Sequential backward feature selection for optimizing permanent strain model of unbound aggregates
Case Studies in Construction Materials(2023)
摘要
This study proposes a novel framework for identifying the optimal feature set required to predict the permanent strain of unbound aggregates. An experimental database consisting of 16 input features is preprocessed and the performance of 10 machine learning models is evaluated. The best-performing model is then paired with a sequential backward selection algorithm to determine the optimal feature set for predicting the permanent strain. Finally, the selected features are used to predict the permanent strain, and the performance is compared with those obtained from the principal components analysis. Six features are selected as the optimal feature set. Furthermore, the selected features accurately predict permanent strain with a root mean square error value of 0.014, which is smaller than those obtained from principal components analysis. Thus, the feature selection approach for machine learning models effectively predicts the permanent strain of unbound aggregates using a limited set of input features.
更多查看译文
关键词
sequential backward feature selection,permanent strain model,aggregates
AI 理解论文
溯源树
样例
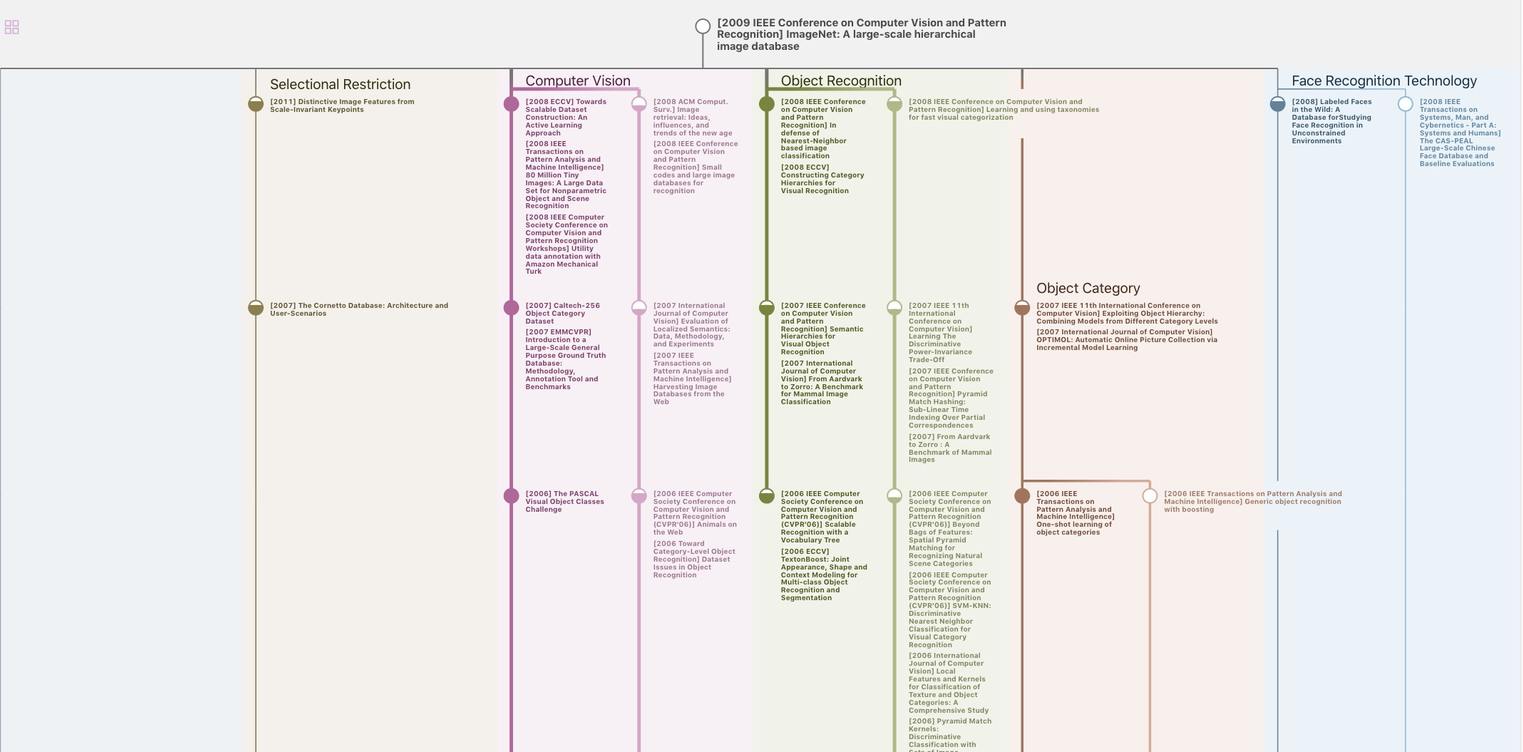
生成溯源树,研究论文发展脉络
Chat Paper
正在生成论文摘要