Degradation-Resistant Offline Optimization via Accumulative Risk Control
ECAI 2023(2023)
摘要
Offline optimization aims to elaborately construct a solution that optimizes a black-box function with only access to the offline dataset. A typical manner of constructing the solution is to train a surrogate model of the black-box function on the offline dataset and optimize the solution guided by the surrogate model. However, this manner often encounters a fundamental challenge that the surrogate model could erroneously estimate out-of-distribution (OOD) solutions. Therefore, the optimizer would be misled to produce inferior solutions for online applications, i.e., degradation of performance. To this end, this paper formalizes the risk of degradation for OOD solutions and proposes an accumulative risk controlled offline optimization (ARCOO) method. Specifically, ARCOO learns a surrogate model in conjunction with an energy model. The energy model characterizes the risk of degradation by learning on high-risk solutions and low-risk ones contrastively. In the optimization procedure, the behavior of the optimizer in each step is controlled by a risk suppression factor calculated via the energy model, which leads to the controllable accumulative risk. Theoretically, we justify the efficacy of energy for accumulative risk control. Extensive experiments on offline optimization tasks show that ARCOO surpasses state-of-the-art methods in both degradation-resistance and optimality of the output solution.
更多查看译文
关键词
optimization,degradation-resistant
AI 理解论文
溯源树
样例
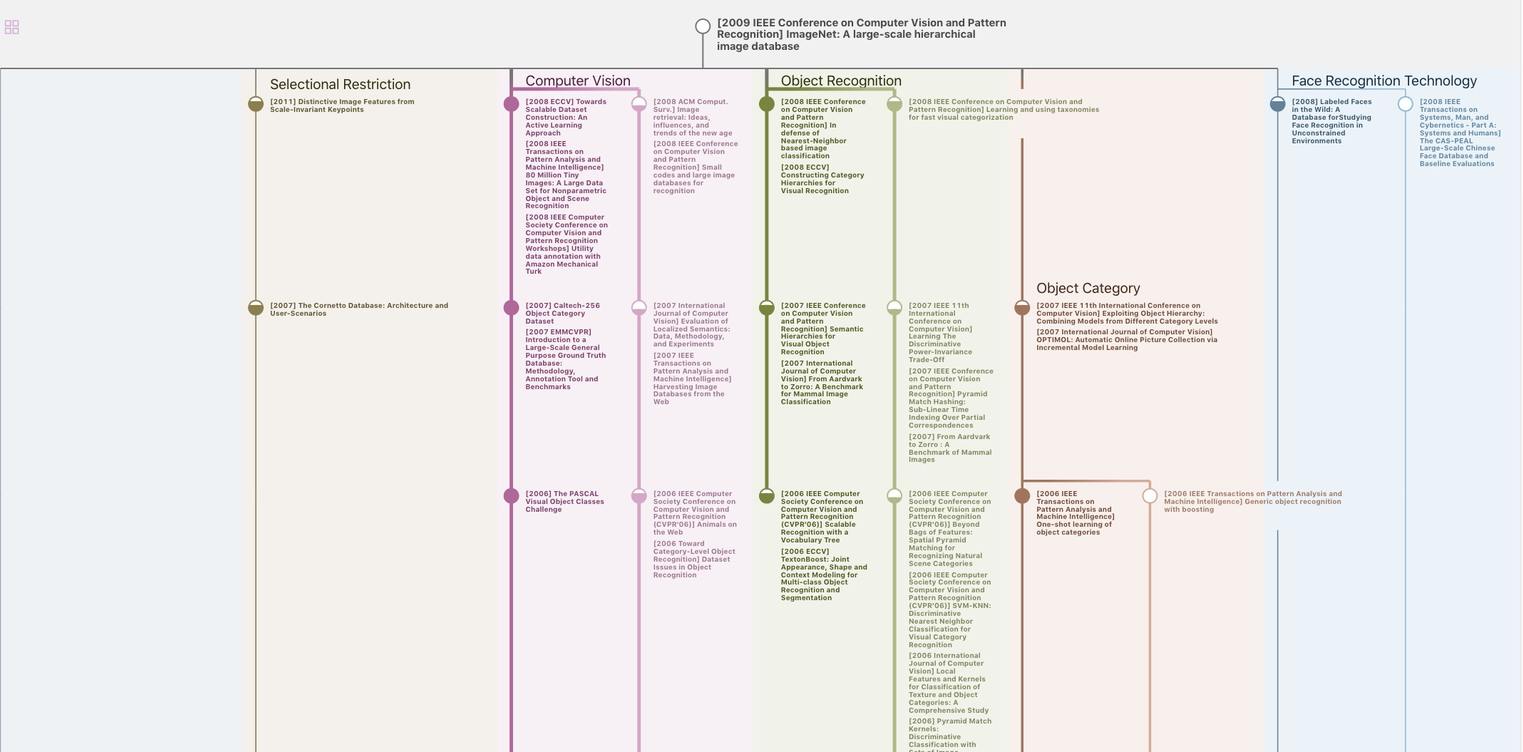
生成溯源树,研究论文发展脉络
Chat Paper
正在生成论文摘要