Does Synthetic Data Make Large Language Models More Efficient?
CoRR(2023)
摘要
Natural Language Processing (NLP) has undergone transformative changes with the advent of deep learning methodologies. One challenge persistently confronting researchers is the scarcity of high-quality, annotated datasets that drive these models. This paper explores the nuances of synthetic data generation in NLP, with a focal point on template-based question generation. By assessing its advantages, including data augmentation potential and the introduction of structured variety, we juxtapose these benefits against inherent limitations, such as the risk of overfitting and the constraints posed by pre-defined templates. Drawing from empirical evaluations, we demonstrate the impact of template-based synthetic data on the performance of modern transformer models. We conclude by emphasizing the delicate balance required between synthetic and real-world data, and the future trajectories of integrating synthetic data in model training pipelines. The findings aim to guide NLP practitioners in harnessing synthetic data's potential, ensuring optimal model performance in diverse applications.
更多查看译文
关键词
large language models,synthetic data,efficient
AI 理解论文
溯源树
样例
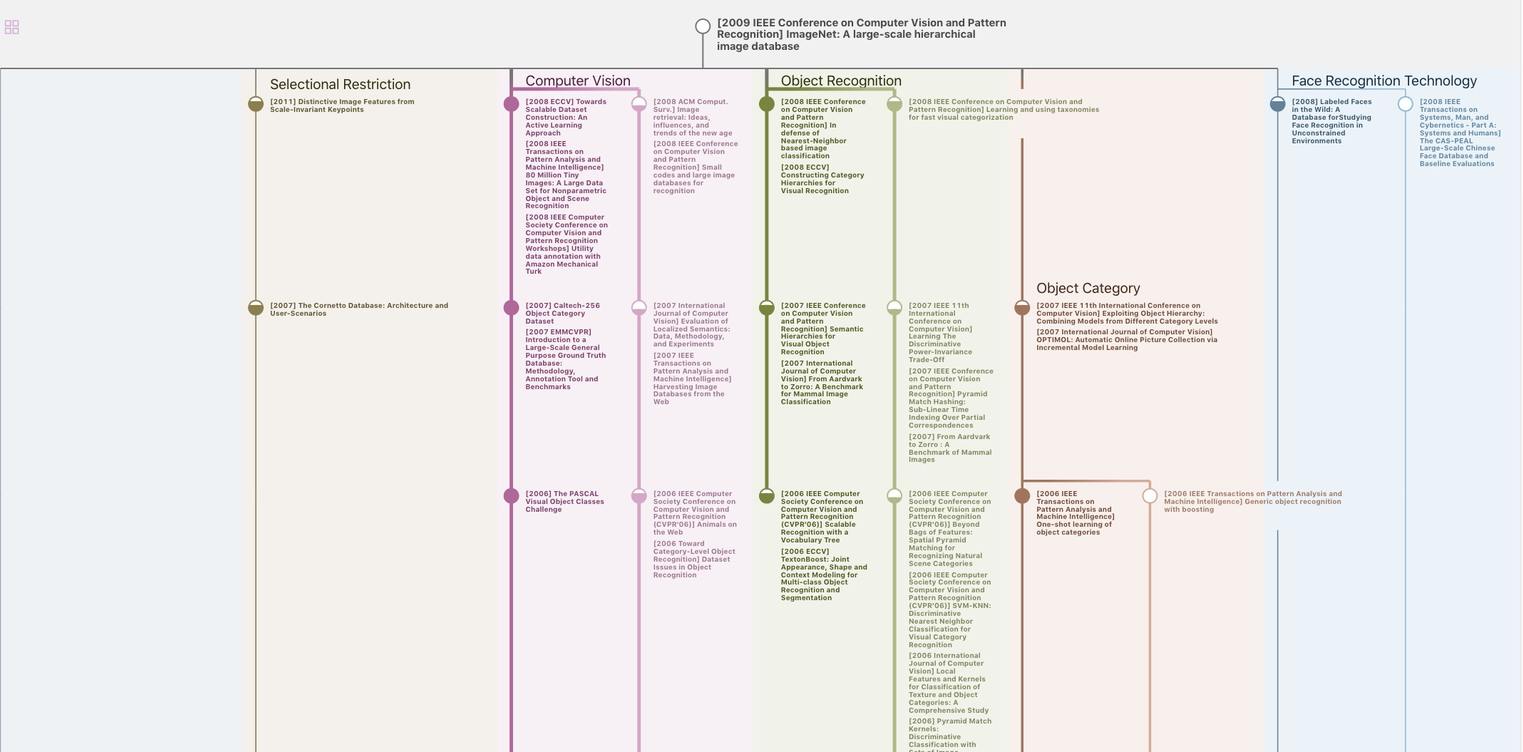
生成溯源树,研究论文发展脉络
Chat Paper
正在生成论文摘要