Protein 3D Graph Structure Learning for Robust Structure-Based Protein Property Prediction
AAAI 2024(2024)
摘要
Protein structure-based property prediction has emerged as a promising approach for various biological tasks, such as protein function prediction and sub-cellular location estimation. The existing methods highly rely on experimental protein structure data and fail in scenarios where these data are unavailable. Predicted protein structures from AI tools (e.g., AlphaFold2) were utilized as alternatives. However, we observed that current practices, which simply employ accurately predicted structures during inference, suffer from notable degradation in prediction accuracy. While similar phenomena have been extensively studied in general fields (e.g., Computer Vision) as model robustness, their impact on protein property prediction remains unexplored. In this paper, we first investigate the reason behind the performance decrease when utilizing predicted structures, attributing it to the structure embedding bias from the perspective of structure representation learning. To study this problem, we identify a Protein 3D Graph Structure Learning Problem for Robust Protein Property Prediction (PGSL-RP3), collect benchmark datasets, and present a protein Structure embedding Alignment Optimization framework (SAO) to mitigate the problem of structure embedding bias between the predicted and experimental protein structures. Extensive experiments have shown that our framework is model-agnostic and effective in improving the property prediction of both predicted structures and experimental structures.
更多查看译文
关键词
ML: Applications,APP: Natural Sciences
AI 理解论文
溯源树
样例
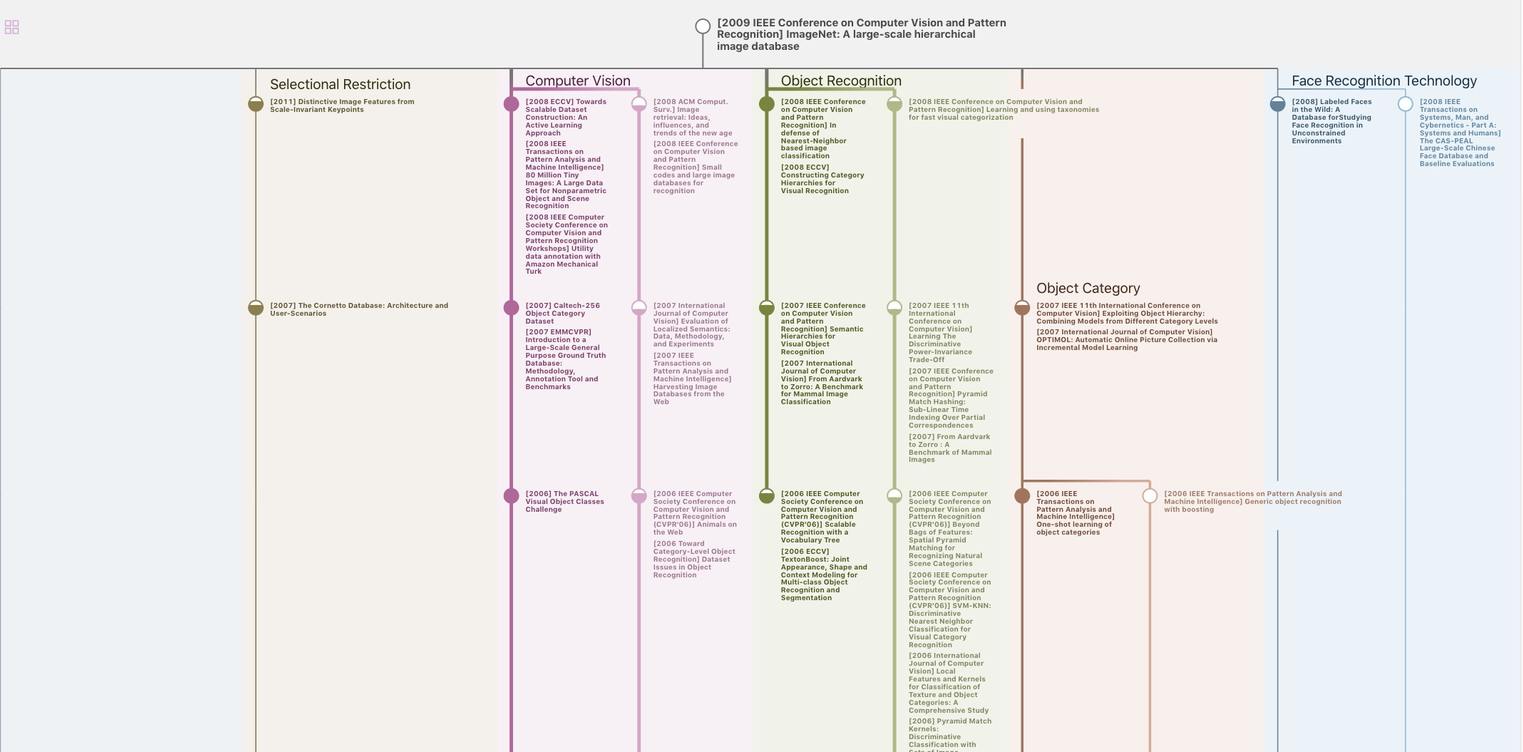
生成溯源树,研究论文发展脉络
Chat Paper
正在生成论文摘要