Lens Parameter Estimation for Realistic Depth of Field Modeling.
ICCV(2023)
摘要
We present a method to estimate the depth of field effect from a single image. Most existing methods related to this task provide either a per-pixel estimation of blur and/or depth. Instead, we go further and propose to use a lens-based representation that models the depth of field using two parameters: the blur factor and focus disparity. Those two parameters, along with the signed defocus representation, result in a more intuitive and linear representation which we solve using a novel weighting network. Furthermore, our method explicitly enforces consistency between the estimated defocus blur, the lens parameters, and the depth map. Finally, we train our deep-learning-based model on a mix of real images with synthetic depth of field and fully synthetic images. These improvements result in a more robust and accurate method, as demonstrated by our state-of-the-art results. In particular, our lens parametrization enables several applications, such as 3D staging for AR environments and seamless object compositing.
更多查看译文
AI 理解论文
溯源树
样例
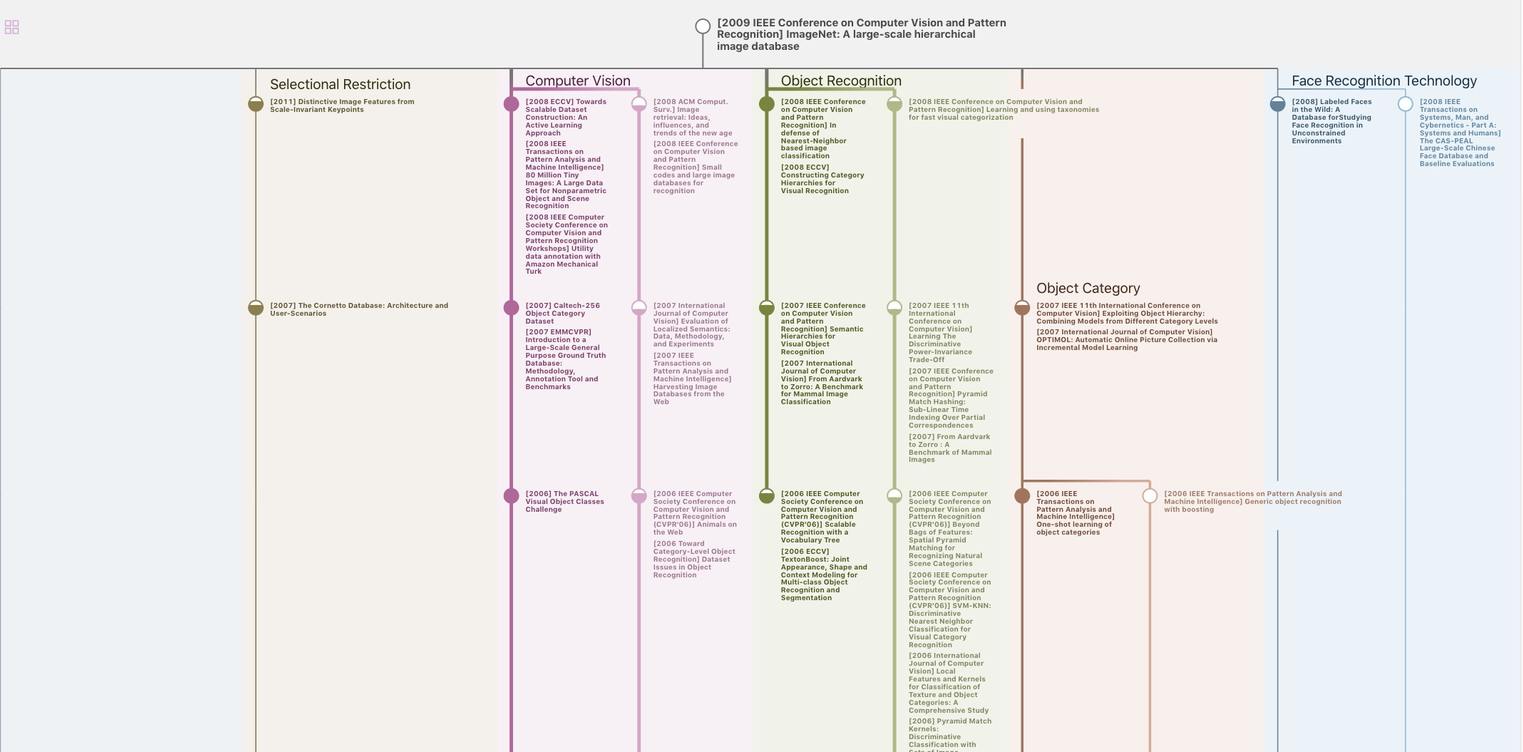
生成溯源树,研究论文发展脉络
Chat Paper
正在生成论文摘要