Hierarchical planning-scheduling-control—Optimality surrogates and derivative-free optimization
Computers & Chemical Engineering(2024)
Abstract
Planning, scheduling, and control typically constitute separate decision-making units within chemical companies. Traditionally, their integration is modelled sequentially, but recent efforts prioritize lower-level feasibility and optimality, leading to large-scale, potentially multi-level, hierarchical formulations. Data-driven techniques, like optimality surrogates or derivative-free optimization, become essential in addressing ensuing tractability challenges. We demonstrate a step-by-step workflow to find a tractable solution to a tri-level formulation of a multi-site, multi-product planning-scheduling-control case study. We discuss solution tractability-accuracy trade-offs and scaling properties for both methods. Despite individual improvements over conventional heuristics, both approaches present drawbacks. Consequently, we synthesize our findings into a methodology combining their strengths. Our approach remains agnostic to the level-specific formulations when the linking variables are identified and retains the heuristic sequential solution as fallback option. We advance the field by leveraging parallelization, hyperparameter tuning, and a combination of off- and on-line computation, to find tractable solutions to more accurate multi-level formulations.
MoreTranslated text
Key words
Black-box optimization,Optimization with embedded surrogates,Integrating operations and control
AI Read Science
Must-Reading Tree
Example
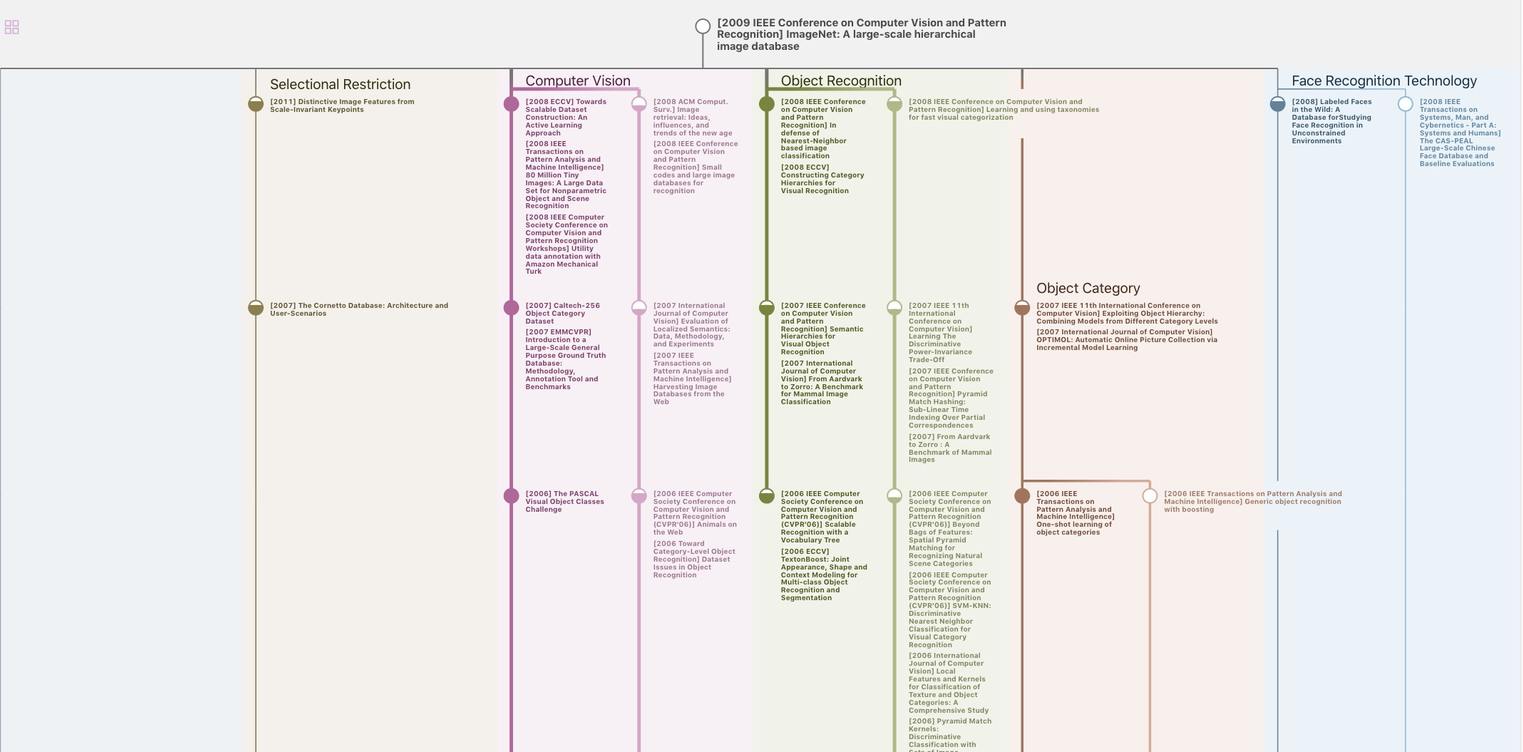
Generate MRT to find the research sequence of this paper
Chat Paper
Summary is being generated by the instructions you defined