Qilin-Med: Multi-stage Knowledge Injection Advanced Medical Large Language Model
arxiv(2023)
摘要
Integrating large language models (LLMs) into healthcare holds great
potential but faces challenges. Pre-training LLMs from scratch for domains like
medicine is resource-heavy and often unfeasible. On the other hand, sole
reliance on Supervised Fine-tuning (SFT) can result in overconfident
predictions and may not tap into domain-specific insights. In response, we
present a multi-stage training method combining Domain-specific Continued
Pre-training (DCPT), SFT, and Direct Preference Optimization (DPO). In
addition, we publish a 3Gb Chinese Medicine (ChiMed) dataset, encompassing
medical question answering, plain texts, knowledge graphs, and dialogues,
segmented into three training stages. The medical LLM trained with our
pipeline, Qilin-Med, shows substantial performance improvement. In the CPT and
SFT phases, Qilin-Med achieved 38.4
respectively. It outperformed the basemodel Baichuan-7B (accuracy: 33.5
7.5
Huatuo-26M test set, bringing further improvement to the SFT phase (12.69 in
BLEU-1 and 24.21 in ROUGE-1). Additionally, we have further enhanced the
model's performance through the Retrieval Augmented Generation (RAG) approach.
Experiments demonstrate that Qilin-Med-RAG achieves an accuracy rate of 42.8
on CMExam. These results highlight the contribution of our novel training
approach in building LLMs for medical applications.
更多查看译文
AI 理解论文
溯源树
样例
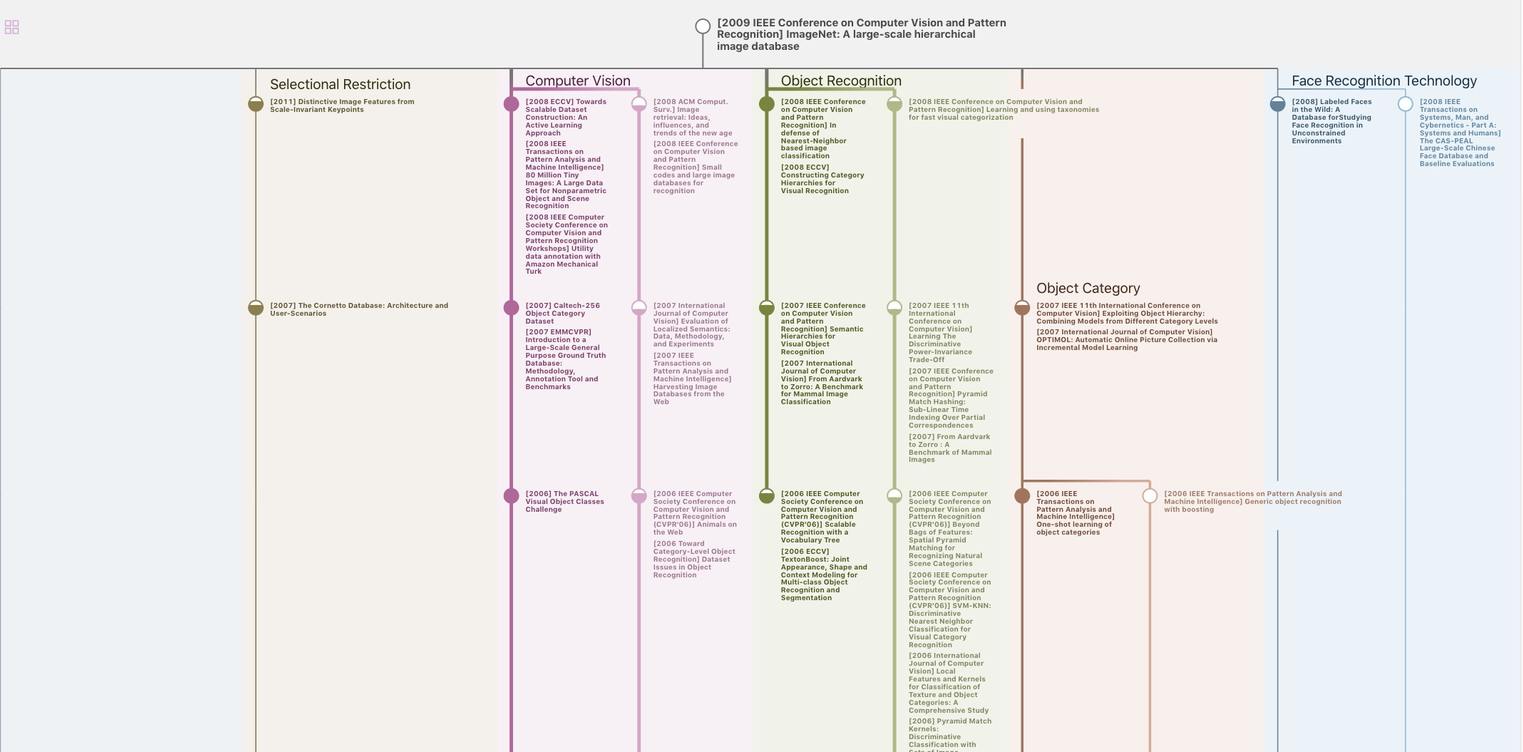
生成溯源树,研究论文发展脉络
Chat Paper
正在生成论文摘要