Multi-parametric optimization of magnetic resonance imaging sequences for magnetic resonance-guided radiotherapy
PHYSICS & IMAGING IN RADIATION ONCOLOGY(2023)
Abstract
Background and purpose: Magnetic Resonance Imaging (MRI) is widely used in oncology for tumor staging, treatment response assessment, and radiation therapy (RT) planning. This study proposes a framework for automatic optimization of MRI sequences based on pulse sequence parameter sets (SPS) that are directly applied on the scanner, for application in RT planning.Materials and methods: A phantom with seven in-house fabricated contrasts was used for measurements. The proposed framework employed a derivative-free optimization algorithm to repeatedly update and execute a parametrized sequence on the MR scanner to acquire new data. In each iteration, the mean-square error was calculated based on the clinical application. Two clinically relevant optimization goals were pursued: achieving the same signal and therefore contrast as in a target image, and maximizing the signal difference (contrast) between specified tissue types. The framework was evaluated using two optimization methods: a covariance matrix adaptation evolution strategy (CMA-ES) and a genetic algorithm (GA). Results: The obtained results demonstrated the potential of the proposed framework for automatic optimization of MRI sequences. Both CMA-ES and GA methods showed promising results in achieving the two optimization goals, however, CMA-ES converged much faster as compared to GA.Conclusions: The proposed framework enables for automatic optimization of MRI sequences based on SPS that are directly applied on the scanner and it may be used to enhance the quality of MRI images for dedicated applications in MR-guided RT.
MoreTranslated text
Key words
Contrast maximization,MR-guided radiotherapy,Remote control MRI,Supervised learning,MR sequences optimization
AI Read Science
Must-Reading Tree
Example
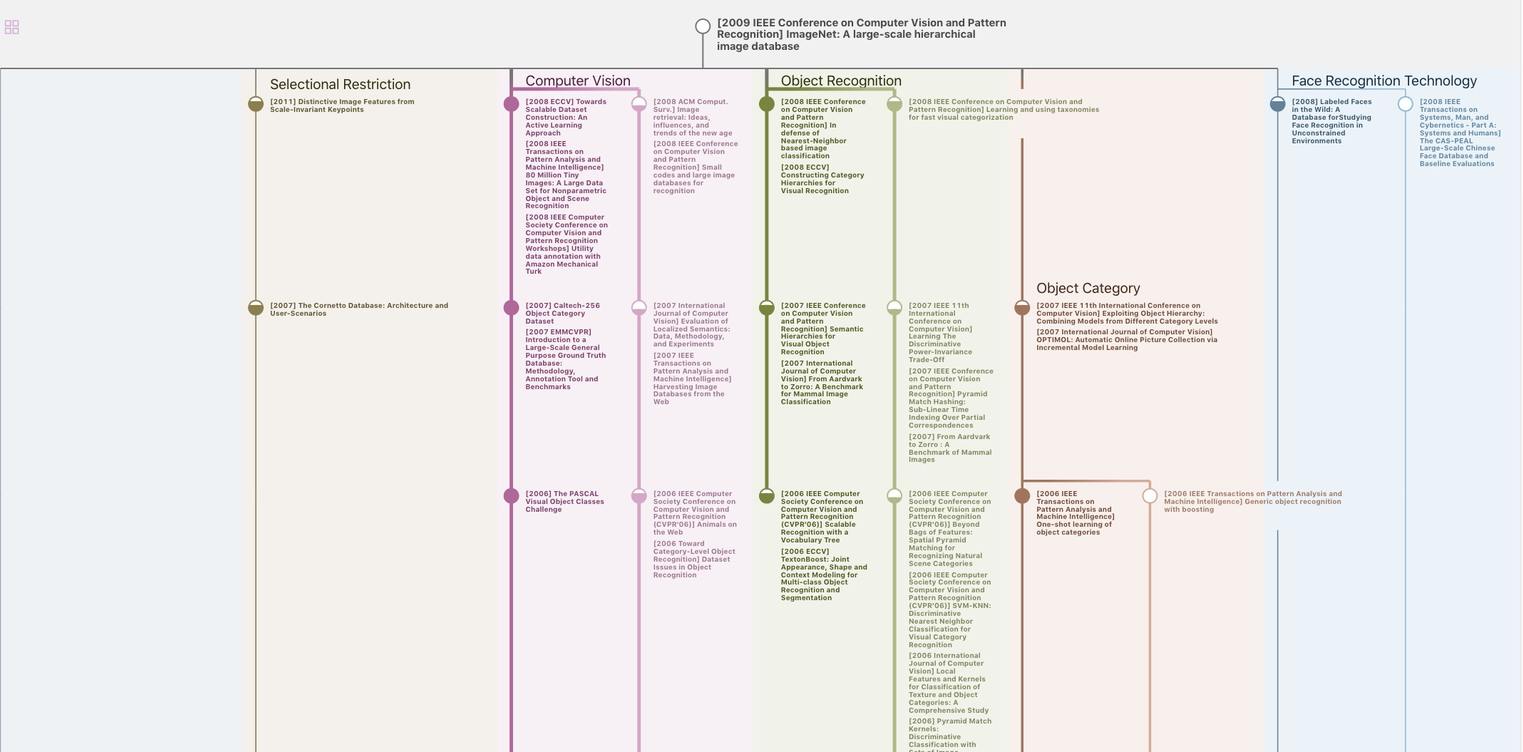
Generate MRT to find the research sequence of this paper
Chat Paper
Summary is being generated by the instructions you defined