InfoGCN++: Learning Representation by Predicting the Future for Online Human Skeleton-based Action Recognition
CoRR(2023)
摘要
Skeleton-based action recognition has made significant advancements recently, with models like InfoGCN showcasing remarkable accuracy. However, these models exhibit a key limitation: they necessitate complete action observation prior to classification, which constrains their applicability in real-time situations such as surveillance and robotic systems. To overcome this barrier, we introduce InfoGCN++, an innovative extension of InfoGCN, explicitly developed for online skeleton-based action recognition. InfoGCN++ augments the abilities of the original InfoGCN model by allowing real-time categorization of action types, independent of the observation sequence's length. It transcends conventional approaches by learning from current and anticipated future movements, thereby creating a more thorough representation of the entire sequence. Our approach to prediction is managed as an extrapolation issue, grounded on observed actions. To enable this, InfoGCN++ incorporates Neural Ordinary Differential Equations, a concept that lets it effectively model the continuous evolution of hidden states. Following rigorous evaluations on three skeleton-based action recognition benchmarks, InfoGCN++ demonstrates exceptional performance in online action recognition. It consistently equals or exceeds existing techniques, highlighting its significant potential to reshape the landscape of real-time action recognition applications. Consequently, this work represents a major leap forward from InfoGCN, pushing the limits of what's possible in online, skeleton-based action recognition. The code for InfoGCN++ is publicly available at https://github.com/stnoah1/infogcn2 for further exploration and validation.
更多查看译文
关键词
learning representation,action,recognition,skeleton-based
AI 理解论文
溯源树
样例
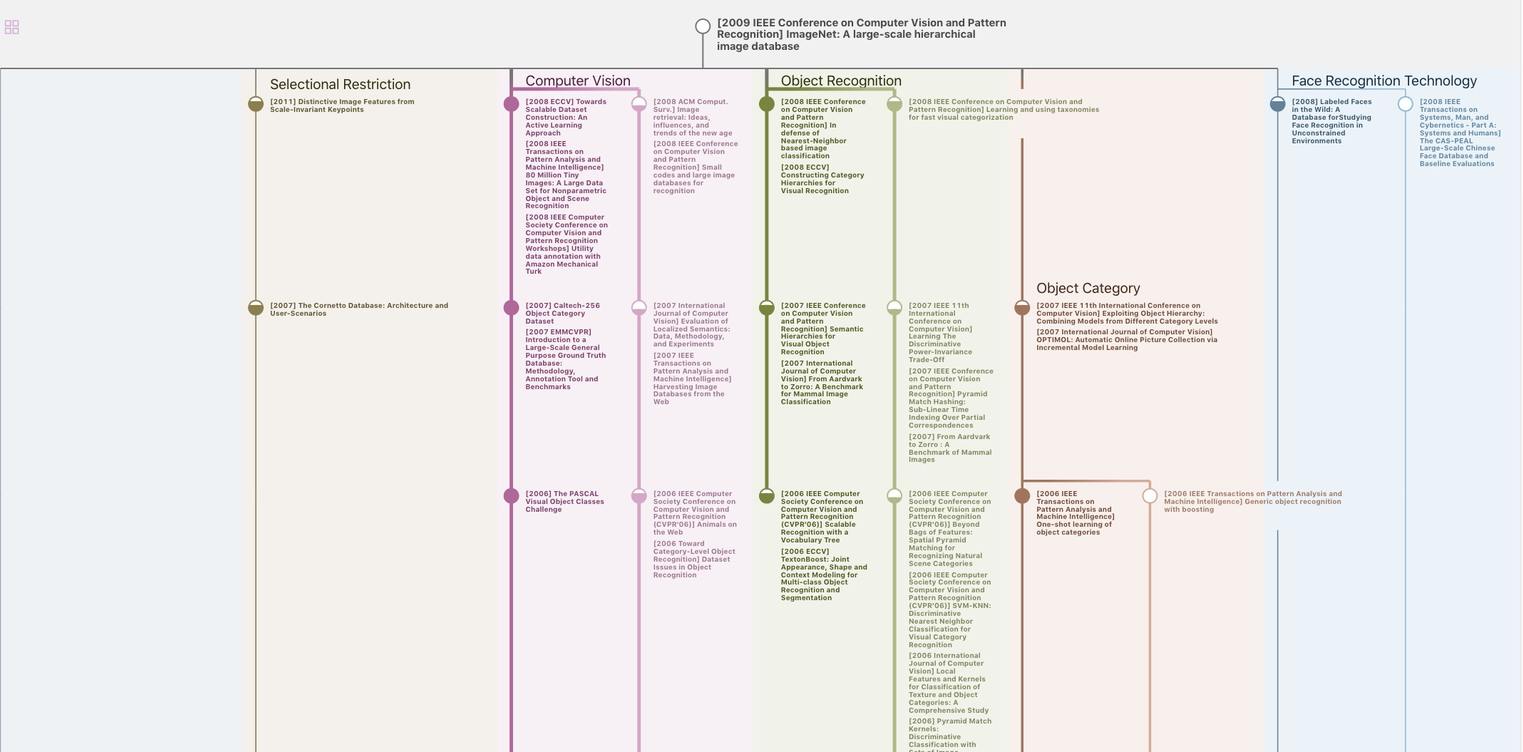
生成溯源树,研究论文发展脉络
Chat Paper
正在生成论文摘要