Matching the Neuronal Representations of V1 is Necessary to Improve Robustness in CNNs with V1-like Front-ends
CoRR(2023)
Abstract
While some convolutional neural networks (CNNs) have achieved great success in object recognition, they struggle to identify objects in images corrupted with different types of common noise patterns. Recently, it was shown that simulating computations in early visual areas at the front of CNNs leads to improvements in robustness to image corruptions. Here, we further explore this result and show that the neuronal representations that emerge from precisely matching the distribution of RF properties found in primate V1 is key for this improvement in robustness. We built two variants of a model with a front-end modeling the primate primary visual cortex (V1): one sampling RF properties uniformly and the other sampling from empirical biological distributions. The model with the biological sampling has a considerably higher robustness to image corruptions that the uniform variant (relative difference of 8.72%). While similar neuronal sub-populations across the two variants have similar response properties and learn similar downstream weights, the impact on downstream processing is strikingly different. This result sheds light on the origin of the improvements in robustness observed in some biologically-inspired models, pointing to the need of precisely mimicking the neuronal representations found in the primate brain.
MoreTranslated text
Key words
neuronal representations,cnns,robustness,front-ends
AI Read Science
Must-Reading Tree
Example
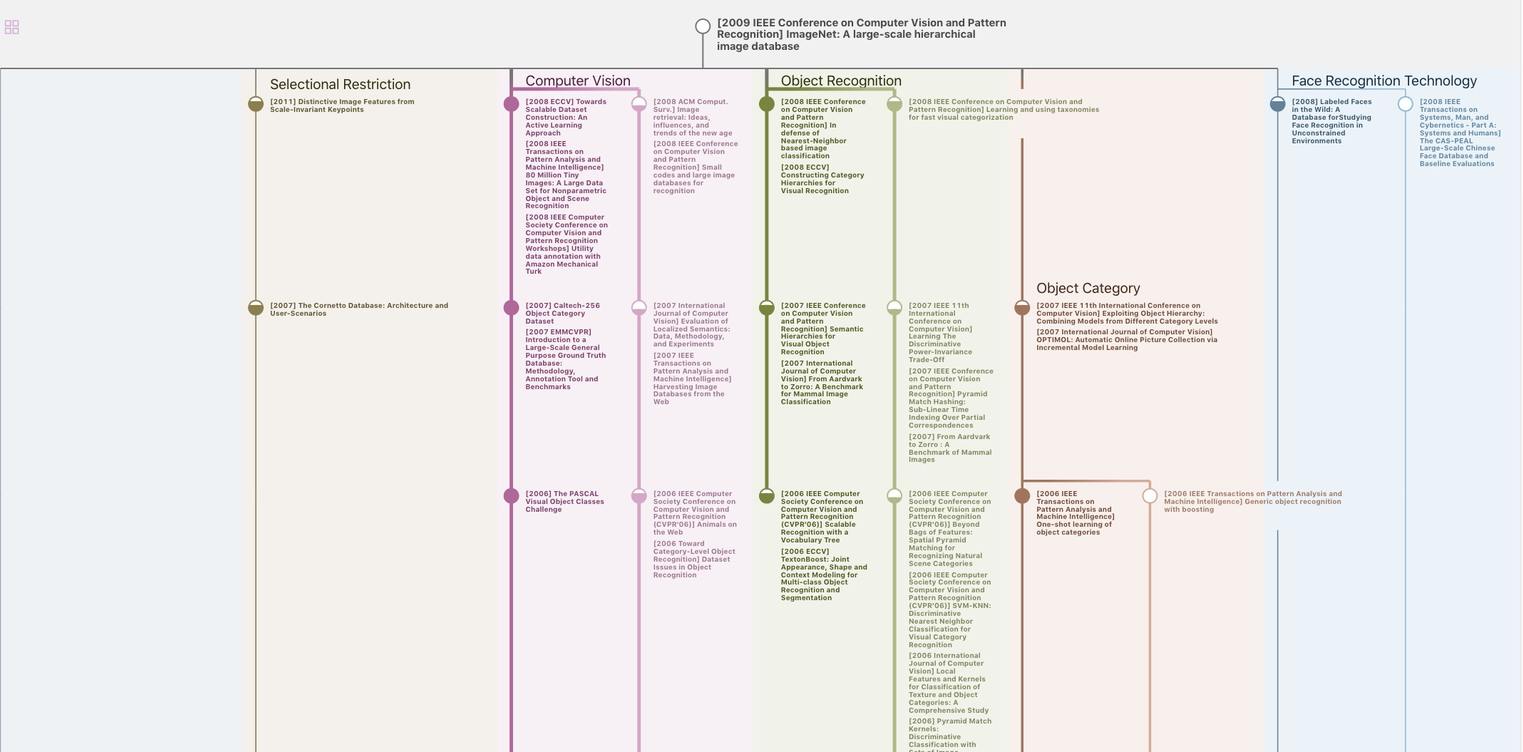
Generate MRT to find the research sequence of this paper
Chat Paper
Summary is being generated by the instructions you defined