Twice attention networks for synthetic speech detection
Neurocomputing(2023)
摘要
Automatic speaker verification (ASV) systems are highly vulnerable to synthetic speech attack. And the artifacts are the key spoofing clue to distinguish real and synthetic speech. In this paper, we focus on the detection of artifacts and proposed the twice attention networks (TA-networks). It is an end-to-end network which consists of feature extraction module and back-end classifier. The feature extraction module is the core of the TA-networks, and it is a twice attention Unet (TA-Unet). It contains two sequential attention modules: (1) a five-layer U-shaped network with attention gate to first obtain the general contour of artifacts and then (2) a softmax-based filter with adaptive coefficient to dynamically highlight the differences between different frequencies, and these differences can be regarded as elaborate artifacts. After the processing of the TA-Unet, the feature maps of real and synthetic speech are more discriminative for the back-end SCG-Res2Net50 classifier. Experimental results show that the TA-networks achieve equal error rates of 1.62% on ASVspoof 2019 logical access sub-challenge, and it is significantly better than most of the other experimental models.
更多查看译文
关键词
Synthetic speech detection,Twice attention networks,Twice attention unet,Artifacts
AI 理解论文
溯源树
样例
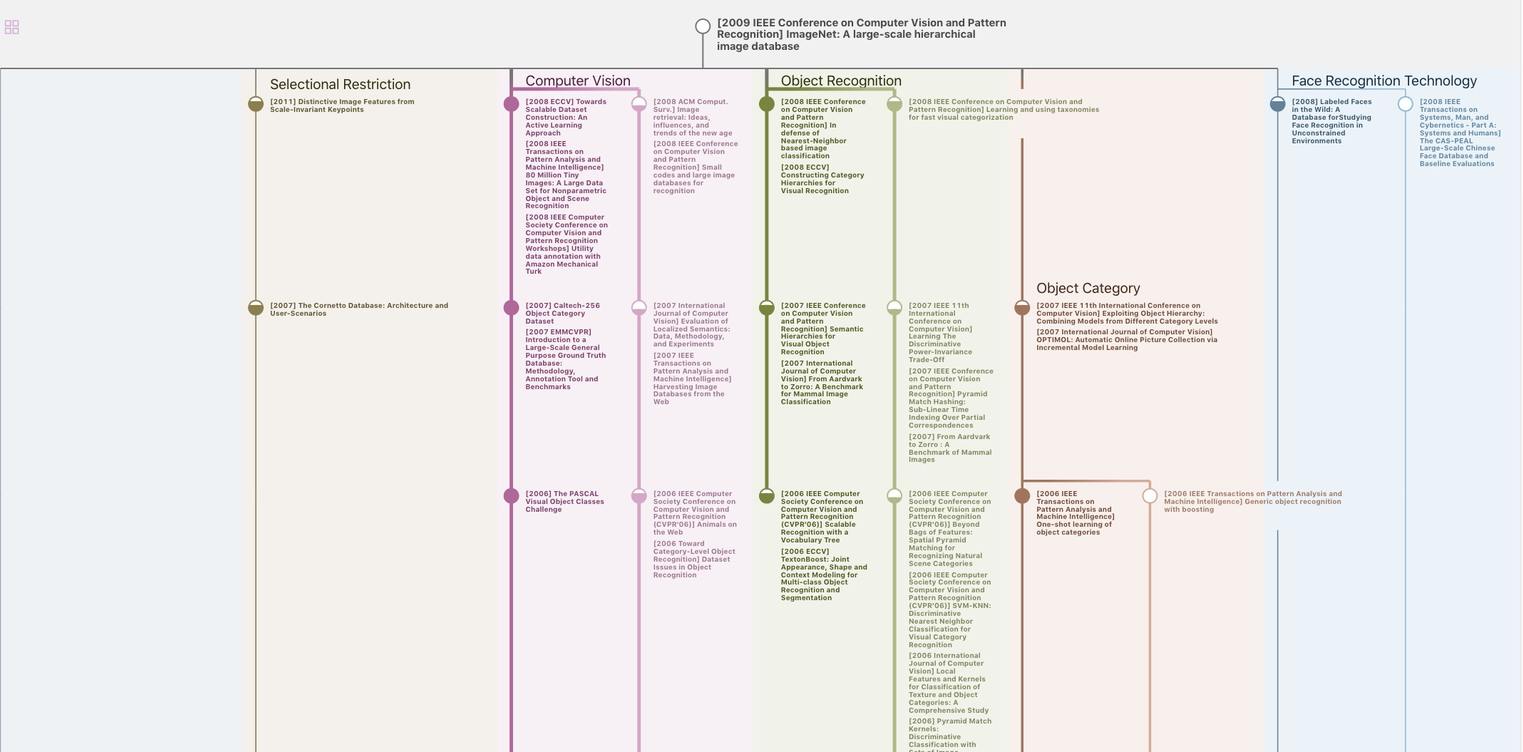
生成溯源树,研究论文发展脉络
Chat Paper
正在生成论文摘要