Improved RAkEL's Fault Diagnosis Method for High-Speed Train Traction Transformer.
Sensors (Basel, Switzerland)(2023)
摘要
The traction system is very important to ensure the safe operation of high-speed trains, and the failure of the traction transformer is the most likely fault in the traction system. Fault diagnosis in actual work relies largely on manual experience. This paper proposes an improved RAkEL (Random -Labelsets) algorithm for the fault diagnosis of high-speed train traction transformers. Firstly, this article starts from the large amount of "sleeping" fault maintenance data accumulated by the railway department, takes a single maintenance record as an instance, uses specific monitoring values to construct an instance vector, and uses the fault phenomena corresponding to the monitoring indicators as labels. Then, this paper improves the step of selecting -labelsets in RAkEL, and extracts associated faults using the Relief algorithm. Finally, this paper excavates and uses the association rules between data and faults to identify traction transformer faults. The results showed that the improved RAkEL diagnostic method had a significant improvement in the evaluation indicators. Compared with other multi-label classification algorithms, including BR (Binary Relevance) and CLR (Calibrated Label Ranking), this method performs well on multiple evaluation indicators. It can further help engineers perform timely maintenance work in the future.
更多查看译文
关键词
multi-label classification, RAkEL, traction transformer, fault diagnosis
AI 理解论文
溯源树
样例
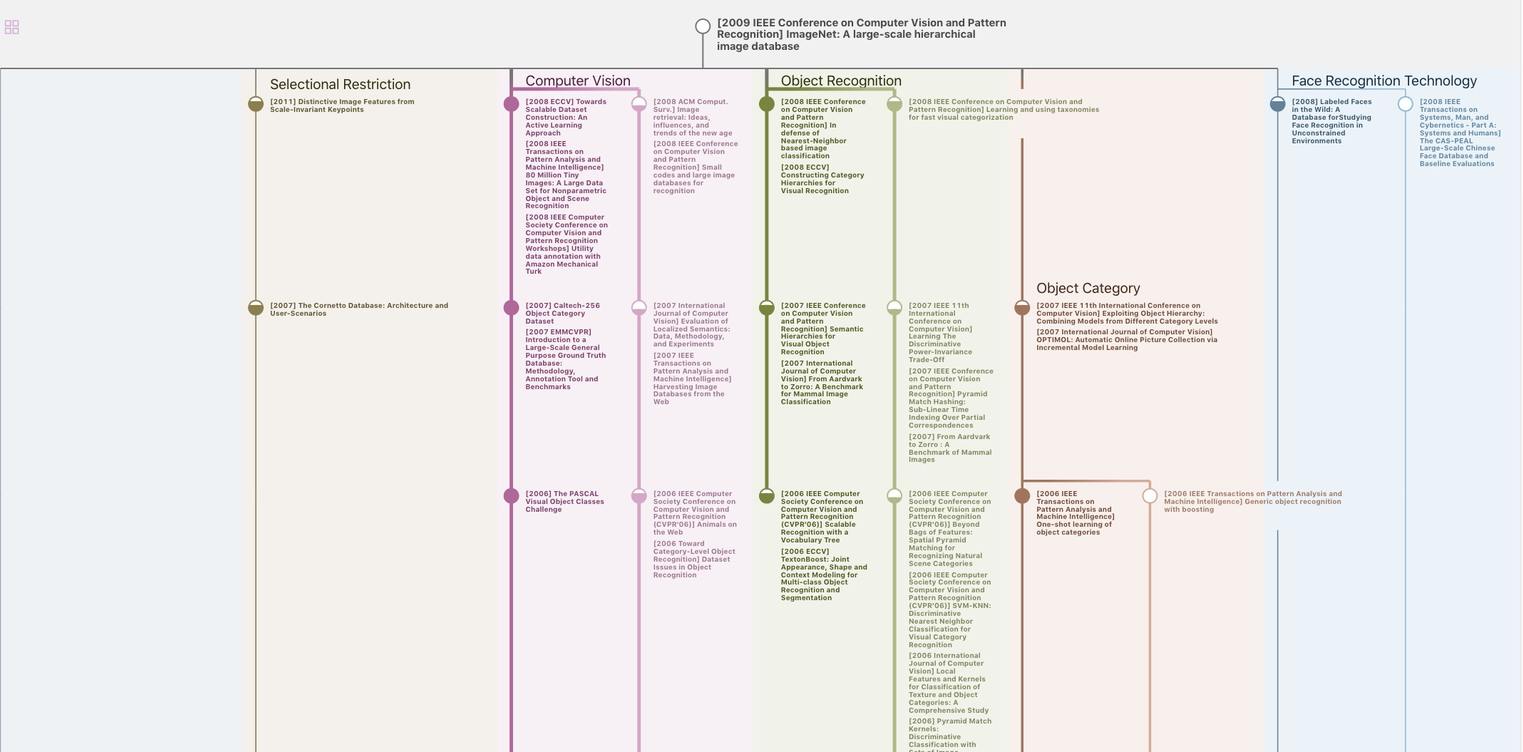
生成溯源树,研究论文发展脉络
Chat Paper
正在生成论文摘要