How Far Pre-trained Models Are from Neural Collapse on the Target Dataset Informs their Transferability.
ICCV(2023)
摘要
This paper focuses on model transferability estimation, i.e., assessing the performance of pre-trained models on a downstream task without performing fine-tuning. Motivated by neural collapse (NC) [25] that reveals particular feature geometry at the terminal stage of training, we consider model transferability as how far the target activations obtained by pre-trained models are from their hypothetical state in the terminal phase of the model fine-tuned on the target domain. We propose a metric that measures this proximity based on three phenomena of NC: within-class variability collapse, simplex encoded label interpolation geometry structure is formed, and the nearest center classifier becomes optimal on training data. Through experiments on 11 datasets, we confirm none of the three NC proxies are dispensable, which allows us to obtain very competitive transferability estimation accuracy with approximately 10× wall-clock time speed up compared to state-of-the-art approaches.
更多查看译文
AI 理解论文
溯源树
样例
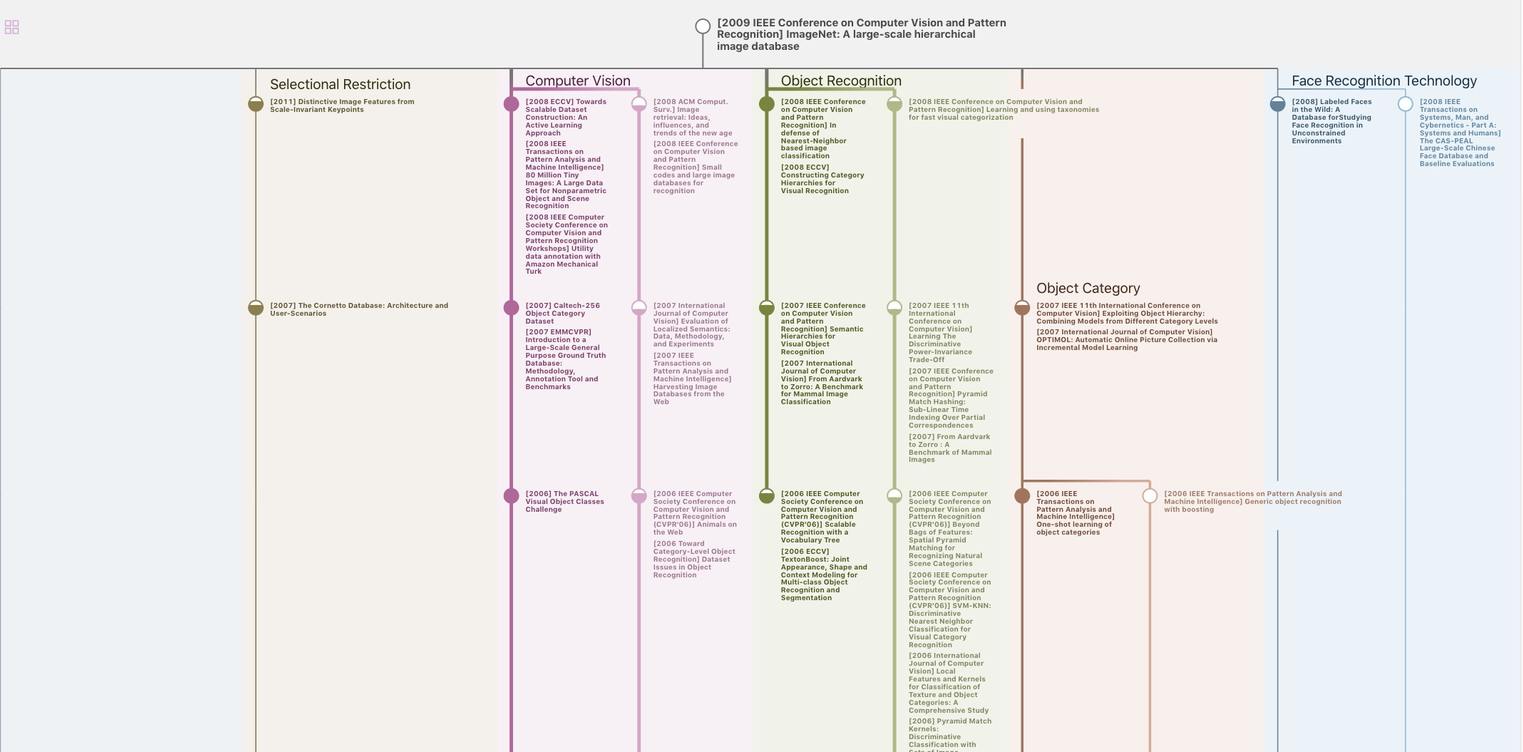
生成溯源树,研究论文发展脉络
Chat Paper
正在生成论文摘要