SVMDformer: A Semi-supervised Vehicular Misbehavior Detection Framework based on Transformer in IoV
2023 IEEE 43RD INTERNATIONAL CONFERENCE ON DISTRIBUTED COMPUTING SYSTEMS, ICDCS(2023)
摘要
Vehicle-to-everything(V2X) based technologies have become an important research topic to solve the problem of road congestion and traffic accidents. However, the rapid growth of connected vehicles enables massive messages to flow around in the Internet of Vehicles (IoV), some of which are misbehaving from insider attackers and thus threaten the security of IoV. Recently, some works have tried solving the misbehavior detection problem, but there are problems such as incomplete misbehavior types, inefficient multi-model detection, and insufficient accuracy. In this paper, we propose a Semi-supervised Vehicular Misbehavior Detection framework based on Transformer (SVMDformer), which transforms vehicular message sequences into misbehavior scores and identifies misbehavior by setting a threshold. The SVMDformer is trained in the cloud server and deployed in the edge server to detect misbehavior by incoming vehicular messages in IoV. Based on an open-source dataset, we demonstrate the model's performance in different environments, analyze its sensitivity, and compare it with baselines. The proposed SVMDformer is more versatile, accurate, and comprehensive than prior works. It is a single model that can detect 19 misbehaviors for vehicular message sequences. It achieves an AUC of 99.87%, better accuracy of 99.66%, better precision of 99.68%, better F1-score of 99.66%, and a false detection rate of 0.34% which is over three times lower than the state-of-the-art (SOTA) work.
更多查看译文
关键词
IoV,Vehicular Misbehavior Detection,Semi-supervised Learning,Transformer
AI 理解论文
溯源树
样例
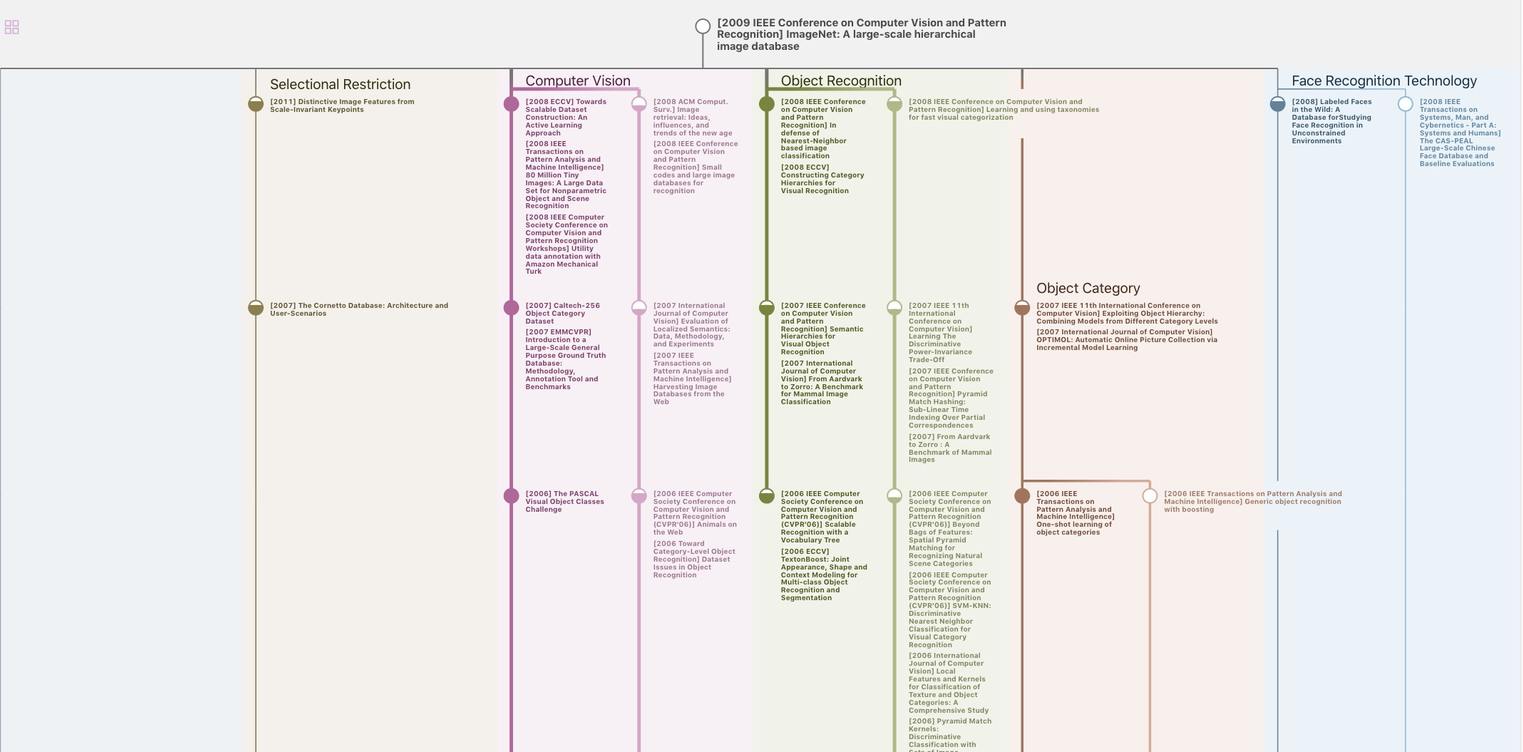
生成溯源树,研究论文发展脉络
Chat Paper
正在生成论文摘要