Quantifying techno-functional properties of ingredients from multiple crops using machine learning
CURRENT RESEARCH IN FOOD SCIENCE(2023)
摘要
Food ingredients with a low degree of refining consist of multiple components. Therefore, it is essential to formulate food products based on techno-functional properties rather than composition. We assessed the potential of quantifying techno-functional properties of ingredient blends from multiple crops as opposed to single crops. The properties quantified were gelation, viscosity, emulsion stability, and foaming capacity of ingredients from yellow pea and lupine seeds. The relationships were quantified using spline regression, random forest, and neural networks. Suitable models were picked based on model accuracy and physical feasibility of model predictions. A single model to quantify the properties of both crops could be created for each techno-functional property, albeit with a trade-off of higher prediction errors as compared to models based on individual crops. A reflection on the number of observations in each dataset showed that they could be reduced for some properties.
更多查看译文
关键词
Food ingredients,Food formulation,Mild fractionation,Techno-functional properties,Machine learning
AI 理解论文
溯源树
样例
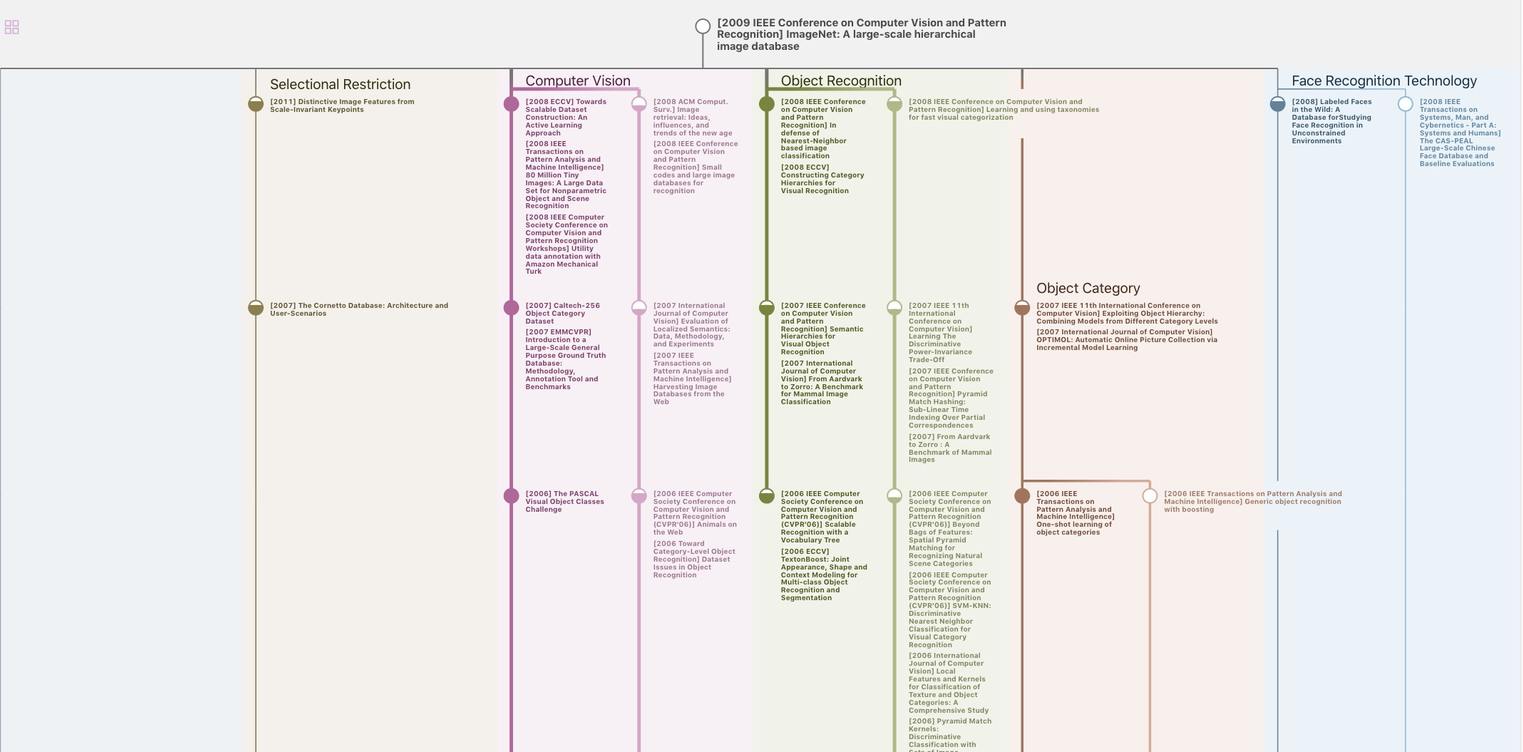
生成溯源树,研究论文发展脉络
Chat Paper
正在生成论文摘要