Software techniques for training restricted Boltzmann machines on size-constrained quantum annealing hardware
Frontiers in Computer Science(2023)
Abstract
Restricted Boltzmann machines are common machine learning models that can utilize quantum annealing devices in their training processes as quantum samplers. While this approach has shown promise as an alternative to classical sampling methods, the limitations of quantum annealing hardware, such as the number of qubits and the lack of connectivity between the qubits, still pose a barrier to wide-scale adoption. We propose the use of multiple software techniques such as dropout method, passive labeling, and parallelization techniques for addressing these hardware limitations. The study found that using these techniques along with quantum sampling showed comparable results to its classical counterparts in certain contexts, while in others the increased complexity of the sampling process hindered the performance of the trained models. This means that further research into the behavior of quantum sampling needs to be done to apply quantum annealing to training tasks of more complicated RBM models.
MoreTranslated text
Key words
boltzmann machines,quantum,size-constrained
AI Read Science
Must-Reading Tree
Example
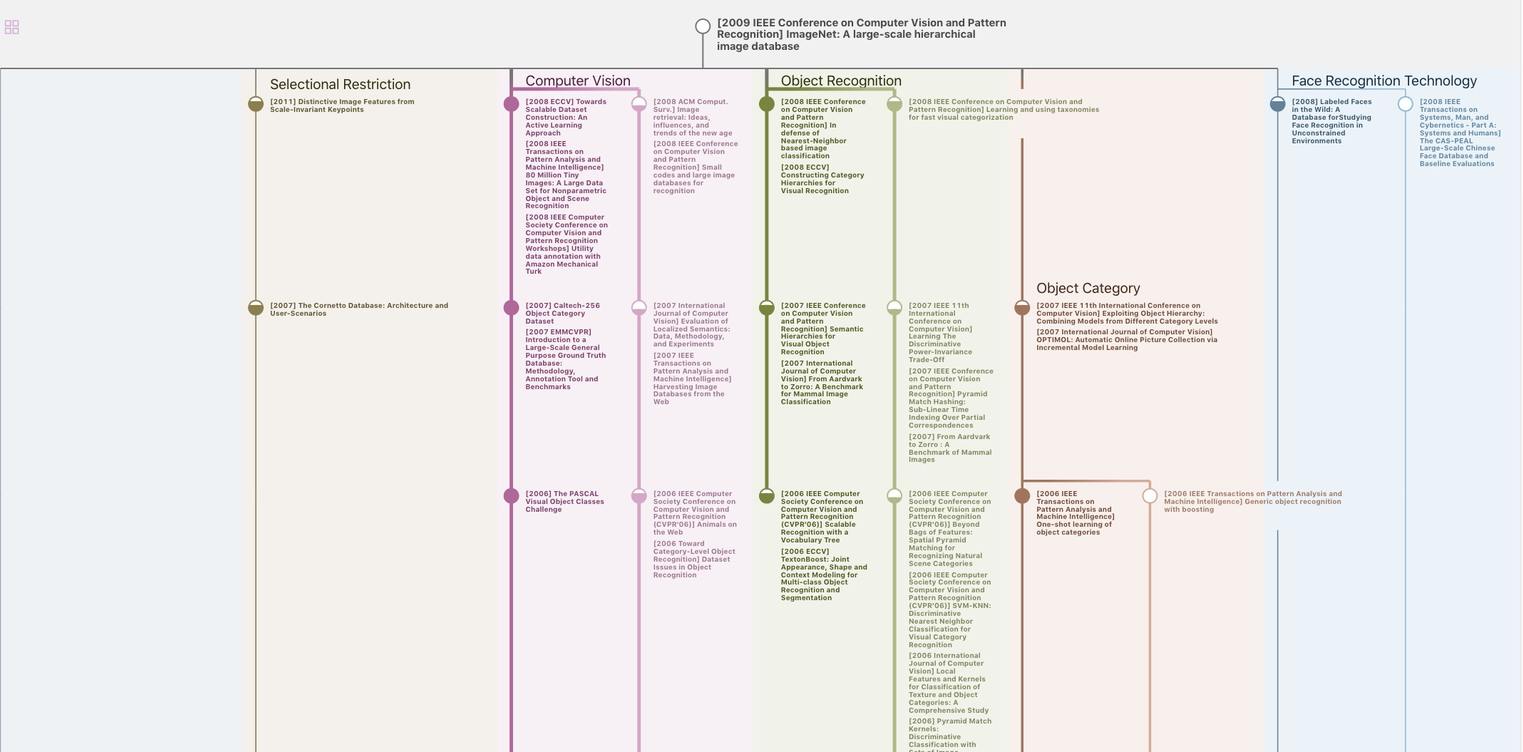
Generate MRT to find the research sequence of this paper
Chat Paper
Summary is being generated by the instructions you defined