Hierarchical Federated Learning with Adaptive Momentum in Multi-Tier Networks
2023 IEEE 43rd International Conference on Distributed Computing Systems (ICDCS)(2023)
摘要
In this paper, we propose and analyze HierAdMo, a three-tier adaptive momentum accelerated client-edge-cloud Federated Learning (FL) algorithm. HierAdMo combines the momentum acceleration on both worker and edge levels. However, simply combining these two levels of momenta may lead to disagreement between them, negatively influencing convergence performance. To this end, we embed an online adaptive method that scales down the momentum when disagreement occurs. We provide mathematical proof for the convergence of HierAdMo for non-i.i.d. data and the tighter convergence upper bound compared with a version of HierAdMo without adaptation (HierAdMo-R). Finally, extensive experiments based on real-world datasets are conducted, verifying that HierAdMo outperforms existing mainstream benchmarks and achieves the optimal or near-optimal convergence performance compared with HierAdMo-R under a wide range of settings.
更多查看译文
关键词
wireless computing,federated learning,edge computing,momentum
AI 理解论文
溯源树
样例
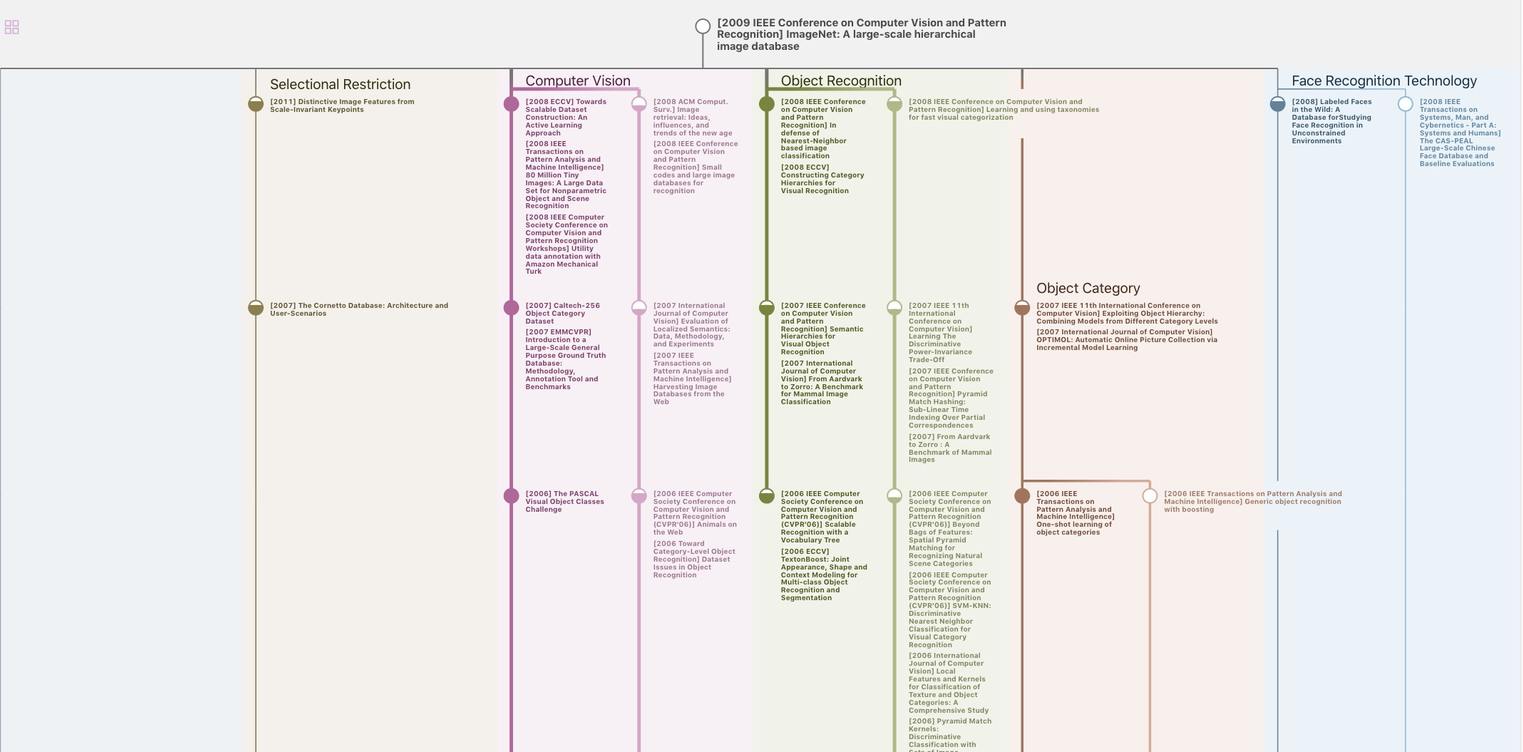
生成溯源树,研究论文发展脉络
Chat Paper
正在生成论文摘要