Most Important Person-guided Dual-branch Cross-Patch Attention for Group Affect Recognition.
Proceedings of the IEEE/CVF International Conference on Computer Vision (ICCV)(2023)
摘要
Group affect refers to the subjective emotion that is evoked by an external stimulus in a group, which is an important factor that shapes group behavior and outcomes. Recognizing group affect involves identifying important individuals and salient objects among a crowd that can evoke emotions. However, most existing methods lack attention to affective meaning in group dynamics and fail to account for the contextual relevance of faces and objects in group-level images. In this work, we propose a solution by incorporating the psychological concept of the Most Important Person (MIP), which represents the most noteworthy face in a crowd and has affective semantic meaning. We present the Dual-branch Cross-Patch Attention Transformer (DCAT) which uses global image and MIP together as inputs. Specifically, we first learn the informative facial regions produced by the MIP and the global context separately. Then, the Cross-Patch Attention module is proposed to fuse the features of MIP and global context together to complement each other. Our proposed method outperforms state-of-the-art methods on GAF 3.0, GroupEmoW, and HECO datasets. Moreover, we demonstrate the potential for broader applications by showing that our proposed model can be transferred to another group affect task, group cohesion, and achieve comparable results.
更多查看译文
关键词
affect,group,attention,recognition,dual-branch,cross-patch
AI 理解论文
溯源树
样例
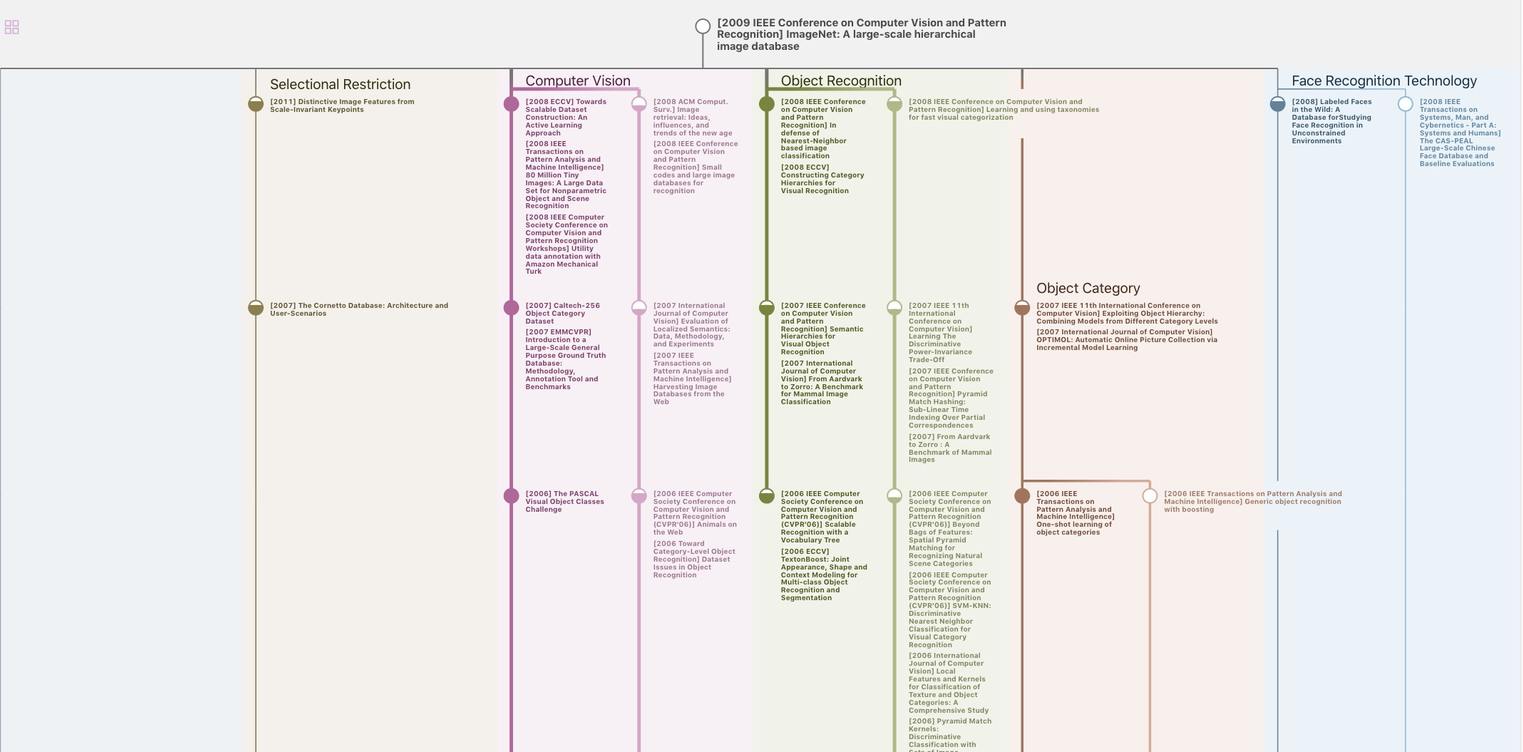
生成溯源树,研究论文发展脉络
Chat Paper
正在生成论文摘要