A University Student Performance Prediction Model and Experiment Based on Multi-Feature Fusion and Attention Mechanism
IEEE Access(2023)
Abstract
Predicting student performance is a crucial research area in educational data mining. Student grades are influenced by various factors such as past academic performance, family background, and personal achievements. However, it is difficult to collect many relevant factors other than past academic performance, and it is not ideal to predict student performance using past academic records alone. Therefore, reducing the difficulty of data collection while maintaining timeliness and prediction accuracy remains a pressing issue. To address this challenge, this study proposes a novel model for predicting university student performance based on multi-feature fusion and attention mechanisms. The proposed model focuses on analyzing historical academic grades from multiple dimensions among university students to extract features that reveal relationships between courses and students, among different students themselves, or even between courses themselves. Additionally, an attention mechanism is introduced to explore the relationship between different dimensional features. This study collected a triplet set of related courses and students’ real historical grades, proved the correlation between courses through data analysis, and verified the effectiveness of different dimensional features. Experimental results show that, compared with traditional machine learning methods, our proposed method achieves better prediction accuracy with a precision rate of 72.5%.
MoreTranslated text
Key words
Education data mining, student performance prediction, multi-feature fusion, attention mechanism
AI Read Science
Must-Reading Tree
Example
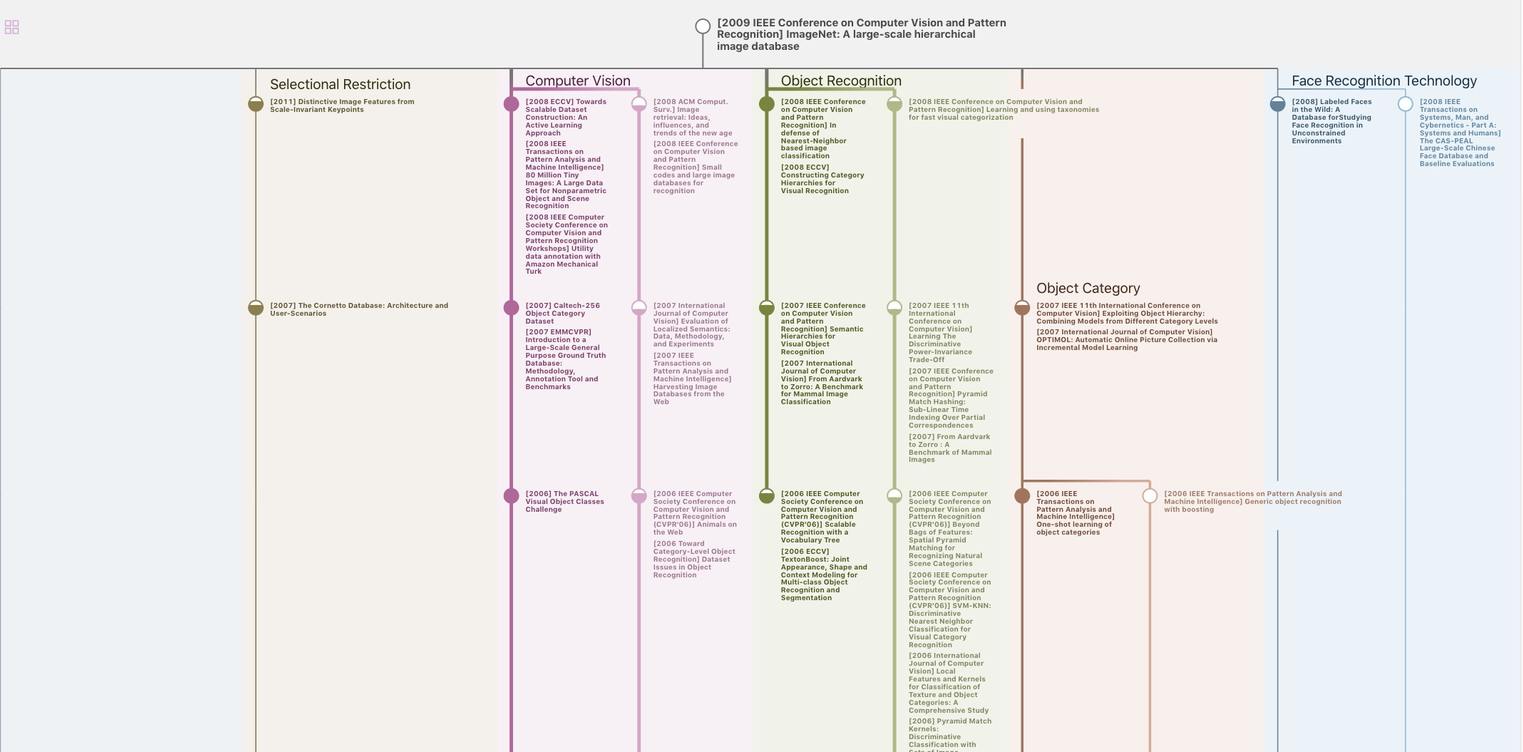
Generate MRT to find the research sequence of this paper
Chat Paper
Summary is being generated by the instructions you defined