Towards Unsupervised Domain Generalization for Face Anti-Spoofing.
Proceedings of the IEEE/CVF International Conference on Computer Vision (ICCV)(2023)
摘要
Generalizable face anti-spoofing (FAS) based on domain generalization (DG) has gained growing attention due to its robustness in real-world applications. However, these DG methods rely heavily on labeled source data, which are usually costly and hard to access. Comparably, unlabeled face data are far more accessible in various scenarios. In this paper, we propose the first Unsupervised Domain Generalization framework for Face Anti-Spoofing, namely UDG-FAS, which could exploit large amounts of easily accessible unlabeled data to learn generalizable features for enhancing the low-data regime of FAS. Yet without supervision signals, learning intrinsic live/spoof features from complicated facial information is challenging, which is even tougher in cross-domain scenarios due to domain shift. Existing unsupervised learning methods tend to learn identity-biased and domain-biased features as shortcuts, and fail to specify spoof cues. To this end, we propose a novel Split-Rotation-Merge module to build identity-agnostic local representations for mining intrinsic spoof cues and search the nearest neighbors in the same domain as positives for mitigating the identity bias. Moreover, we propose to search cross-domain neighbors with domain-specific normalization and merged local features to learn a domain-invariant feature space. To our best knowledge, this is the first attempt to learn generalized FAS features in a fully unsupervised way. Extensive experiments show that UDG-FAS significantly outperforms state-of-the-art methods on six diverse practical protocols.
更多查看译文
AI 理解论文
溯源树
样例
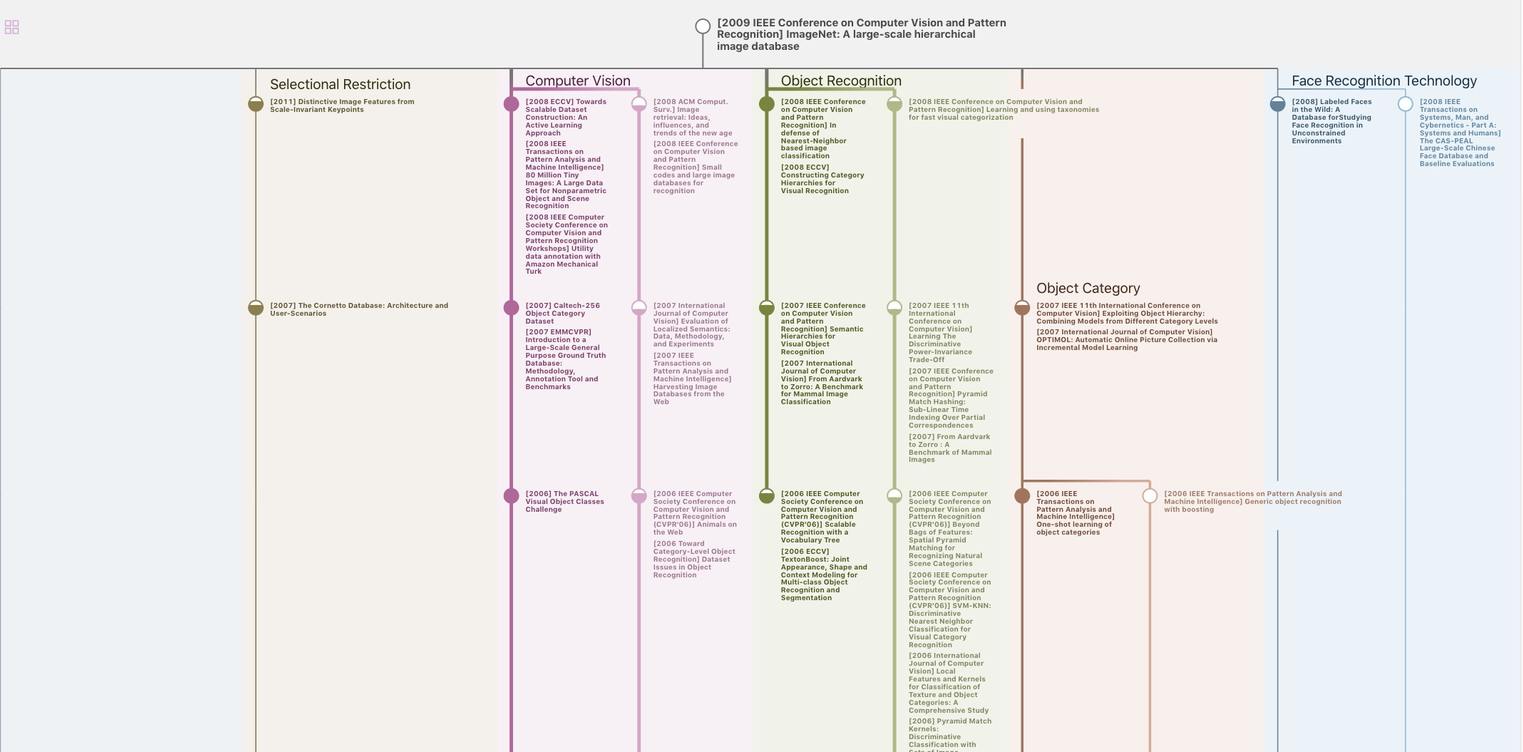
生成溯源树,研究论文发展脉络
Chat Paper
正在生成论文摘要