Adverse Weather Removal with Codebook Priors.
ICCV(2023)
摘要
Despite recent advancements in unified adverse weather removal methods, there remains a significant challenge of achieving realistic fine-grained texture and reliable background reconstruction to mitigate serious distortions.Inspired by recent advancements in codebook and vector quantization (VQ) techniques, we present a novel Adverse Weather Removal network with Codebook Priors (AWRCP) to address the problem of unified adverse weather removal. AWRCP leverages high-quality codebook priors derived from undistorted images to recover vivid texture details and faithful background structures. However, simply utilizing high-quality features from the codebook does not guarantee good results in terms of fine-grained details and structural fidelity. Therefore, we develop a deformable cross-attention with sparse sampling mechanism for flexible perform feature interaction between degraded features and high-quality features from codebook priors. In order to effectively incorporate high-quality texture features while maintaining the realism of the details generated by codebook priors, we propose a hierarchical texture warping head that gradually fuses hierarchical codebook prior features into high-resolution features at final restoring stage.With the utilization of the VQ codebook as a feature dictionary of high quality and the proposed designs, AWRCP can largely improve the restored quality of texture details, achieving the state-of-the-art performance across multiple adverse weather removal benchmark.
更多查看译文
AI 理解论文
溯源树
样例
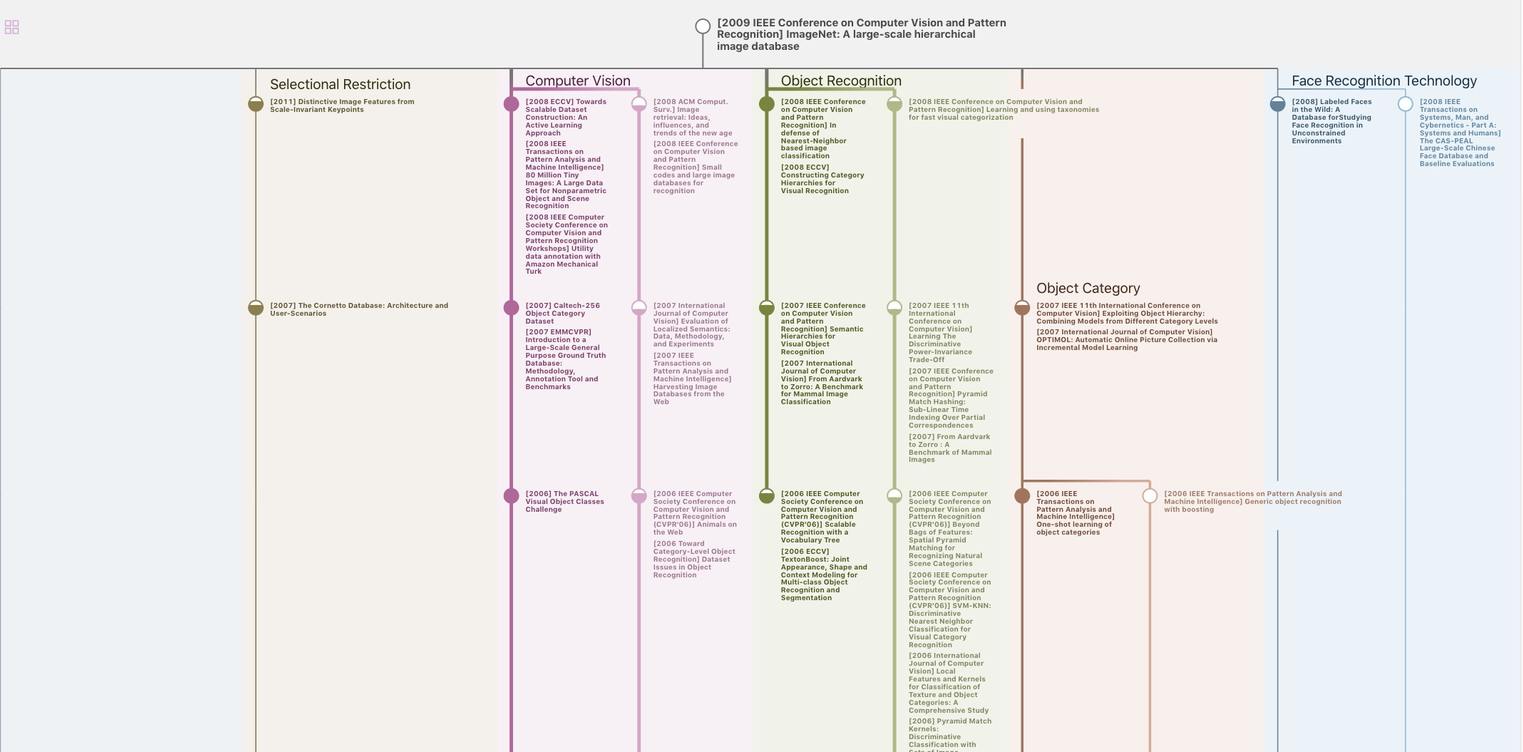
生成溯源树,研究论文发展脉络
Chat Paper
正在生成论文摘要