WaveIPT: Joint Attention and Flow Alignment in the Wavelet domain for Pose Transfer.
Proceedings of the IEEE/CVF International Conference on Computer Vision (ICCV)(2023)
摘要
Human pose transfer aims to synthesis a new image of the source person in a target pose. Among the various existing methods, attention and flow have emerged as two of the most popular and effective approaches. Attention excels at preserving the semantic structure of the source image, which is more reflected in the low-frequency domain. Contrastively, flow is better at retaining fine-grained texture details in the high-frequency domain. To leverage the advantages of both attention and flow simultaneously, this paper proposes Wavelet-aware Image-based Pose Transfer (WaveIPT) as a novel approach to fuse the attention and flow in the wavelet domain. To improve the fusion effect and avoid interference from irrelevant information across different frequencies, WaveIPT first applies Intra-scale Local Correlation (ILC) to adaptively fuse attention and flow in the same scale according to their strengths in low and high-frequency domains. Subsequently, WaveIPT employs Inter-scale Feature Interaction (IFI) to explore inter-scale frequency features, facilitating effective information transfer across different scales. Furthermore, we introduce Progressive Flow Regularization (PFR), an effective method that alleviates the challenges of flow estimation under large pose differences. The experiments on the DeepFashion dataset demonstrate that WaveIPT achieves a new state-of-the-art in terms of both FID and LPIPS, with improvements of 4.97% and 3.89%, respectively.
更多查看译文
AI 理解论文
溯源树
样例
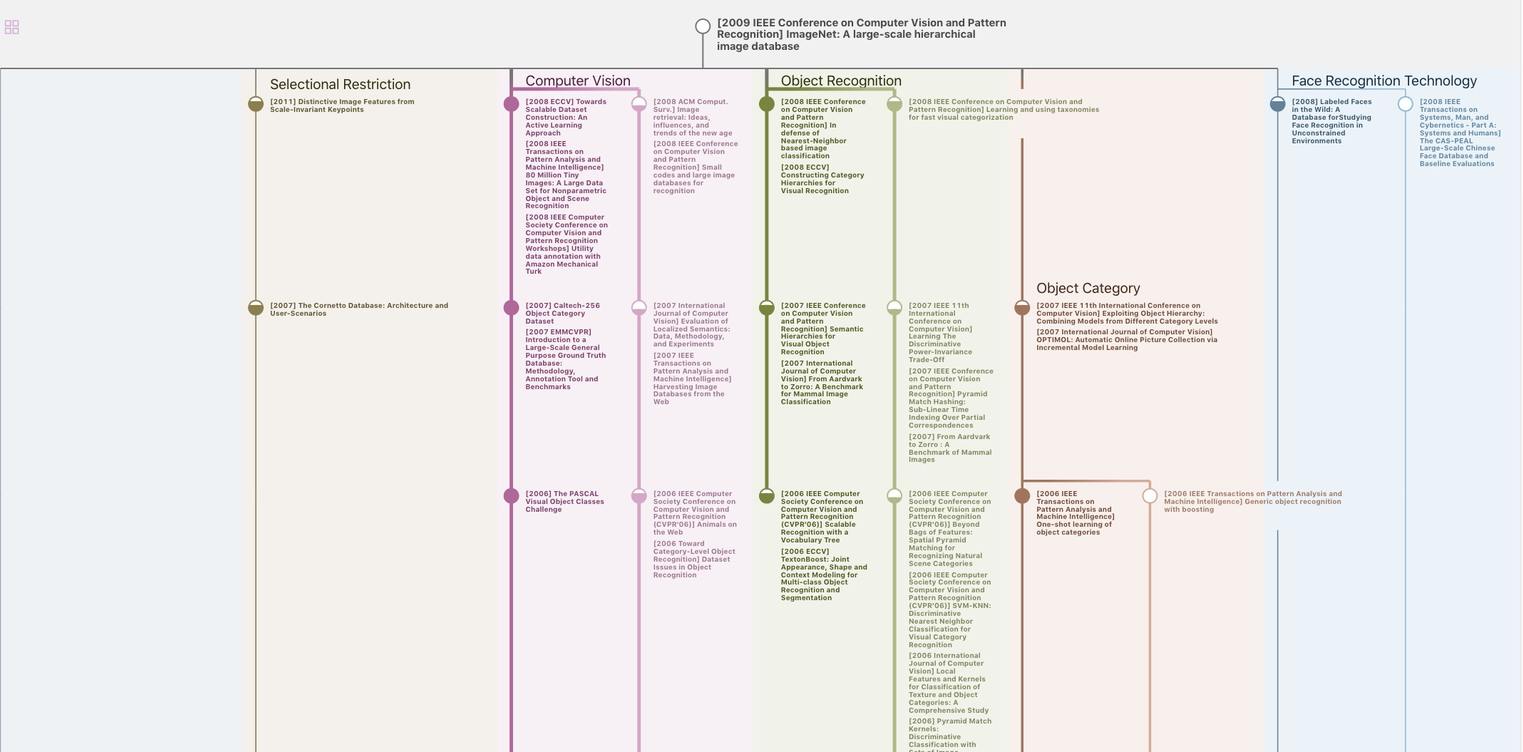
生成溯源树,研究论文发展脉络
Chat Paper
正在生成论文摘要