Multi-View Predicate Recognition for Solving Semantic Ambiguity Problem in Scene Graph Generation
PROCEEDINGS OF THE 1ST INTERNATIONAL WORKSHOP ON MULTIMEDIA CONTENT GENERATION AND EVALUATION, MCGE 2023: New Methods and Practice(2023)
摘要
Recent works on Scene Graph Generation (SGG) have been concentrating on solving the problem of long-tailed distribution. While these methods are making significant improvements on the tail predicate categories, they sacrifice the performance of the head ones severely. The major issue lies in the semantic ambiguity problem, which is the contradiction between the commonly used criterion and the nature of relationships in the SGG datasets. The models are evaluated with graph constraint, which allows merely one relationship between a pair of objects. However, the relationships are much more complex and can always be described from different views. For example, when a man is in front of a computer, we can also say he is watching it. Both options are plausible, describing the different aspects of the relationship. Which of them is determined to be the ground-truth is highly subjective. In this paper, we claim that the relationships should be considered from multiple views to avoid the semantic ambiguity. In other words, the model should provide all the possibilities, rather than being biased to any one of the options. To this end, we propose the Multi-View Predicate Recognition (MVPR), which separates the label set into multiple views and enables the model to represent and predict in a "multi-view" style. Specifically, MVPR can be divided into three parts: Adaptive Bounding Box for Predicate is proposed to help the model attend to the crucial areas for the predicate categories in different views; Multi-View Predicate Feature Learning is designed to separate the feature space of different views of predicate categories; Multi-View Predicate Prediction and Multi-View Graph Constraint are used to allow the model to provide multiview predictions to accurately estimate ambiguous relationships. Experimental results on the Visual Genome dataset show that our MVPR can significantly improve the model performance on the SGG task, and achieves a new state-of-the-art.
更多查看译文
关键词
Scene graph generation,long-tailed distribution,multi-view,semantic ambiguity
AI 理解论文
溯源树
样例
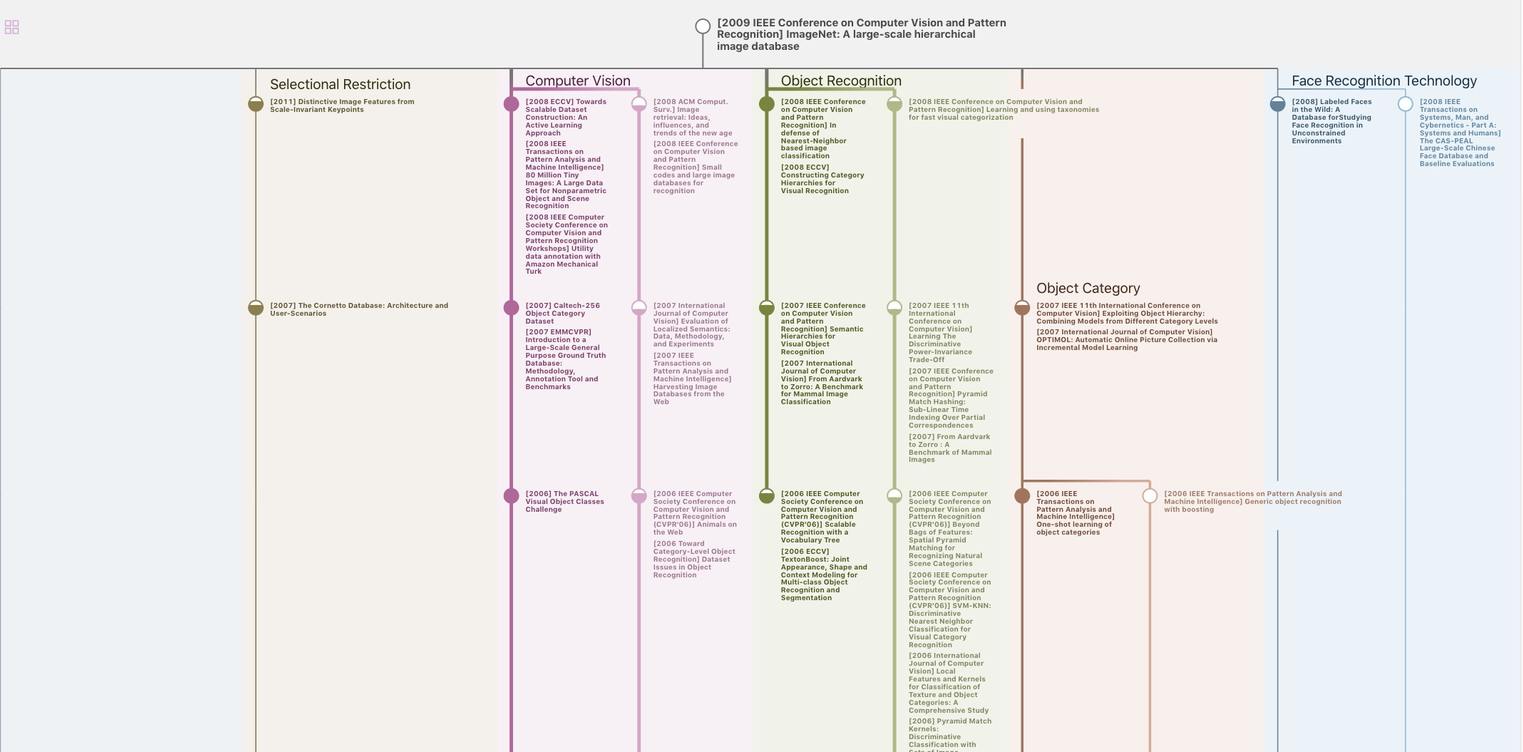
生成溯源树,研究论文发展脉络
Chat Paper
正在生成论文摘要