Uncertainty-guided Learning for Improving Image Manipulation Detection.
Proceedings of the IEEE/CVF International Conference on Computer Vision (ICCV)(2023)
摘要
Image manipulation detection (IMD) is of vital importance as faking images and spreading misinformation can be malicious and harm our daily life. IMD is the core technique to solve these issues and poses challenges in two main aspects: (1) Data Uncertainty, i.e., the manipulated artifacts are often hard for humans to discern and lead to noisy labels, which may disturb model training; (2) Model Uncertainty, i.e., the same object may hold different categories (tampered or not) due to manipulation operations, which could potentially confuse the model training and result in unreliable outcomes. Previous works mainly focus on solving the model uncertainty issue by designing meticulous features and networks, however, the data uncertainty problem is rarely considered. In this paper, we address both problems by introducing an uncertainty-guided learning framework, which measures data and model uncertainties by a novel Uncertainty Estimation Network (UEN). UEN is trained under dynamic supervision, and outputs estimated uncertainty maps to refine manipulation detection results, which significantly alleviates the learning difficulties. To our knowledge, this is the first work to embed uncertainty modeling into IMD. Extensive experiments on various datasets demonstrate state-of-the-art performance, validating the effectiveness and generalizability of our method.
更多查看译文
AI 理解论文
溯源树
样例
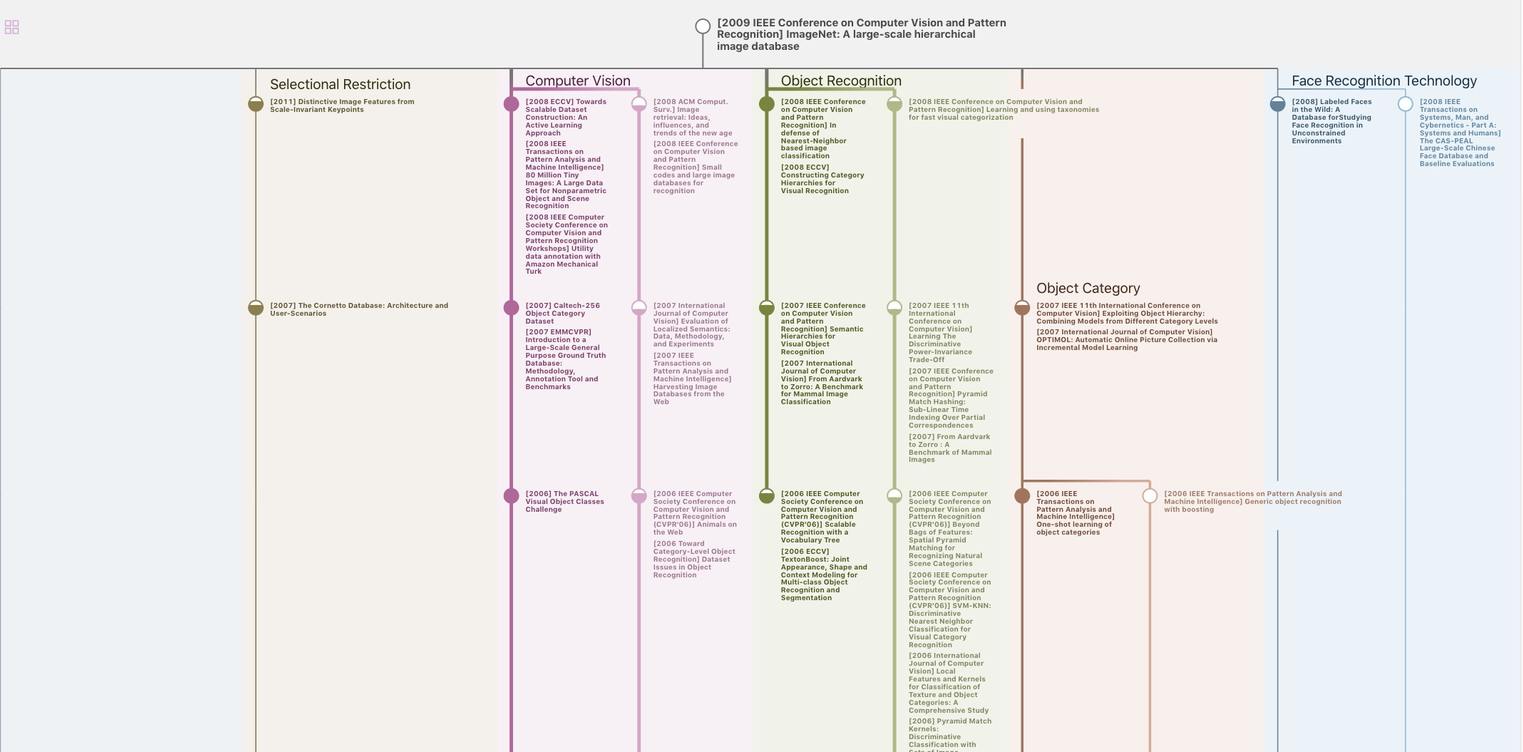
生成溯源树,研究论文发展脉络
Chat Paper
正在生成论文摘要