Bottom-Up Short-Term Load Forecasting Considering Macro-Region and Weighting by Meteorological Region
Energies(2023)
摘要
Activities related to the planning and operation of power systems use premise load forecasting, which is responsible for providing a load estimative for a given horizon that assists mainly in the operation of an electrical system. Hierarchical short-term load forecasting (STLF) becomes an approach used for this purpose, where the overall forecast is performed through system partition in smaller macro-regions and, soon after, is aggregated to compose a global forecast. In this context, this paper presents a bottom-up STLF approach for macro-regions. The main innovation is the Average Consumption per Meteorological Region (CERM) index, used to weigh the importance of each station meteorological (EM) in total load demand. Another index, the Variation of Load and Temperature (IVCT), based on historical temperature and demand changes, is proposed. These indexes are incorporated into an ANN model of the multi-layer perceptron type (MLP). The results showed a higher average performance of the index CERM and variable IVCT in relation to the other combinations performed, and the best results were used to compose the prediction of the MTR. Finally, the proposed model presented a Mean Absolute Percentage Error lower than 1%, presenting superior performance compared to an aggregate model for MTR, which shows the efficiency and contribution of the proposed methodology.
更多查看译文
关键词
artificial neural network,hierarchical short-term load forecasting,multi-region forecasting,meteorological variables weighting
AI 理解论文
溯源树
样例
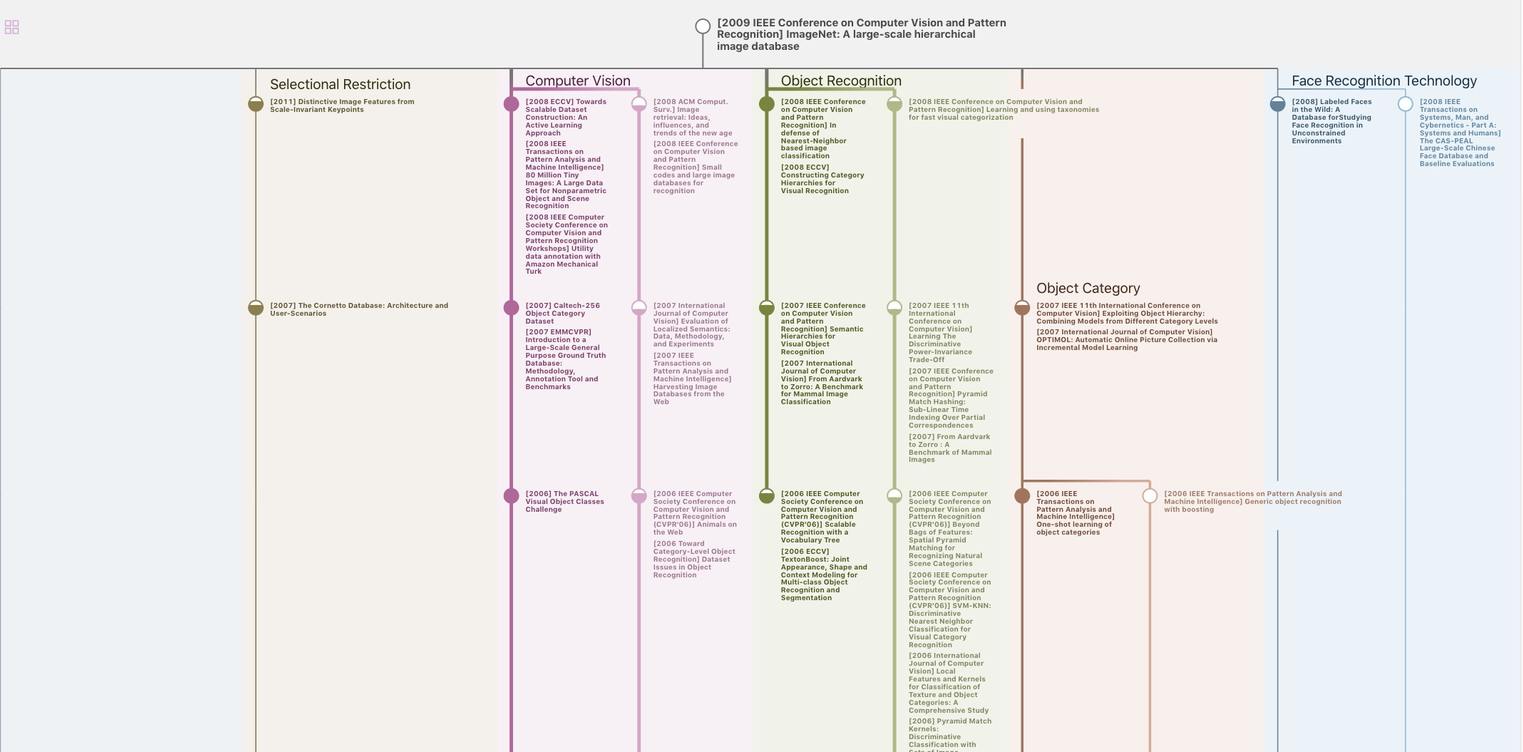
生成溯源树,研究论文发展脉络
Chat Paper
正在生成论文摘要