Self-supervised Representation Learning From Random Data Projectors
ICLR 2024(2023)
摘要
Self-supervised representation learning~(SSRL) has advanced considerably by exploiting the transformation invariance assumption under artificially designed data augmentations. While augmentation-based SSRL algorithms push the boundaries of performance in computer vision and natural language processing, they are often not directly applicable to other data modalities, and can conflict with application-specific data augmentation constraints. This paper presents an SSRL approach that can be applied to any data modality and network architecture because it does not rely on augmentations or masking. Specifically, we show that high-quality data representations can be learned by reconstructing random data projections. We evaluate the proposed approach on a wide range of representation learning tasks that span diverse modalities and real-world applications. We show that it outperforms multiple state-of-the-art SSRL baselines. Due to its wide applicability and strong empirical results, we argue that learning from randomness is a fruitful research direction worthy of attention and further study.
更多查看译文
关键词
Representation learning,Self-supervised learning,random data projections,domain-agnostic representation learning
AI 理解论文
溯源树
样例
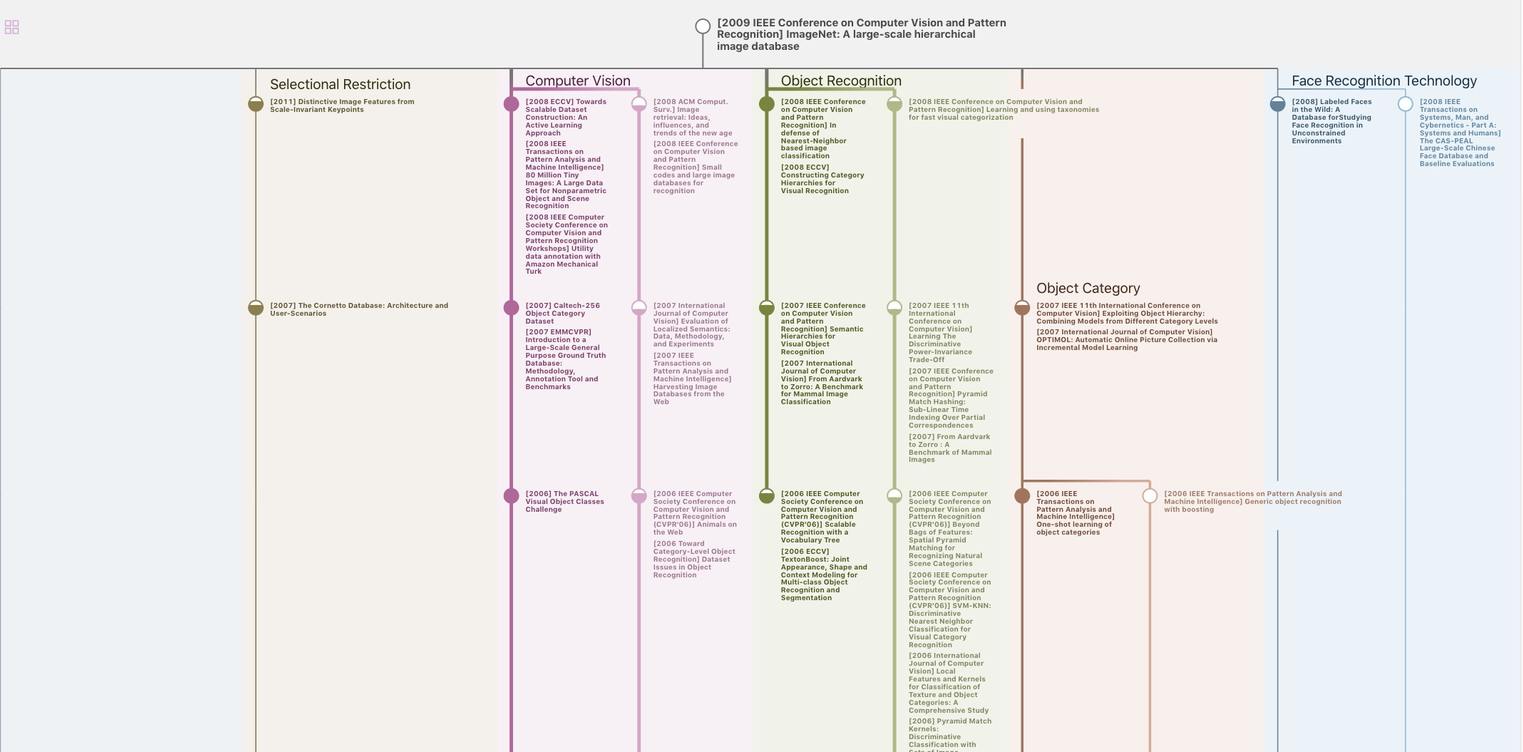
生成溯源树,研究论文发展脉络
Chat Paper
正在生成论文摘要