A differential evolution with autonomous strategy selection and its application in remote sensing image denoising
EXPERT SYSTEMS WITH APPLICATIONS(2024)
摘要
Differential evolution (DE) is an efficient and effective global optimization algorithm, and many DE variants with adaptive strategy selection have been proposed. However, among the existing DE variants with adaptive strategy selection, only a few last generations of population information are utilized to conduct statistical analysis for strategy selection, and the population cumulative historical experience information does not be fully used to assist population to effectively search. In this paper, a DE with autonomous strategy selection (ASS-DE) is proposed to make full use of the population cumulative historical experience information. In ASS-DE, an individual can autonomously choose the better mutation strategy guided by the cumulative historical experience. As the same time, a parameter updating mechanism with archive is introduced to assign appropriate control parameters to the strategy. Additionally, an evolutionary learning mechanism based on individual similarity is proposed to guide the learning of historical experience information. To verify the performance of ASS-DE, CEC2015 and CEC2017 benchmark functions and the real-world optimization problem of wavelet parameter optimization in remote sensing image denoising is compared with some state-of-the-art intelligence algorithms. The experimental results show that ASS-DE obtains the promising performance compared with other DE variants. The source code of ASS-DE can be found in GitHub at https://github.com/Ramessis/ASS-DE_denoising.git.
更多查看译文
关键词
Differential evolution,Reinforcement learning,Autonomous learning,Mutation strategy,Historical experience,Remote sensing
AI 理解论文
溯源树
样例
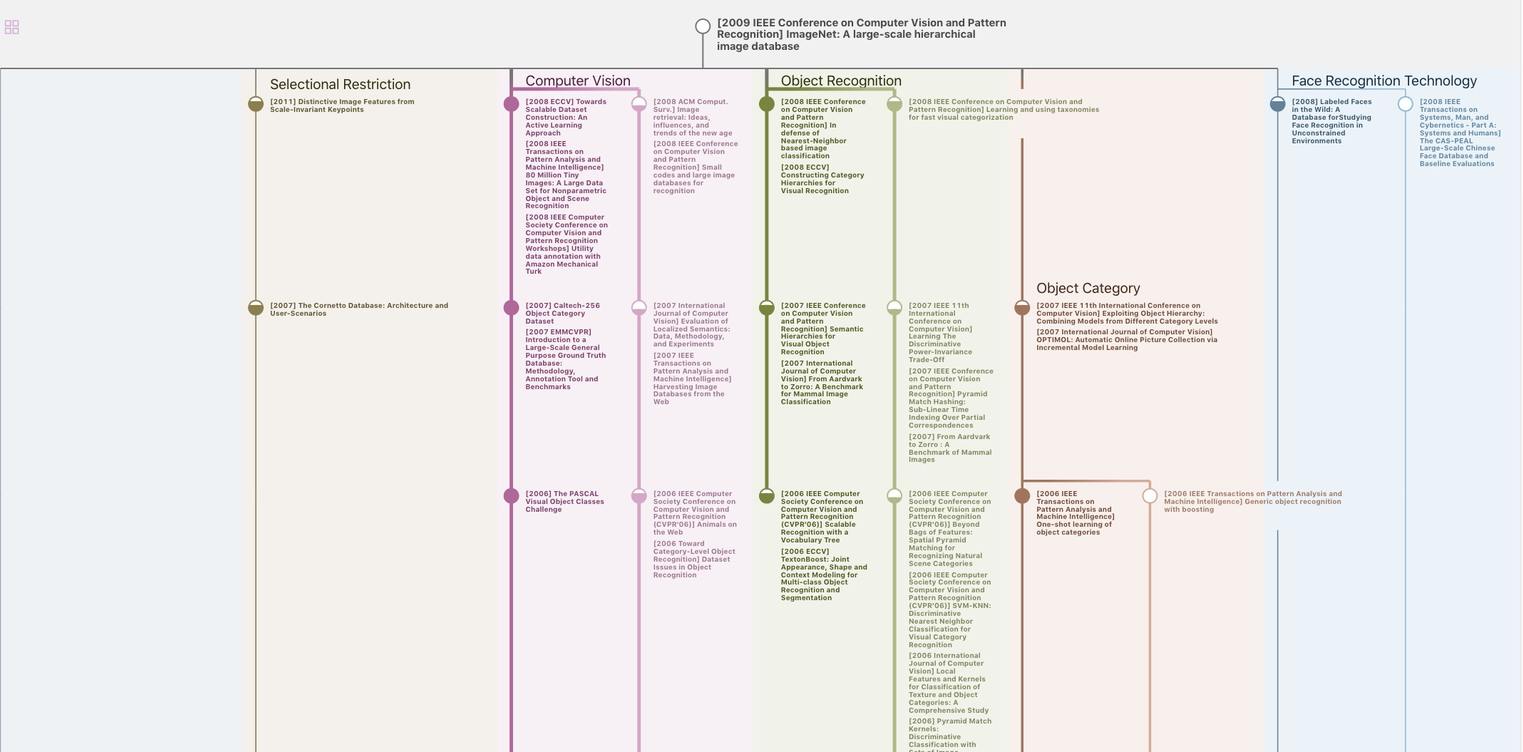
生成溯源树,研究论文发展脉络
Chat Paper
正在生成论文摘要