Learning Concordant Attention via Target-aware Alignment for Visible-Infrared Person Re-identification.
ICCV(2023)
摘要
Owing to the large distribution gap between the heterogeneous data in Visible-Infrared Person Re-identification (VI Re-ID), we point out that existing paradigms often suffer from the inter-modal semantic misalignment issue and thus fail to align and compare local details properly. In this paper, we present Concordant Attention Learning (CAL), a novel framework that learns semantic-aligned representations for VI Re-ID. Specifically, we design the Target-aware Concordant Alignment paradigm, which allows target-aware attention adaptation when aligning heterogeneous samples (i.e., adaptive attention adjustment according to the target image being aligned). This is achieved by exploiting the discriminative clues from the modality counterpart and designing effective modality-agnostic correspondence searching strategies. To ensure semantic concordance during the cross-modal retrieval stage, we further propose MatchDistill, which matches the attention patterns across modalities and learns their underlying semantic correlations by bipartite-graph-based similarity modeling and cross-modal knowledge exchange. Extensive experiments on VI Re-ID benchmark datasets demonstrate the effectiveness and superiority of the proposed CAL.
更多查看译文
AI 理解论文
溯源树
样例
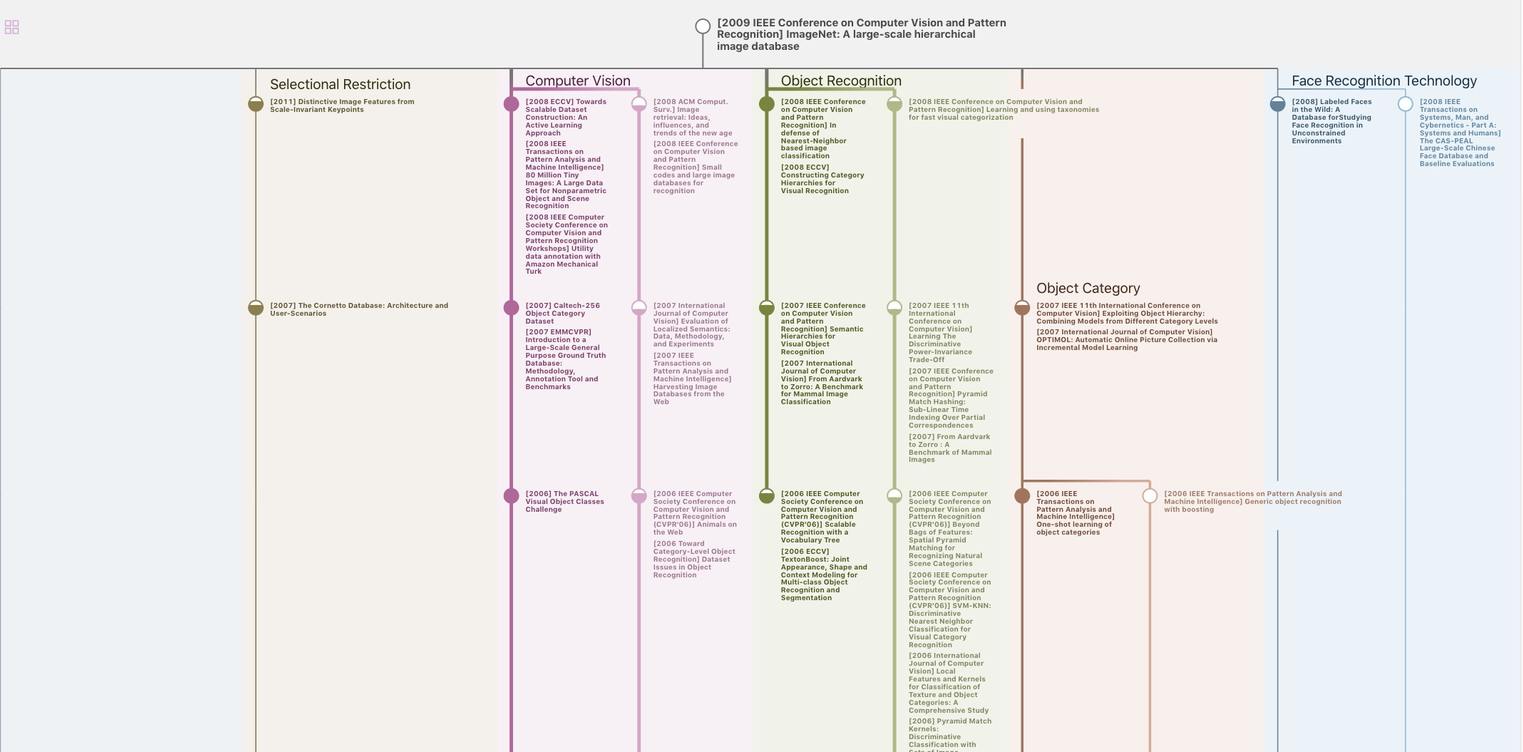
生成溯源树,研究论文发展脉络
Chat Paper
正在生成论文摘要