Designing Observables for Measurements with Deep Learning
CoRR(2023)
Abstract
Many analyses in particle and nuclear physics use simulations to infer fundamental, effective, or phenomenological parameters of the underlying physics models. When the inference is performed with unfolded cross sections, the observables are designed using physics intuition and heuristics. We propose to design optimal observables with machine learning. Unfolded, differential cross sections in a neural network output contain the most information about parameters of interest and can be well-measured by construction. We demonstrate this idea using two physics models for inclusive measurements in deep inelastic scattering.
MoreTranslated text
Key words
deep learning,measurements
AI Read Science
Must-Reading Tree
Example
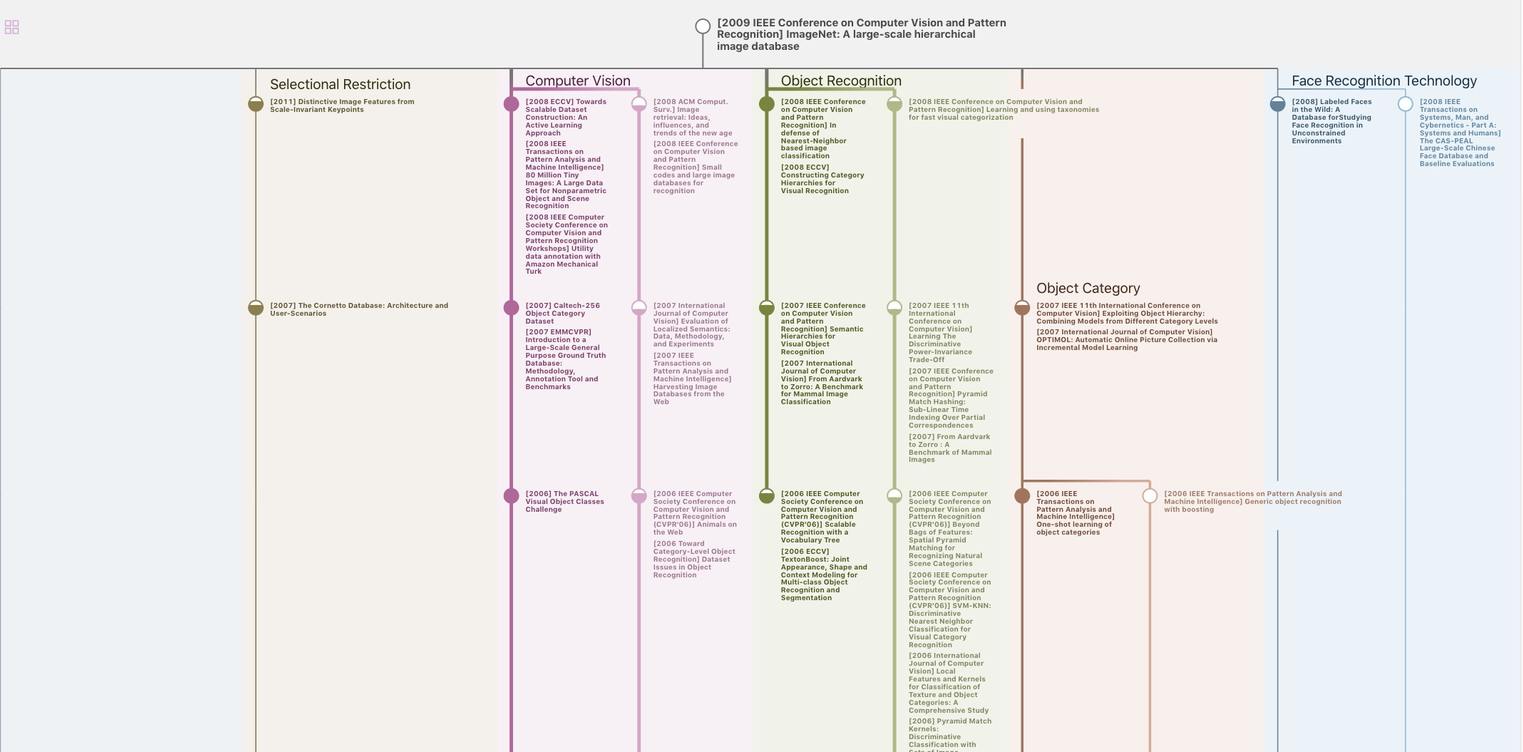
Generate MRT to find the research sequence of this paper
Chat Paper
Summary is being generated by the instructions you defined