μSplit: image decomposition for fluorescence microscopy.
Proceedings of the IEEE/CVF International Conference on Computer Vision (ICCV)(2023)
摘要
We present μSplit, a dedicated approach for trained image decomposition in the context of fluorescence microscopy images. We find that best results using regular deep architectures are achieved when large image patches are used during training, making memory consumption the limiting factor to further improving performance. We therefore introduce lateral contextualization (LC), a novel meta-architecture that enables the memory efficient incorporation of large image-context, which we observe is a key ingredient to solving the image decomposition task at hand. We integrate LC with U-Nets, Hierarchical AEs, and Hierarchical VAEs, for which we formulate a modified ELBO loss. Additionally, LC enables training deeper hierarchical models than otherwise possible and, interestingly, helps to reduce tiling artefacts that are inherently impossible to avoid when using tiled VAE predictions. We apply μSplit to five decomposition tasks, one on a synthetic dataset, four others derived from real microscopy data. Our method consistently achieves best results (average improvements to the best baseline of 2.25 dB PSNR), while simultaneously requiring considerably less GPU memory. Our code and datasets can be found at https://github.com/juglab/uSplit.
更多查看译文
AI 理解论文
溯源树
样例
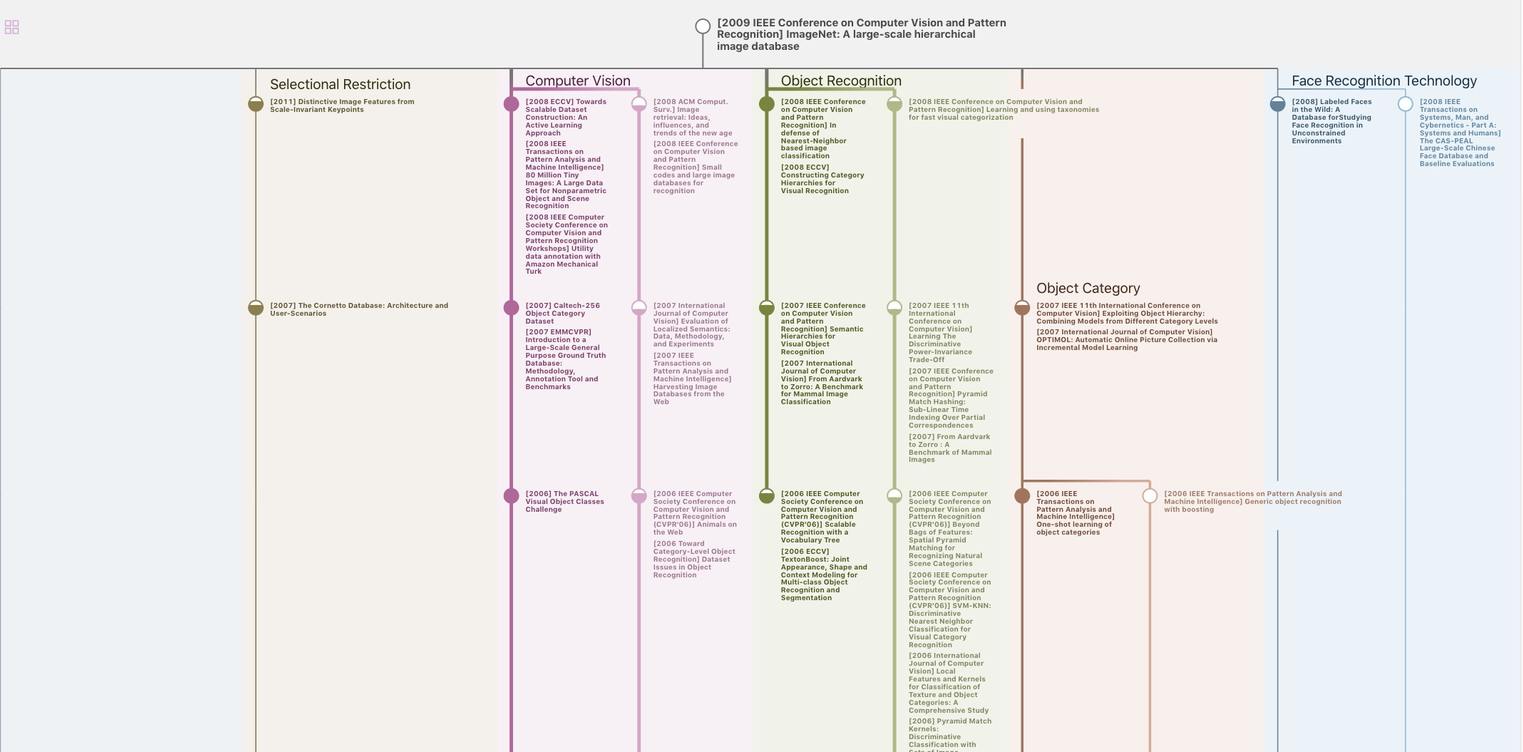
生成溯源树,研究论文发展脉络
Chat Paper
正在生成论文摘要