Sentinel: An Aggregation Function to Secure Decentralized Federated Learning
CoRR(2023)
摘要
The rapid integration of Federated Learning (FL) into networking encompasses various aspects such as network management, quality of service, and cybersecurity while preserving data privacy. In this context, Decentralized Federated Learning (DFL) emerges as an innovative paradigm to train collaborative models, addressing the single point of failure limitation. However, the security and trustworthiness of FL and DFL are compromised by poisoning attacks, negatively impacting its performance. Existing defense mechanisms have been designed for centralized FL and they do not adequately exploit the particularities of DFL. Thus, this work introduces Sentinel, a defense strategy to counteract poisoning attacks in DFL. Sentinel leverages the accessibility of local data and defines a three-step aggregation protocol consisting of similarity filtering, bootstrap validation, and normalization to safeguard against malicious model updates. Sentinel has been evaluated with diverse datasets and various poisoning attack types and threat levels, improving the state-of-the-art performance against both untargeted and targeted poisoning attacks.
更多查看译文
关键词
federated
AI 理解论文
溯源树
样例
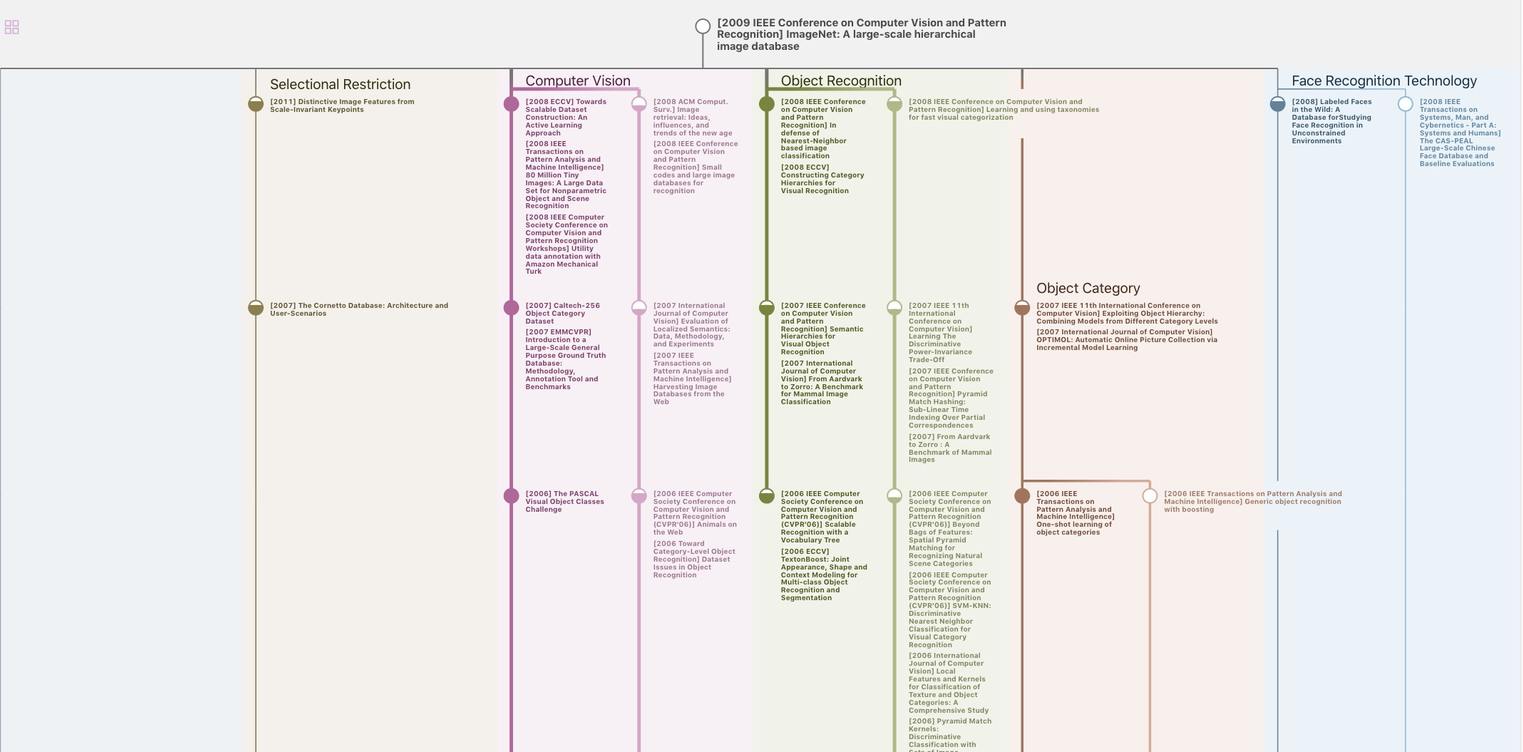
生成溯源树,研究论文发展脉络
Chat Paper
正在生成论文摘要