RekomGNN: Visualizing, Contextualizing and Evaluating Graph Neural Networks Recommendations
CoRR(2023)
摘要
Content recommendation tasks increasingly use Graph Neural Networks, but it remains challenging for machine learning experts to assess the quality of their outputs. Visualization systems for GNNs that could support this interrogation are few. Moreover, those that do exist focus primarily on exposing GNN architectures for tuning and prediction tasks and do not address the challenges of recommendation tasks. We developed RekomGNN, a visual analytics system that supports ML experts in exploring GNN recommendations across several dimensions and making annotations about their quality. RekomGNN straddles the design space between Neural Network and recommender system visualization to arrive at a set of encoding and interaction choices for recommendation tasks. We found that RekomGNN helps experts make qualitative assessments of the GNN's results, which they can use for model refinement. Overall, our contributions and findings add to the growing understanding of visualizing GNNs for increasingly complex tasks.
更多查看译文
关键词
networks recommendations,evaluating graph neural
AI 理解论文
溯源树
样例
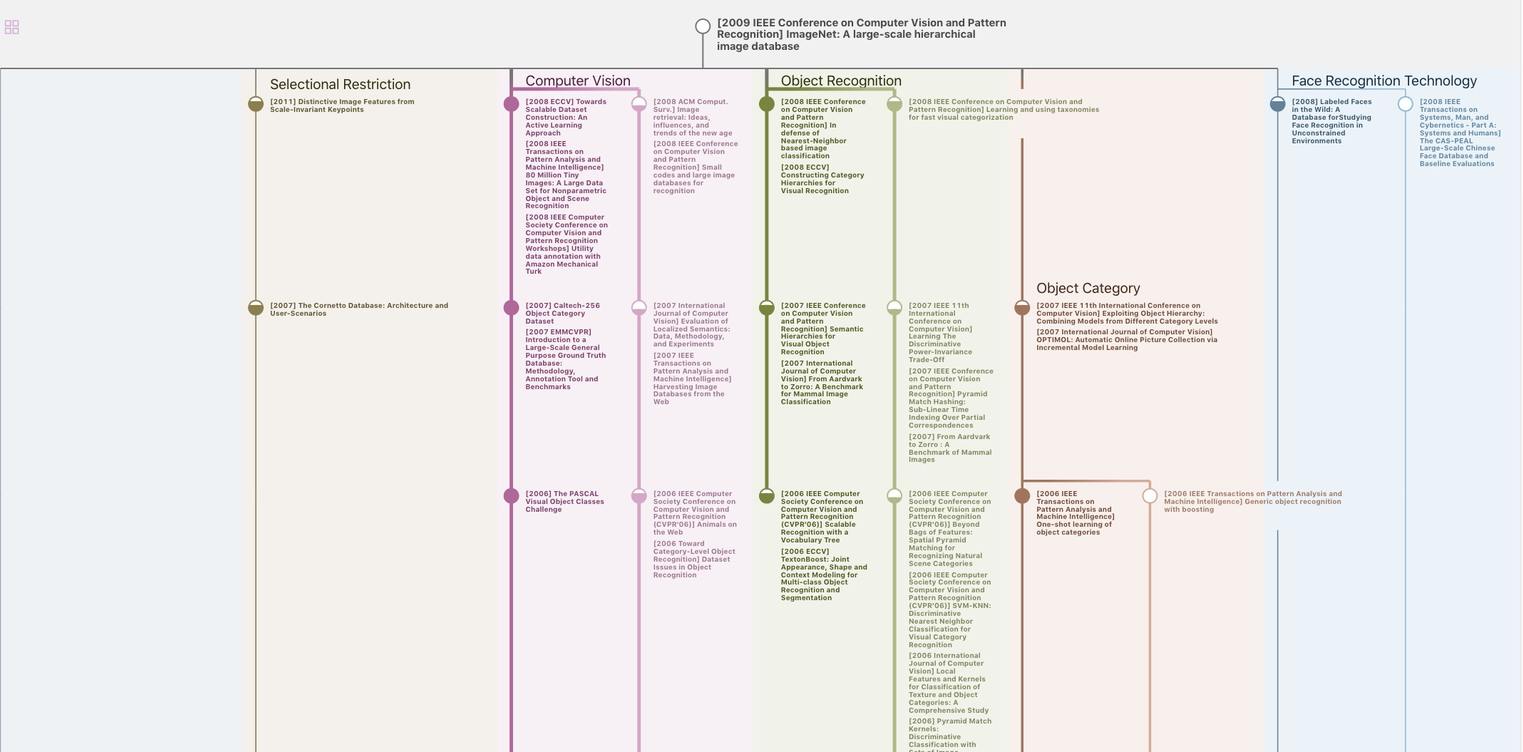
生成溯源树,研究论文发展脉络
Chat Paper
正在生成论文摘要