Lifelong Audio-video Masked Autoencoder with Forget-robust Localized Alignments
CoRR(2023)
摘要
We present a lifelong audio-video masked autoencoder that continually learns the multimodal representations from a video stream containing audio-video pairs, while its distribution continually shifts over time. Specifically, we propose two novel ideas to tackle the problem: (1) Localized Alignment: We introduce a small trainable multimodal encoder that predicts the audio and video tokens that are well-aligned with each other. This allows the model to learn only the highly correlated audiovisual patches with accurate multimodal relationships. (2) Forget-robust multimodal patch selection: We compare the relative importance of each audio-video patch between the current and past data pair to mitigate unintended drift of the previously learned audio-video representations. Our proposed method, FLAVA (Forget-robust Localized Audio-Video Alignment), therefore, captures the complex relationships between the audio and video modalities during training on a sequence of pre-training tasks while alleviating the forgetting of learned audiovisual correlations. Our experiments validate that FLAVA outperforms the state-of-the-art continual learning methods on several benchmark datasets under continual audio-video representation learning scenarios.
更多查看译文
关键词
audio-video,forget-robust
AI 理解论文
溯源树
样例
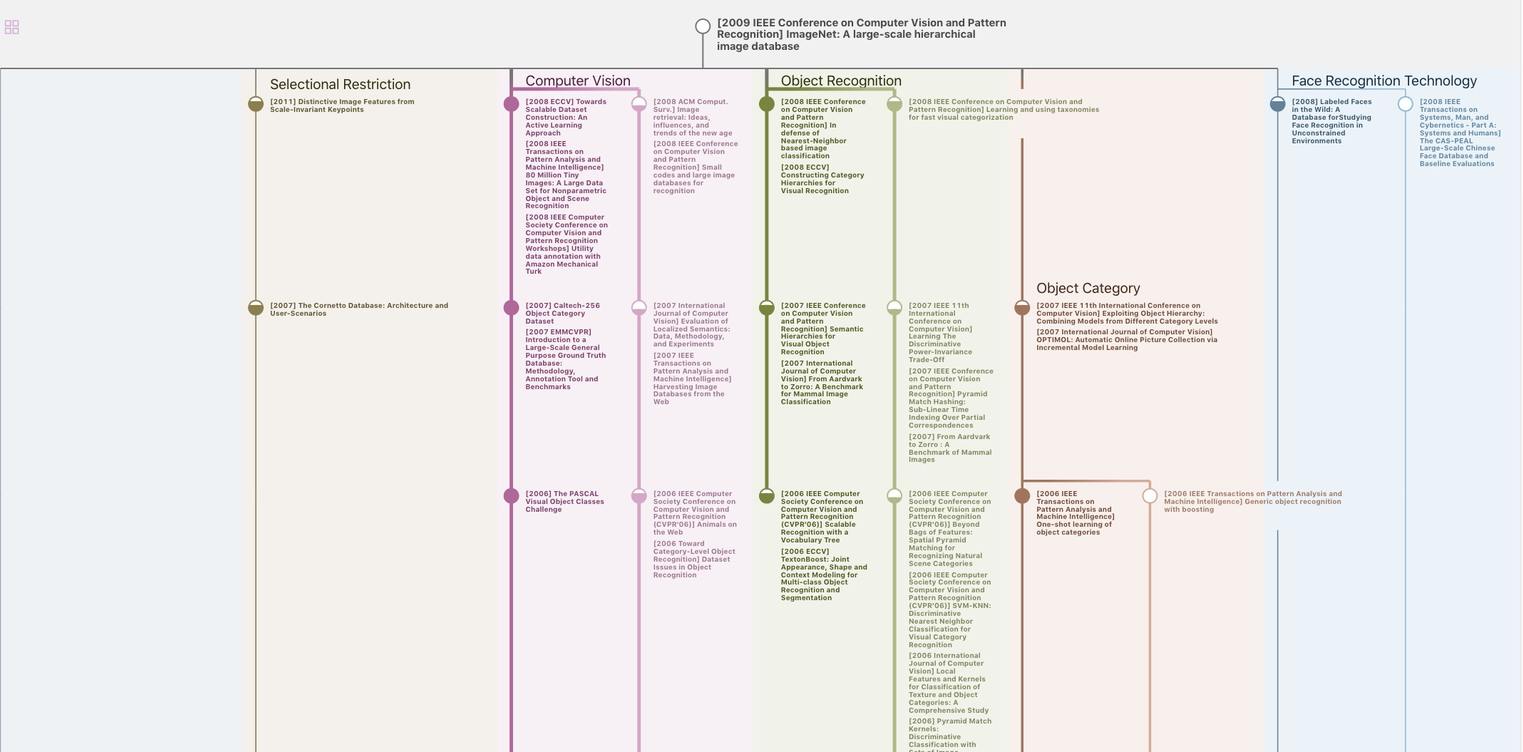
生成溯源树,研究论文发展脉络
Chat Paper
正在生成论文摘要