Enhancing Text Generation with Cooperative Training
ECAI 2023(2023)
摘要
Recently, there has been a surge in the use of generated data to enhance the performance of downstream models, largely due to the advancements in pre-trained language models. However, most prevailing methods trained generative and discriminative models in isolation, which left them unable to adapt to changes in each other. These approaches lead to generative models that are prone to deviating from the true data distribution and providing limited benefits to discriminative models. While some works have proposed jointly training generative and discriminative language models, their methods remain challenging due to the non-differentiable nature of discrete data. To overcome these issues, we introduce a self-consistent learning framework in the text field that involves training a discriminator and generator cooperatively in a closed-loop manner until a scoring consensus is reached. By learning directly from selected samples, our framework are able to mitigate training instabilities such as mode collapse and non-convergence. Extensive experiments on four downstream benchmarks, including AFQMC, CHIP-STS, QQP, and MRPC, demonstrate the efficacy of the proposed framework.
更多查看译文
关键词
text generation,cooperative training
AI 理解论文
溯源树
样例
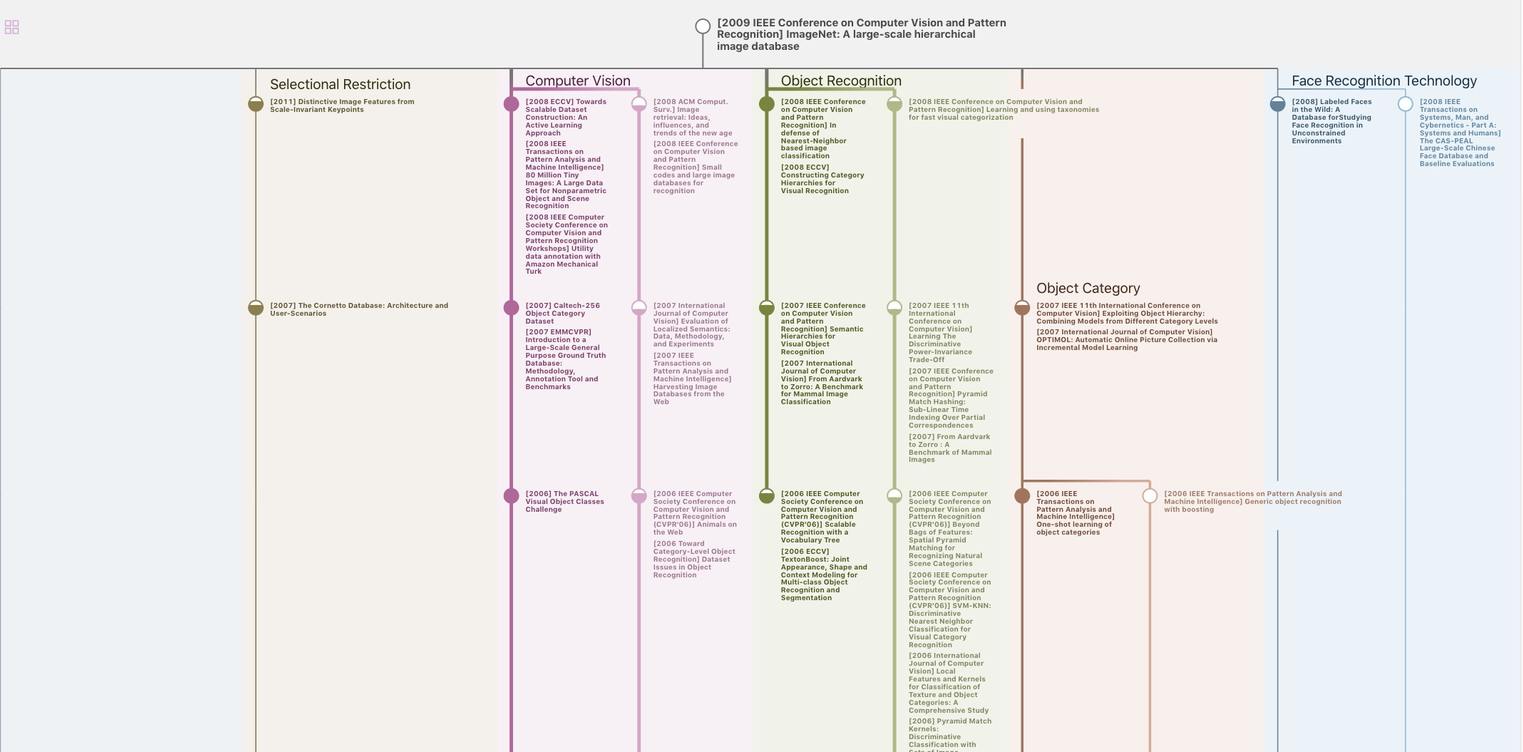
生成溯源树,研究论文发展脉络
Chat Paper
正在生成论文摘要