Toward Label-Efficient Emotion and Sentiment Analysis
Proceedings of the IEEE(2023)
摘要
Emotion and sentiment play a central role in various human activities, such as perception, decision-making, social interaction, and logical reasoning. Developing artificial emotional intelligence (AEI) for machines is becoming a bottleneck in human–computer interaction. The first step of AEI is to recognize the emotion and sentiment that are conveyed in different affective signals. Traditional supervised emotion and sentiment analysis (ESA) methods, especially deep learning-based ones, usually require large-scale labeled training data. However, due to the essential subjectivity, complexity, uncertainty and ambiguity, and subtlety, collecting such annotations is expensive, time-consuming, and difficult in practice. In this article, we introduce label-efficient ESA from the computational perspective. First, we present a hierarchical taxonomy for label-efficient learning based on the availability of sample labels, emotion categories, and data domains during training. Second, for each of the seven paradigms, i.e., unsupervised, semisupervised, weakly supervised, low-shot, incremental, domain-adaptive, and domain-generalizable ESA, we give the definition, summarize existing methods, and present our views on the quantitative and qualitative comparison. Finally, we provide several promising real-world applications, followed by unsolved challenges and potential future directions.
更多查看译文
关键词
| Affective computing, artificial emotional intelligence (AEI), emotion and sentiment analysis (ESA), label-efficient learning
AI 理解论文
溯源树
样例
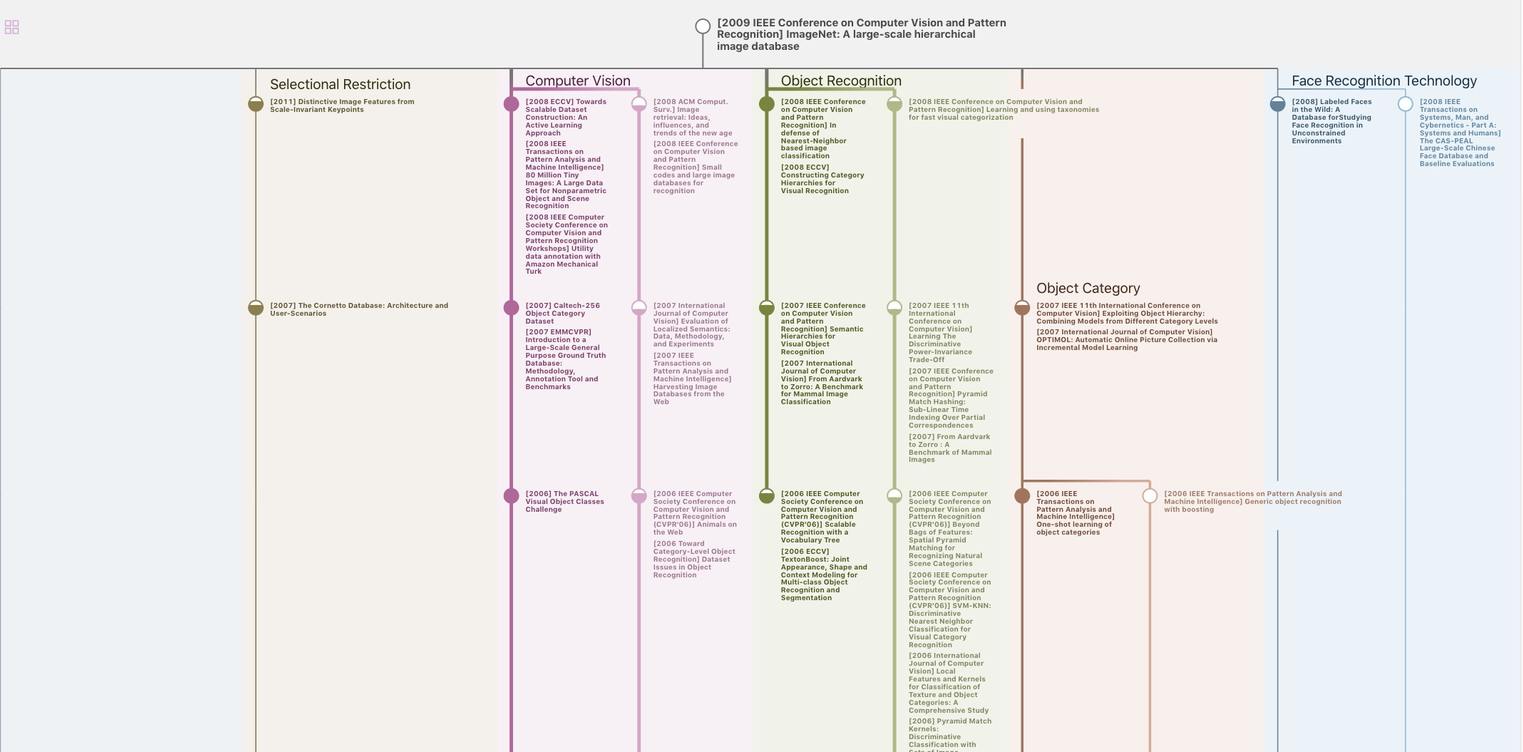
生成溯源树,研究论文发展脉络
Chat Paper
正在生成论文摘要