Global–Local Information Fusion Network for Road Extraction: Bridging the Gap in Accurate Road Segmentation in China
Remote Sensing(2023)
摘要
Road extraction is crucial in urban planning, rescue operations, and military applications. Compared to traditional methods, using deep learning for road extraction from remote sensing images has demonstrated unique advantages. However, previous convolutional neural networks (CNN)-based road extraction methods have had limited receptivity and failed to effectively capture long-distance road features. On the other hand, transformer-based methods have good global information-capturing capabilities, but face challenges in extracting road edge information. Additionally, existing excellent road extraction methods lack validation for the Chinese region. To address these issues, this paper proposes a novel road extraction model called the global–local information fusion network (GLNet). In this model, the global information extraction (GIE) module effectively integrates global contextual relationships, the local information extraction (LIE) module accurately captures road edge information, and the information fusion (IF) module combines the output features from both global and local branches to generate the final extraction results. Further, a series of experiments on two different Chinese road datasets with geographic robustness demonstrate that our model outperforms the state-of-the-art deep learning models for road extraction tasks in China. On the CHN6-CUG dataset, the overall accuracy (OA) and intersection over union (IoU) reach 97.49% and 63.27%, respectively, while on the RDCME dataset, OA and IoU reach 98.73% and 84.97%, respectively. These research results hold significant implications for road traffic, humanitarian rescue, and environmental monitoring, particularly in the context of the Chinese region.
更多查看译文
关键词
road extraction,high-resolution remote sensing images,global–local information fusion network,deep learning
AI 理解论文
溯源树
样例
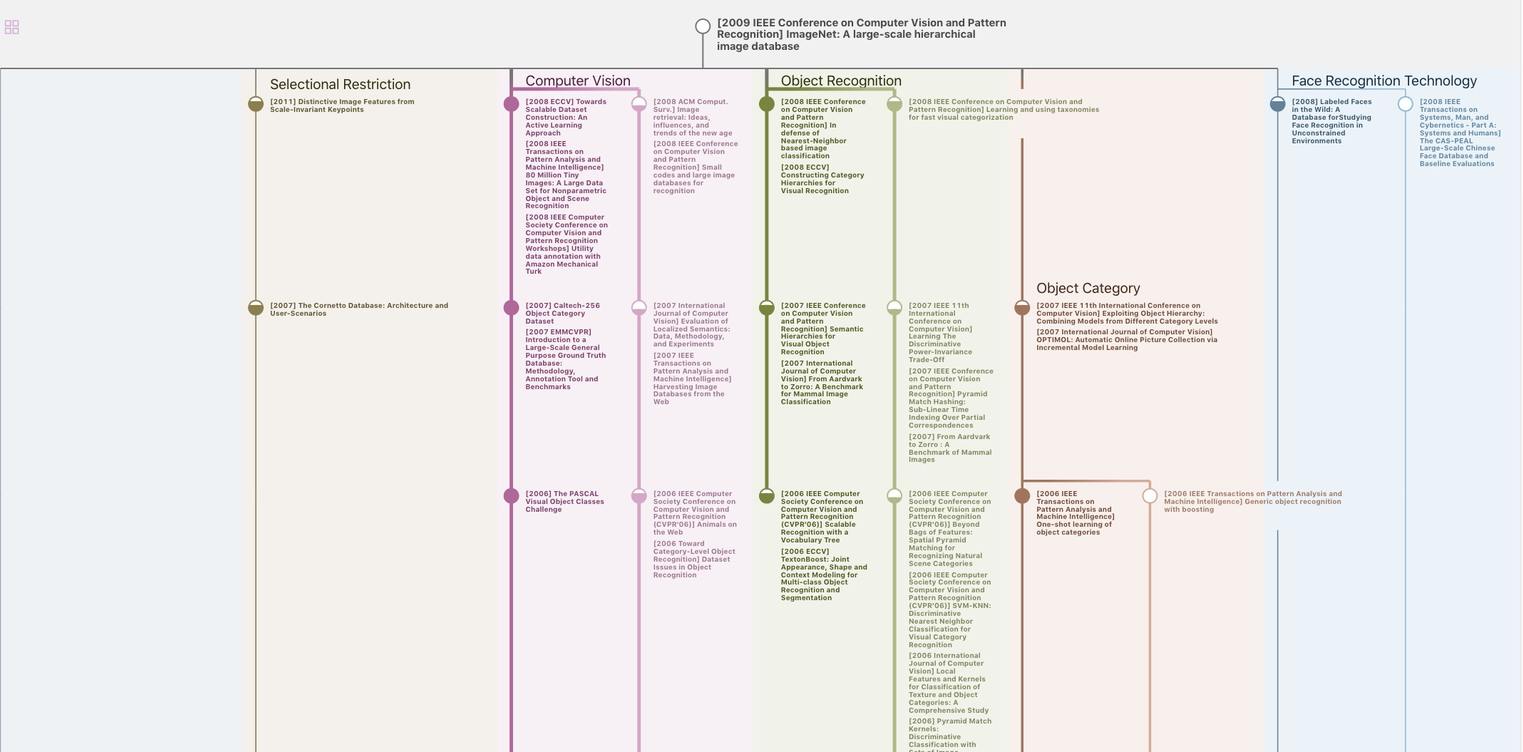
生成溯源树,研究论文发展脉络
Chat Paper
正在生成论文摘要