Convolutional Neural Network for Fully Automated Cerebellar Volumetry in Children in Comparison to Manual Segmentation and Developmental Trajectory of Cerebellar Volumes
Cerebellum (London, England)(2024)
Abstract
The purpose of this study was to develop a fully automated and reliable volumetry of the cerebellum of children during infancy and childhood using deep learning algorithms in comparison to manual segmentation. In addition, the clinical usefulness of measuring the cerebellar volume is shown. One hundred patients (0 to 16.3 years old) without infratentorial signal abnormalities on conventional MRI were retrospectively selected from our pool of pediatric MRI examinations. Based on a routinely acquired 3D T1-weighted magnetization prepared rapid gradient echo (MPRAGE) sequence, the cerebella were manually segmented using ITK-SNAP. The data set of all 100 cases was divided into four splits (four-fold cross-validation) to train the network (NN) to delineate the boundaries of the cerebellum. First, the accuracy of the newly created neural network was compared with the manual segmentation. Secondly, age-related volume changes were investigated. Our trained NN achieved an excellent Spearman correlation coefficient of 0.99, a Dice Coefficient of 95.0 +/- 2.1%, and an intersection over union (IoU) of 90.6 +/- 3.8%. Cerebellar volume increased continuously with age, showing an exponentially rapid growth within the first year of life. Using a convolutional neural network, it was possible to achieve reliable, fully automated cerebellar volume measurements in childhood and infancy, even when based on a relatively small cohort. In this preliminary study, age-dependent cerebellar volume changes could be acquired.
MoreTranslated text
Key words
Cerebellum,Magnetic resonance imaging,Automated segmentation,Neural network,Pediatrics
AI Read Science
Must-Reading Tree
Example
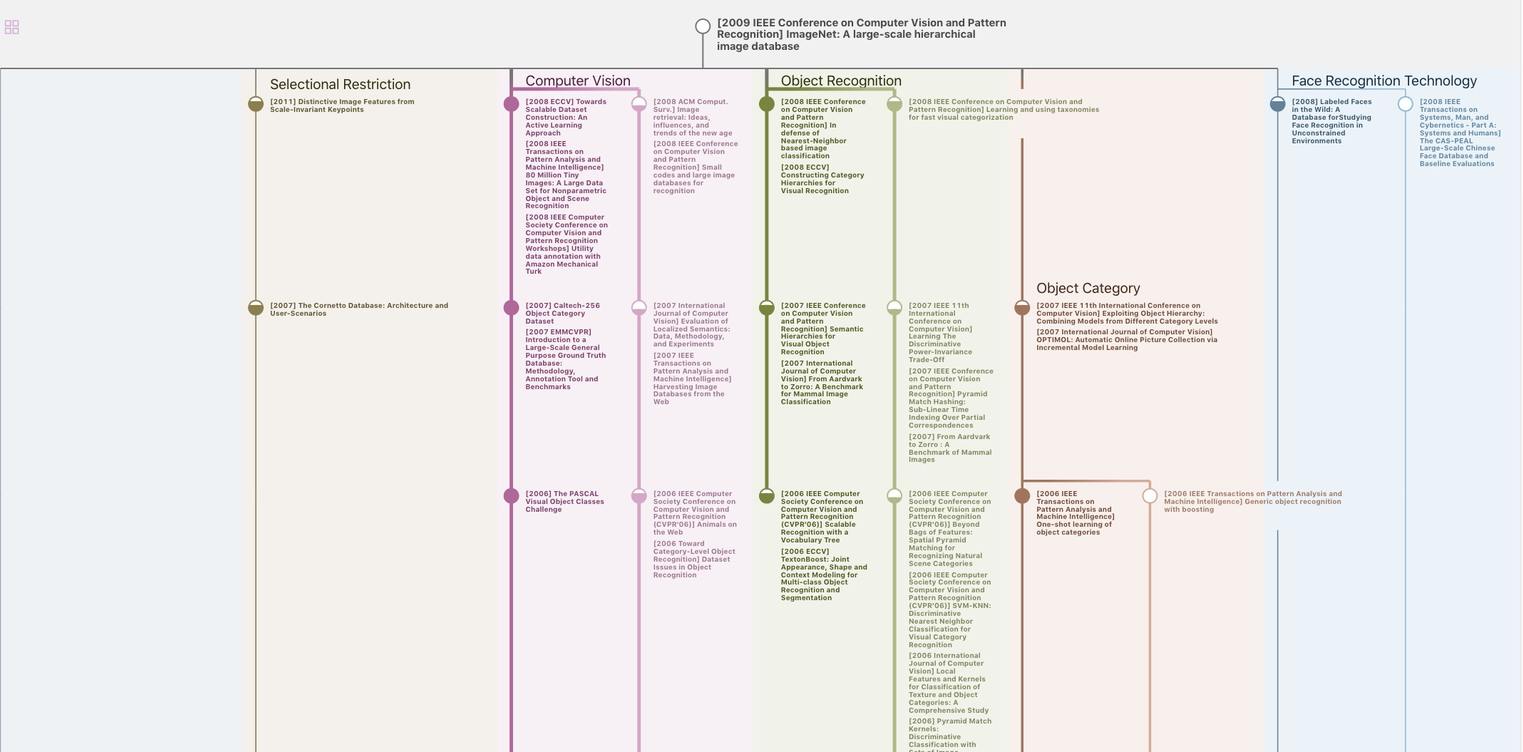
Generate MRT to find the research sequence of this paper
Chat Paper
Summary is being generated by the instructions you defined