Deep learning based on Transformer architecture for power system short-term voltage stability assessment with class imbalance
RENEWABLE & SUSTAINABLE ENERGY REVIEWS(2024)
摘要
Most existing data-driven power system short-term voltage stability assessment (STVSA) approaches presume class-balanced input data. However, in practical applications, the occurrence of short-term voltage instability following a disturbance is minimal, leading to a significant class imbalance problem and a consequent decline in classifier performance. This work proposes a Transformer-based STVSA method to address this challenge. By utilizing the basic Transformer architecture, a stability assessment Transformer (StaaT) is developed as a classification model to reflect the correlation between the operational states of the system and the resulting stability outcomes. To combat the negative impact of imbalanced datasets, this work employs a conditional Wasserstein generative adversarial network with gradient penalty (CWGAN-GP) for synthetic data generation, aiding in the creation of a balanced, representative training set for the classifier. Semi-supervised clustering learning is implemented to enhance clustering quality, addressing the lack of a unified quantitative criterion for short-term voltage stability. Numerical tests on the IEEE 39-bus test system extensively demonstrate that the proposed method exhibits robust performance under class imbalances up to 100:1 and noisy environments, and maintains consistent effectiveness even with an increased penetration of renewable energy. Comparative results reveal that the CWGAN-GP generates more balanced datasets than traditional oversampling methods and that the StaaT outperforms other deep learning algorithms. This study presents a compelling solution for real-world STVSA applications that often face class imbalance and data noise challenges.
更多查看译文
关键词
Power system,Short-term voltage stability assessment,Transformer architecture,Class imbalance,Renewable energy penetration,Conditional wasserstein generative adversarial,network with gradient penalty (CWGAN-GP)
AI 理解论文
溯源树
样例
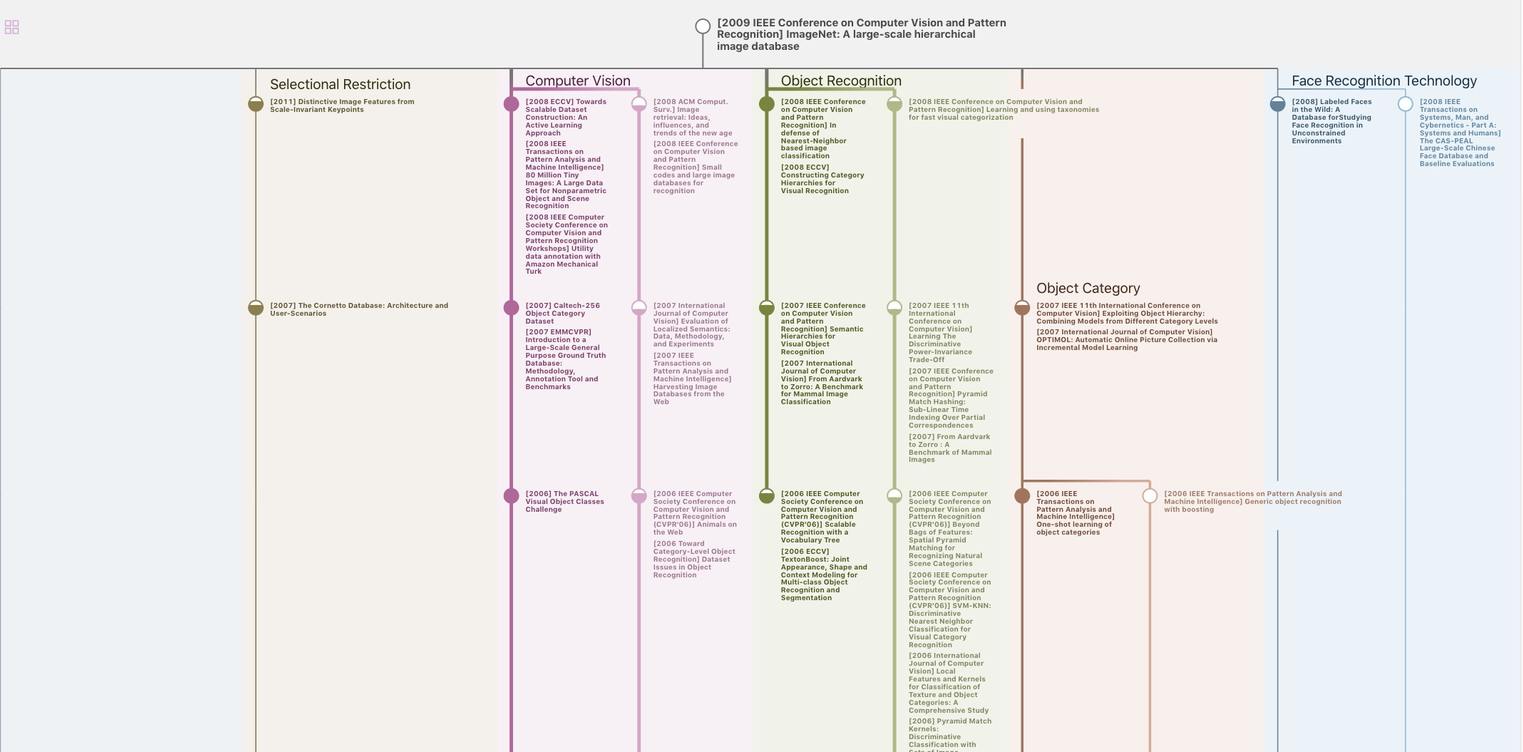
生成溯源树,研究论文发展脉络
Chat Paper
正在生成论文摘要