Statistical Inference of Rate Constants in Chemical and Biochemical Reaction Networks Using an "Inverse" Event-Driven Kinetic Monte Carlo Method
JOURNAL OF PHYSICAL CHEMISTRY B(2023)
摘要
The use of rate models for networks of stochastic reactions is frequently used to comprehend the macroscopically observed dynamic properties of finite size reactive systems as well as their relationship to the underlying molecular events. tau his particular approach usually stumbles on parameter derivation associated with stochastic kinetics, a quite demanding procedure. The present study incorporates a novel algorithm, which infers kinetic parameters from the system's time evolution, manifested as changes in molecular species populations. The proposed methodology reconstructs distributions required to infer kinetic parameters of a stochastic process pertaining to either a simulation or experimental data. The suggested approach accurately replicates rate constants of the stochastic reaction networks, which have evolved over time by event-driven Monte Carlo (MC) simulations using the Gillespie algorithm. Furthermore, our approach has been successfully used to estimate rate constants of association and dissociation events between molecular species developing during molecular dynamics (MD) simulations. We certainly believe that our method will be remarkably helpful for considering the macroscopic characteristic molecular roots related to stochastic physical and biological processes.
更多查看译文
关键词
biochemical reaction networks,rate constants,statistical inference,chemical,event-driven
AI 理解论文
溯源树
样例
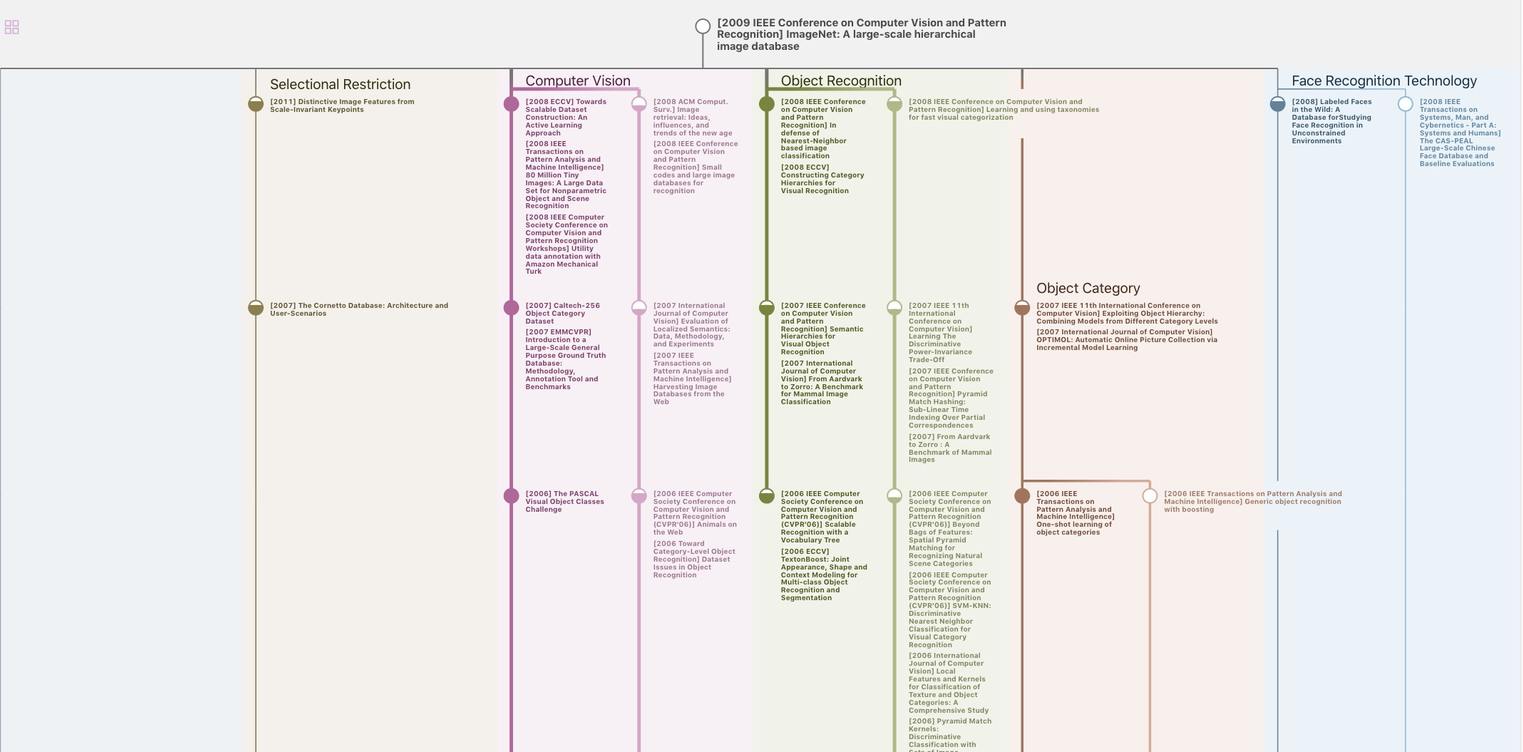
生成溯源树,研究论文发展脉络
Chat Paper
正在生成论文摘要