LRB-T: local reasoning back-projection transformer for the removal of bad weather effects in images
Neural Computing and Applications(2023)
Abstract
In computer vision, transformers have shown increasing effectiveness for high-level vision tasks. To further cope with low-level vision tasks, we propose a general framework, namely local reasoning back-projection transformer (LRB-T) for removing multiple types of bad weather (rain, haze, rain fog, raindrop, et al.) affecting images. Specifically, this paper first integrates the back-projection mechanism into the transformer architecture, where iterative up- and down-projection modules effectively feed and correct the feature reconstruction errors for spatial information preservation, and reduce computational costs since nearly half of the feature maps participate in the back-projection using half resolution. Besides, the proposed adaptive local reasoning block captures important neighborhood information through multiple local reasoning schemes. It can aggregate adjacent tokens to produce spatial-specific involution kernels, attention weights and dynamic positional encodings for local structure updating, and provide implicit spatial feature transform to achieve spatial-wise feature modulation. A pyramid scale guidance module is also established to enable arbitrary size generation consistent with the input, and generate scale-dependent trainable parameters to enhance skip connections. Extensive experiments on four types of well-known bad weather datasets show that the proposed LRB-T improves the image deraining, dehazing, de-rain fog and de-raindrop performance in terms of PSNR and SSIM effectively and outperforms state-of-the-art task-specific bad weather removal methods.
MoreTranslated text
Key words
Image restoration,Bad weather removal,Vision transformer,Back projection,Adaptive local reasoning
AI Read Science
Must-Reading Tree
Example
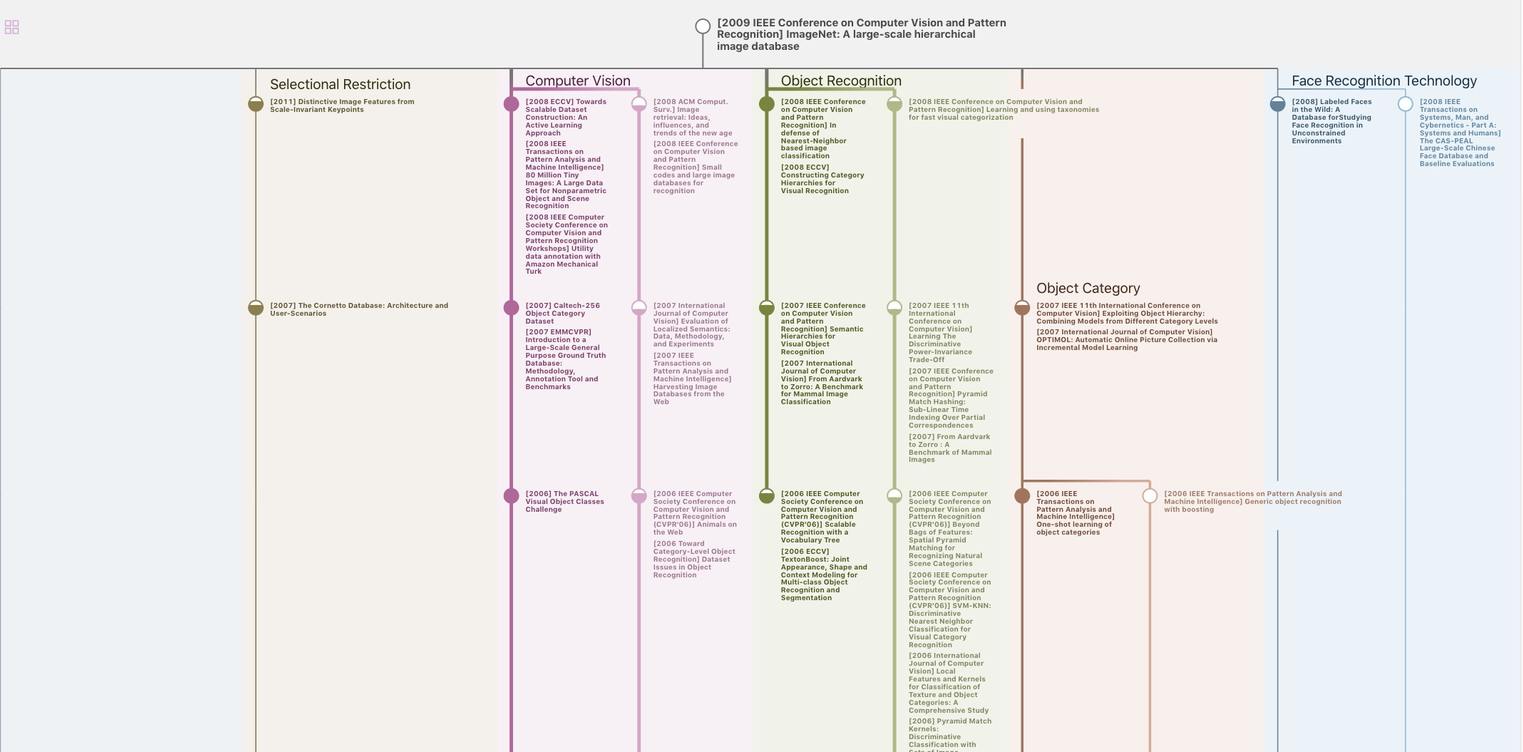
Generate MRT to find the research sequence of this paper
Chat Paper
Summary is being generated by the instructions you defined