Integrating predictive modeling techniques with geospatial data for landslide susceptibility assessment in northern Pakistan
Journal of Mountain Science(2023)
Abstract
The eastern road section of the China-Pakistan Economic Corridor (CPEC) traverses the challenging terrain of northern Pakistan, where frequent landslides pose a significant threat to socioeconomic development and infrastructure. However, the insufficient data on landslide hazards presents a substantial challenge to practical mitigation efforts. Therefore, we conducted an extensive study to gain insight into landslide assessment along the Mansehra-Muzaffarabad-Mirpur and Mangla (MMMM) Expressway. This study involved preparing a landslide inventory, analyzing landslide causative factors, and developing landslide susceptibility models (LSMs) using published data, remote sensing interpretations, field excursions and integrated predictive techniques. We first used Pearson’s correlation coefficient (PCC), variable importance factors (VIF), and information gain ratio (IGR) to evaluate multicollinearity among the selected landslide causative factors (LCFs). Then, the topographic roughness index (TRI) with VIF > 5 and PCC > 0.7 was considered a redundant factor and thus removed before the data modeling. Finally, we adopted multiple machine-learning methods to analyze landslide susceptibility. The results indicate that the landslide inventory contains 1,776 events, of which 674 were classified based on geometrical and lithological configurations. The IGR results show that the rainfall, lithology, PGA, drainage density, slope, and distance to fault are the most effective LCFs. The AUC values for random forest (RF) (0.901), extreme gradient boosting (XGBoost) (0.884), and K-nearest neighbor (KNN) (0.872) remained higher than evidential belief function (EBF) (0.833), weight of evidence (WoE) (0.820), and certainty factor (CF) (0.810), respectively. The RF model outperformed all other models in terms of prediction. However, these models are accurate but newer in the area; thus, susceptible zones were verified with comprehensive field investigations. The northern and central regions accounted for the high and very high susceptibility classes in the final landslide susceptibility mapping (LSM) compared to the southern areas.
MoreTranslated text
Key words
Landslides,MMMM expressway,Machine learning,Landslide susceptibility,Northern Pakistan
AI Read Science
Must-Reading Tree
Example
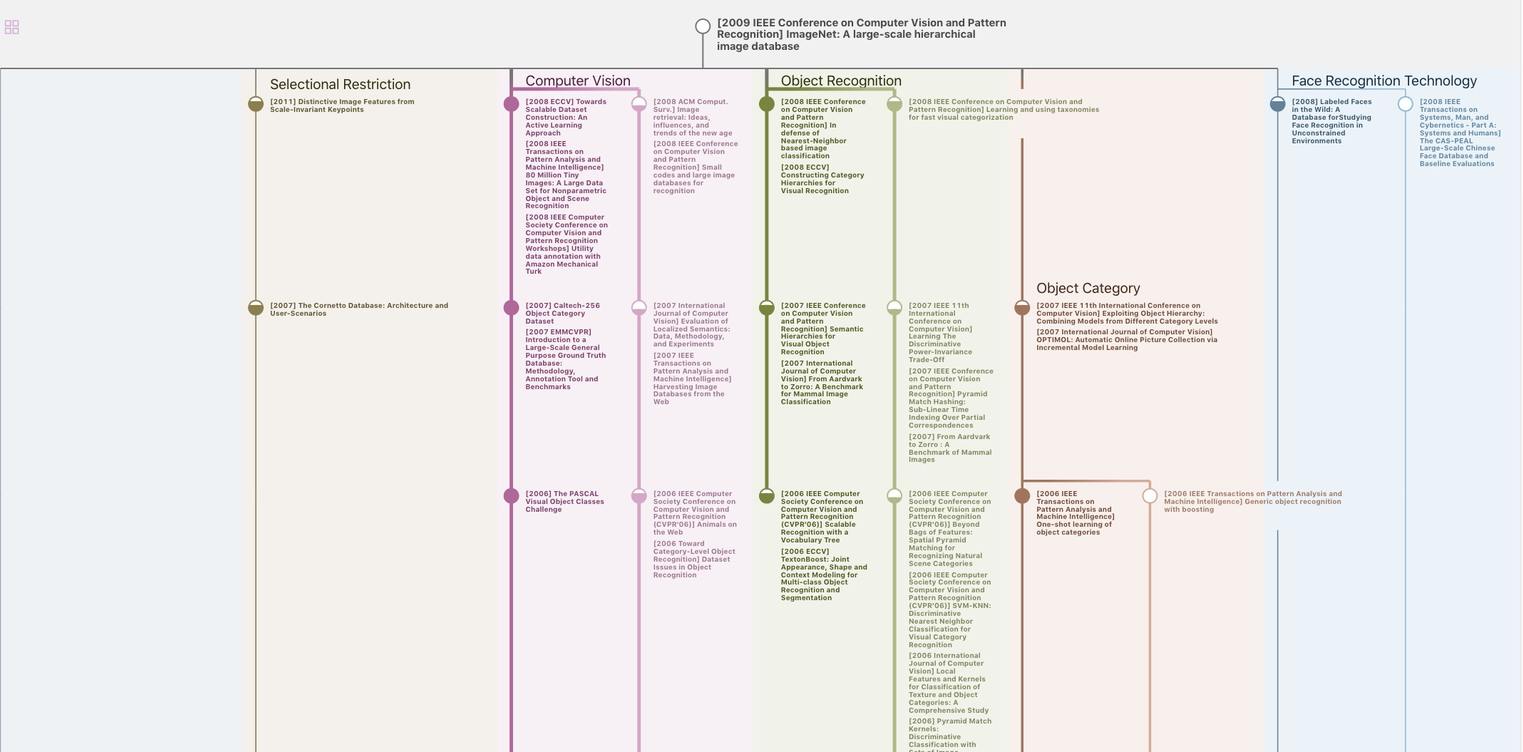
Generate MRT to find the research sequence of this paper
Chat Paper
Summary is being generated by the instructions you defined