and industrial emissions (23%) were the main common sources of PM2.5 and CO2. Controlling the combustion of fossil fuels and long-term vehicle electrification still hold significant importance for reducing pollutions and carbon emissions. The results of data-driven ML models show that temperature had the highest effect among meteorological factors, reflecting the seasonal changes of CO2 and PM2.5. The study provides a feasible framework for exploring co-benefits of clean air policies on greenhouse gas emissions based on online observation dataset.
更多查看译文
关键词
Ensemble source apportionment,Machine learning,Carbon dioxide,Particulate matter,Coordinated control
AI 理解论文
溯源树
样例
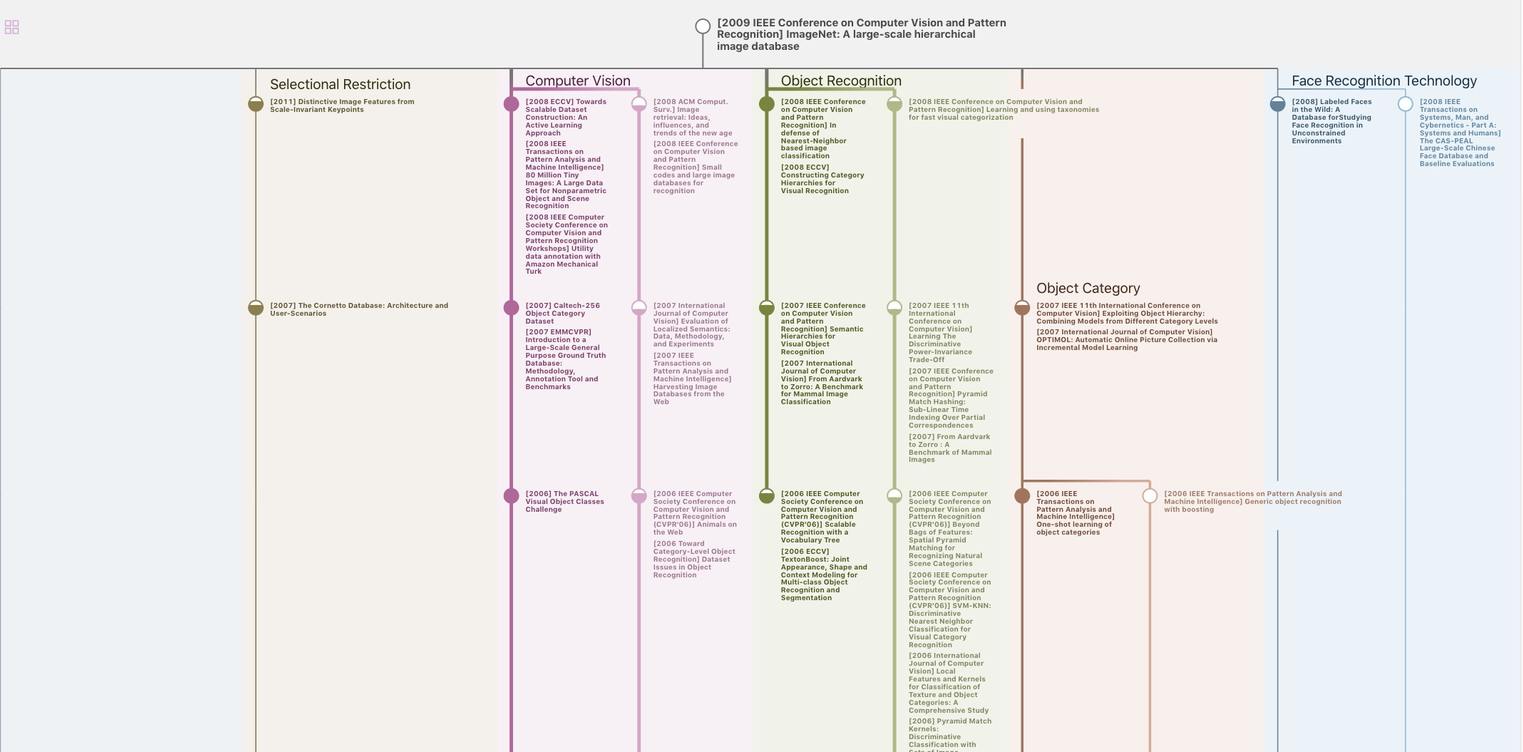
生成溯源树,研究论文发展脉络
Chat Paper
正在生成论文摘要