MAGI: Multi-Annotated Explanation-Guided Learning.
ICCV(2023)
摘要
Explanation supervision is a technique in which the model is guided by human-generated explanations during training. This technique aims to improve the predictability of the model by incorporating human understanding of the prediction process into the training phase. This is a challenging task since it relies on the accuracy of human annotation labels. To obtain high-quality explanation annotations, using multiple annotations to do explanation supervision is a reasonable method. However, how to use multiple annotations to improve accuracy is particularly challenging due to the following: 1) The noisiness of annotations from different annotators; 2) The lack of pre-given information about the corresponding relationship between annotations and annotators; 3) Missing annotations since some images are not labeled by all annotators. To solve these challenges, we propose a Multi-annotated explanation-guided learning (MAGI) framework to do explanation supervision with comprehensive and high-quality generated annotations. We first propose a novel generative model to generate annotations from all annotators and infer them using a newly proposed variational inference-based technique by learning the characteristics of each annotator. We also incorporate an alignment mechanism into the generative model to infer the correspondence between annotations and annotators in the training process. Extensive experiments on two datasets from the medical imaging domain demonstrate the effectiveness of our proposed framework in handling noisy annotations while obtaining superior prediction performance compared with previous SOTA.
更多查看译文
AI 理解论文
溯源树
样例
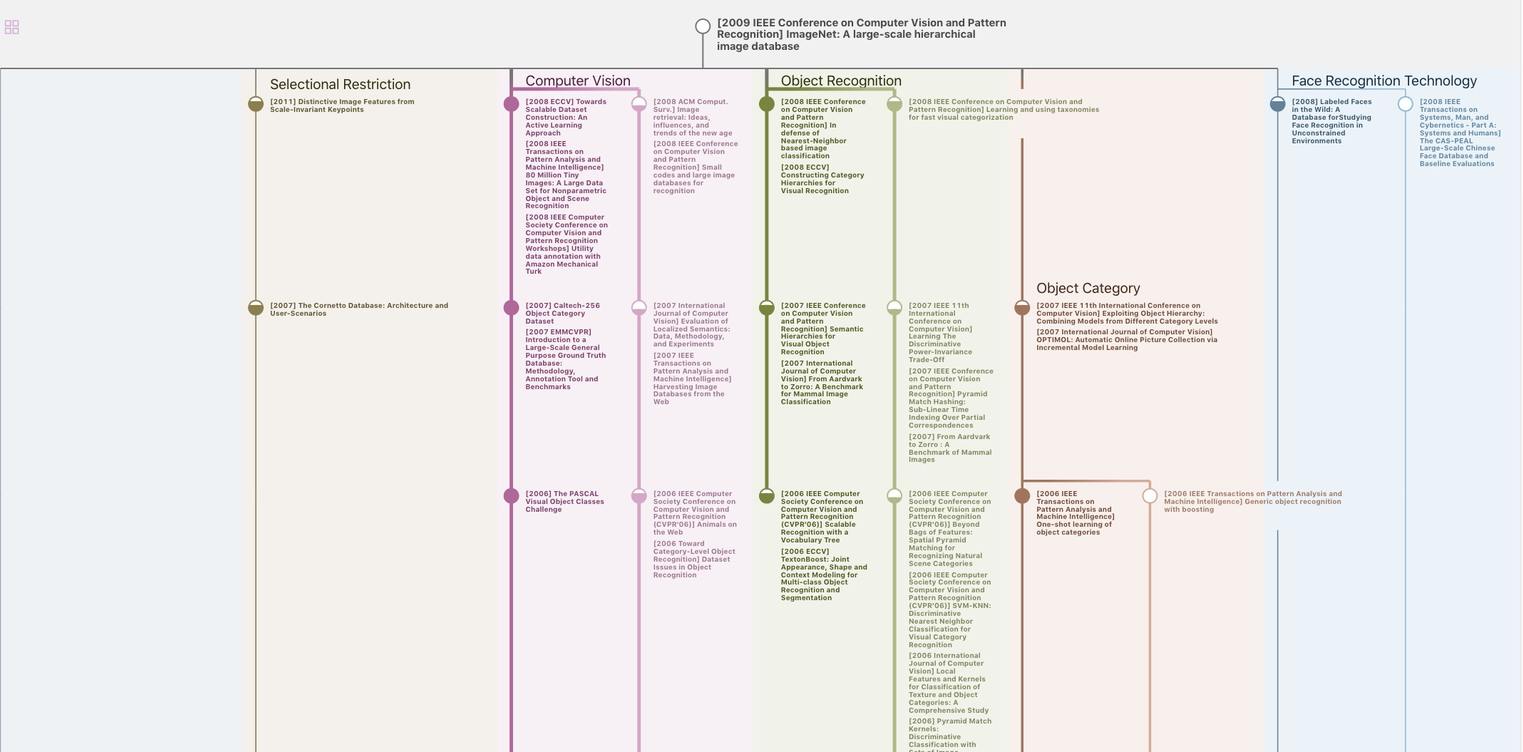
生成溯源树,研究论文发展脉络
Chat Paper
正在生成论文摘要