Block-missing data in linear systems: An unbiased stochastic gradient descent approach
CoRR(2023)
摘要
Achieving accurate approximations to solutions of large linear systems is crucial, especially when those systems utilize real-world data. A consequence of using real-world data is that there will inevitably be missingness. Current approaches for dealing with missing data, such as deletion and imputation, can introduce bias. Recent studies proposed an adaptation of stochastic gradient descent (SGD) in specific missing-data models. In this work, we propose a new algorithm, $\ell$-tuple mSGD, for the setting in which data is missing in a block-wise, tuple pattern. We prove that our proposed method uses unbiased estimates of the gradient of the least squares objective in the presence of tuple missing data. We also draw connections between $\ell$-tuple mSGD and previously established SGD-type methods for missing data. Furthermore, we prove our algorithm converges when using updating step sizes and empirically demonstrate the convergence of $\ell$-tuple mSGD on synthetic data. Lastly, we evaluate $\ell$-tuple mSGD applied to real-world continuous glucose monitoring (CGM) device data.
更多查看译文
关键词
unbiased stochastic gradient,linear systems,descent approach,data,block-missing
AI 理解论文
溯源树
样例
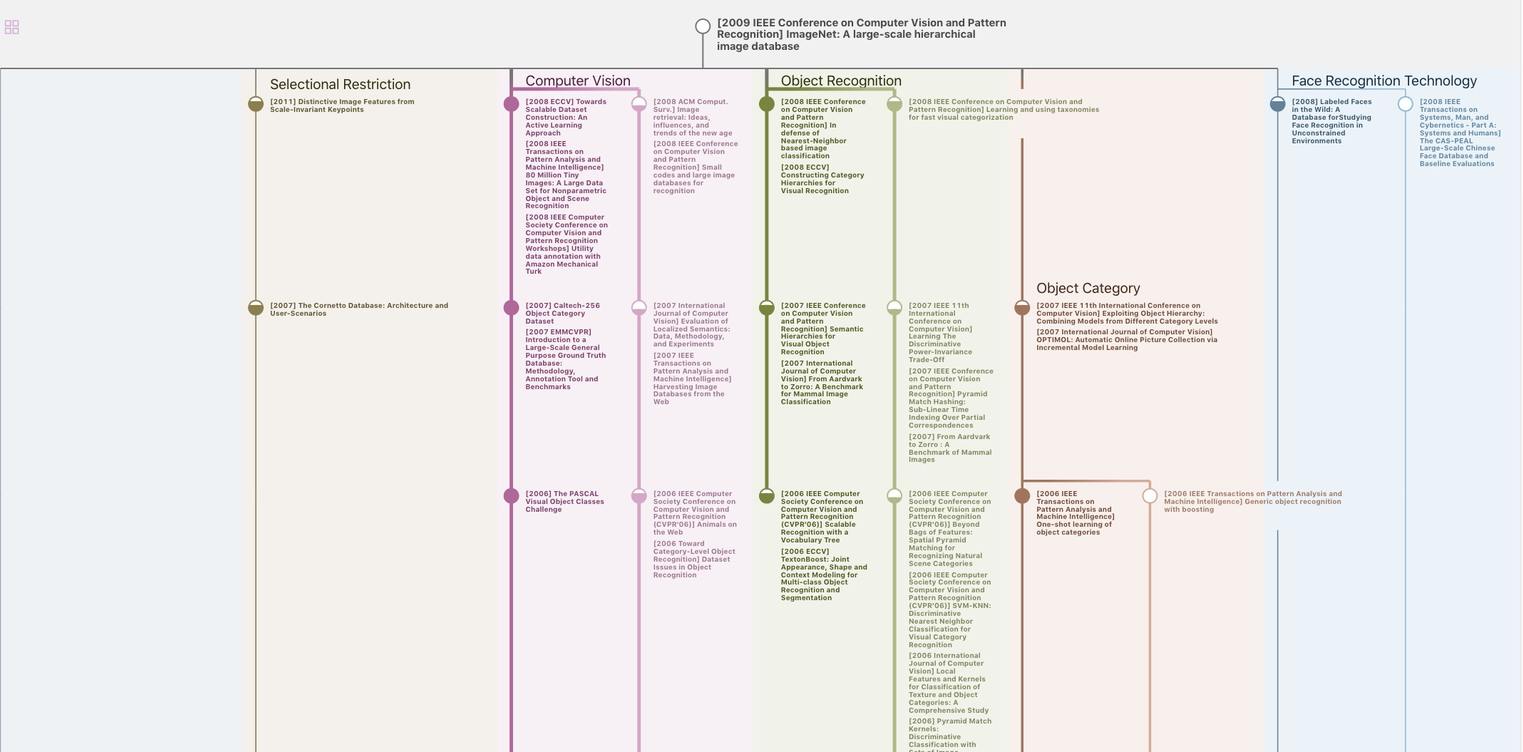
生成溯源树,研究论文发展脉络
Chat Paper
正在生成论文摘要