Outlier Detection Using Generative Models with Theoretical Performance Guarantees
CoRR(2023)
摘要
This paper considers the problem of recovering signals modeled by generative models from linear measurements contaminated with sparse outliers. We propose an outlier detection approach for reconstructing the ground-truth signals modeled by generative models under sparse outliers. We establish theoretical recovery guarantees for reconstruction of signals using generative models in the presence of outliers, giving lower bounds on the number of correctable outliers. Our results are applicable to both linear generator neural networks and the nonlinear generator neural networks with an arbitrary number of layers. We propose an iterative alternating direction method of multipliers (ADMM) algorithm for solving the outlier detection problem via $\ell_1$ norm minimization, and a gradient descent algorithm for solving the outlier detection problem via squared $\ell_1$ norm minimization. We conduct extensive experiments using variational auto-encoder and deep convolutional generative adversarial networks, and the experimental results show that the signals can be successfully reconstructed under outliers using our approach. Our approach outperforms the traditional Lasso and $\ell_2$ minimization approach.
更多查看译文
关键词
generative models,detection
AI 理解论文
溯源树
样例
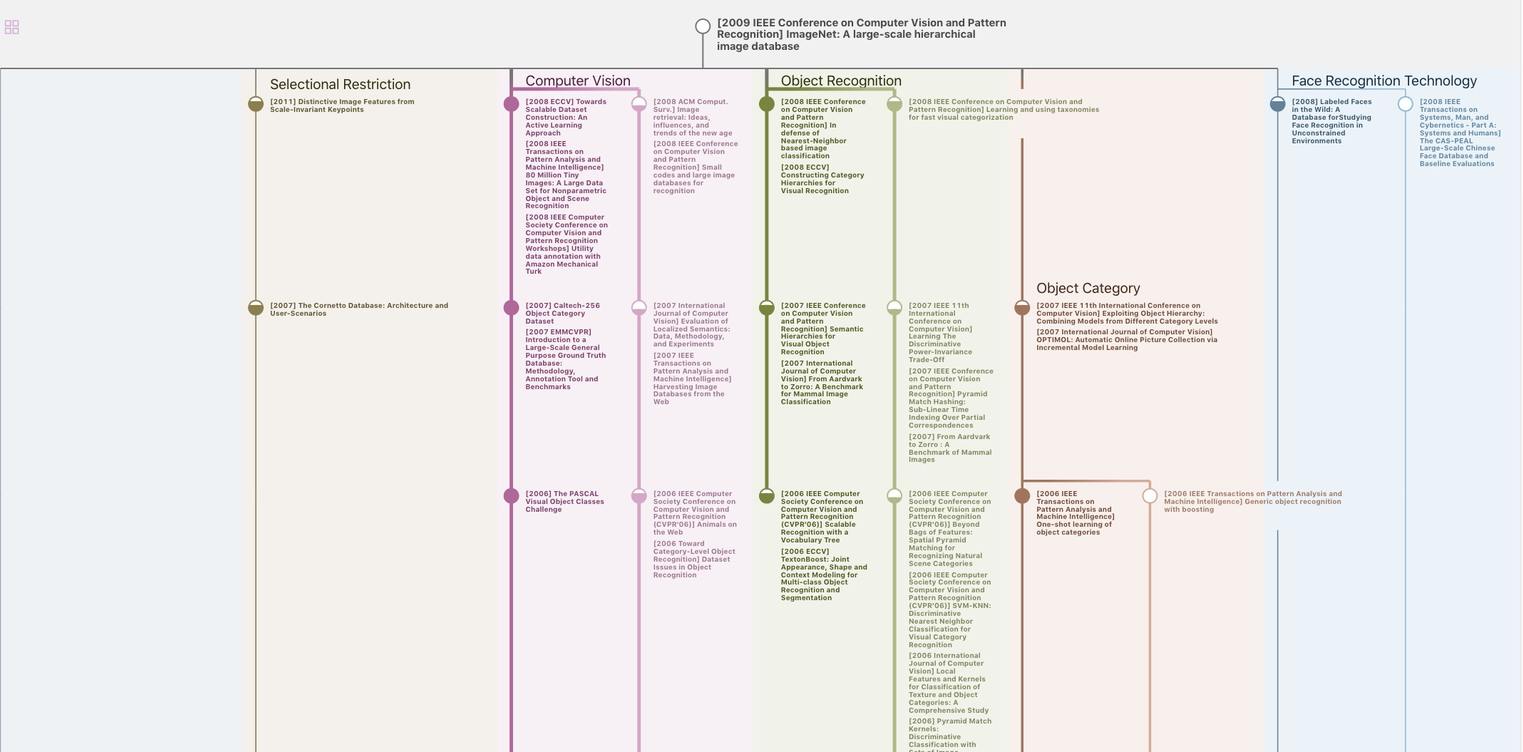
生成溯源树,研究论文发展脉络
Chat Paper
正在生成论文摘要