Tackling Heterogeneity in Medical Federated learning via Vision Transformers
CoRR(2023)
摘要
Optimization-based regularization methods have been effective in addressing the challenges posed by data heterogeneity in medical federated learning, particularly in improving the performance of underrepresented clients. However, these methods often lead to lower overall model accuracy and slower convergence rates. In this paper, we demonstrate that using Vision Transformers can substantially improve the performance of underrepresented clients without a significant trade-off in overall accuracy. This improvement is attributed to the Vision transformer's ability to capture long-range dependencies within the input data.
更多查看译文
关键词
medical federated learning,vision
AI 理解论文
溯源树
样例
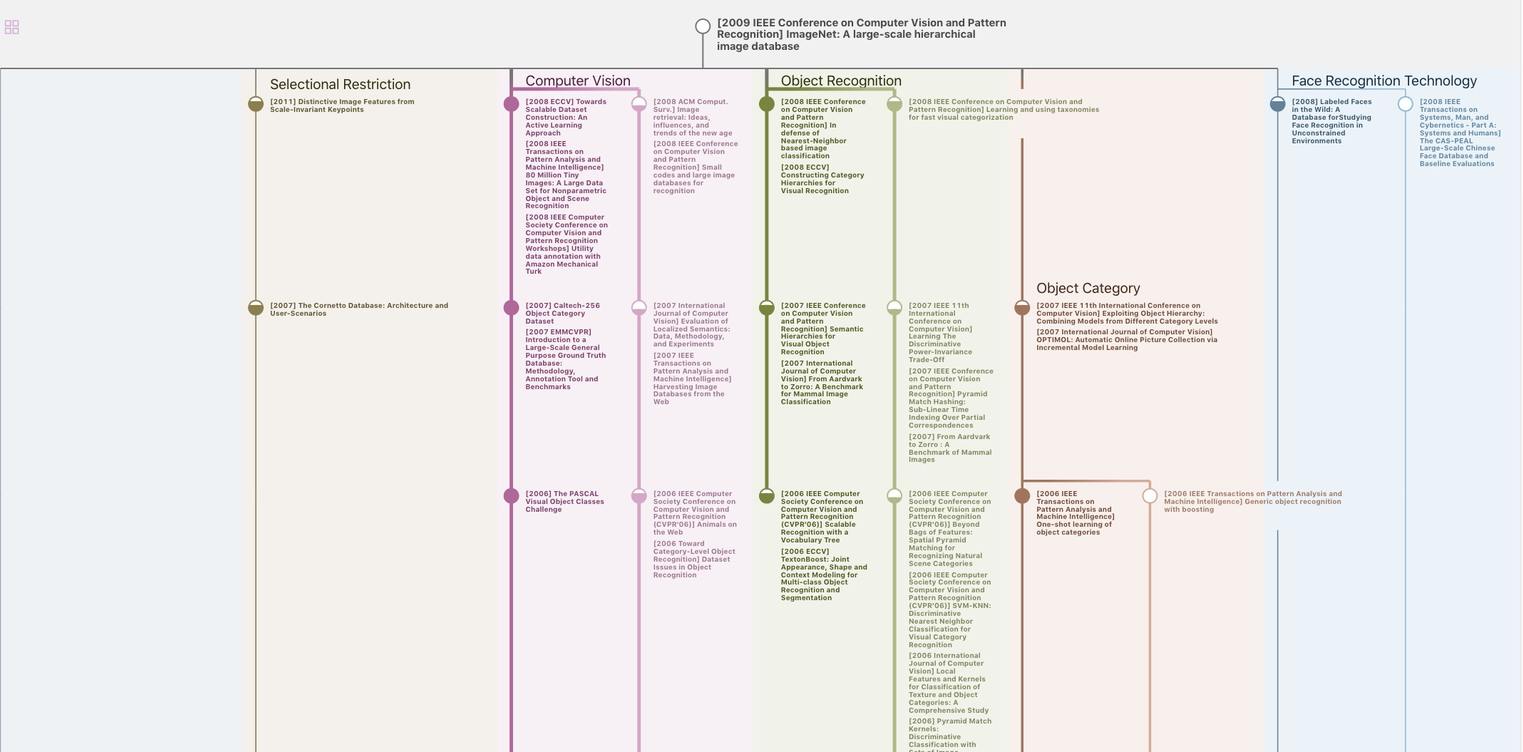
生成溯源树,研究论文发展脉络
Chat Paper
正在生成论文摘要