Robust-MBFD: A Robust Deep Learning System for Motor Bearing Faults Detection Using Multiple Deep Learning Training Strategies and A Novel Double Loss Function
CoRR(2023)
摘要
This paper presents a comprehensive analysis of motor bearing fault detection (MBFD), which involves the task of identifying faults in a motor bearing based on its vibration. To this end, we first propose and evaluate various machine learning based systems for the MBFD task. Furthermore, we propose three deep learning based systems for the MBFD task, each of which explores one of the following training strategies: supervised learning, semi-supervised learning, and unsupervised learning. The proposed machine learning based systems and deep learning based systems are evaluated, compared, and then they are used to identify the best model for the MBFD task. We conducted extensive experiments on various benchmark datasets of motor bearing faults, including those from the American Society for Mechanical Failure Prevention Technology (MFPT), Case Western Reserve University Bearing Center (CWRU), and the Condition Monitoring of Bearing Damage in Electromechanical Drive Systems from Paderborn University (PU). The experimental results on different datasets highlight two main contributions of this study. First, we prove that deep learning based systems are more effective than machine learning based systems for the MBFD task. Second, we achieve a robust and general deep learning based system with a novel loss function for the MBFD task on several benchmark datasets, demonstrating its potential for real-life MBFD applications.
更多查看译文
关键词
motor bearing faults
AI 理解论文
溯源树
样例
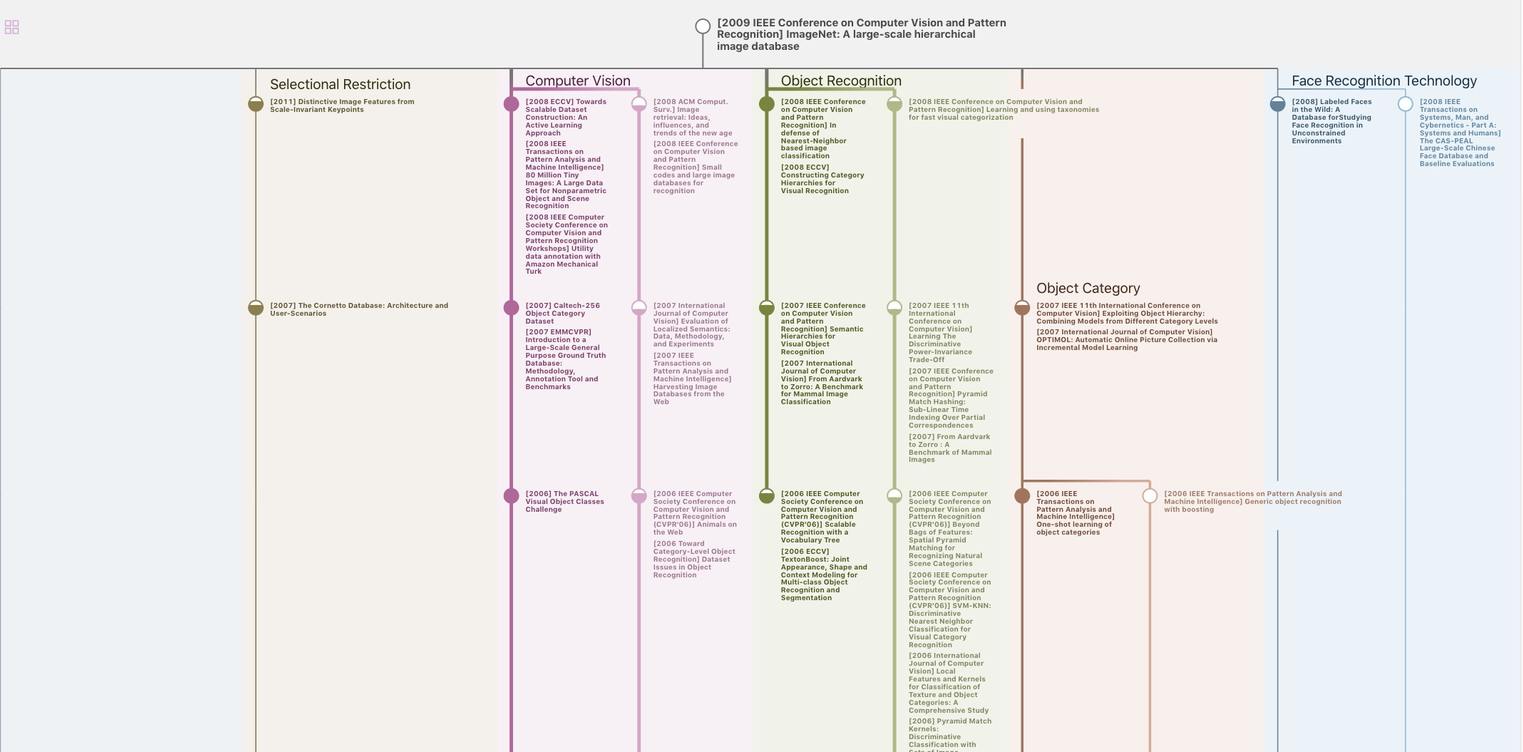
生成溯源树,研究论文发展脉络
Chat Paper
正在生成论文摘要