Survey on Factuality in Large Language Models: Knowledge, Retrieval and Domain-Specificity
CoRR(2023)
Abstract
This survey addresses the crucial issue of factuality in Large Language Models (LLMs). As LLMs find applications across diverse domains, the reliability and accuracy of their outputs become vital. We define the Factuality Issue as the probability of LLMs to produce content inconsistent with established facts. We first delve into the implications of these inaccuracies, highlighting the potential consequences and challenges posed by factual errors in LLM outputs. Subsequently, we analyze the mechanisms through which LLMs store and process facts, seeking the primary causes of factual errors. Our discussion then transitions to methodologies for evaluating LLM factuality, emphasizing key metrics, benchmarks, and studies. We further explore strategies for enhancing LLM factuality, including approaches tailored for specific domains. We focus two primary LLM configurations standalone LLMs and Retrieval-Augmented LLMs that utilizes external data, we detail their unique challenges and potential enhancements. Our survey offers a structured guide for researchers aiming to fortify the factual reliability of LLMs.
MoreTranslated text
Key words
factuality,large language models,retrieval,knowledge,domain-specificity
AI Read Science
Must-Reading Tree
Example
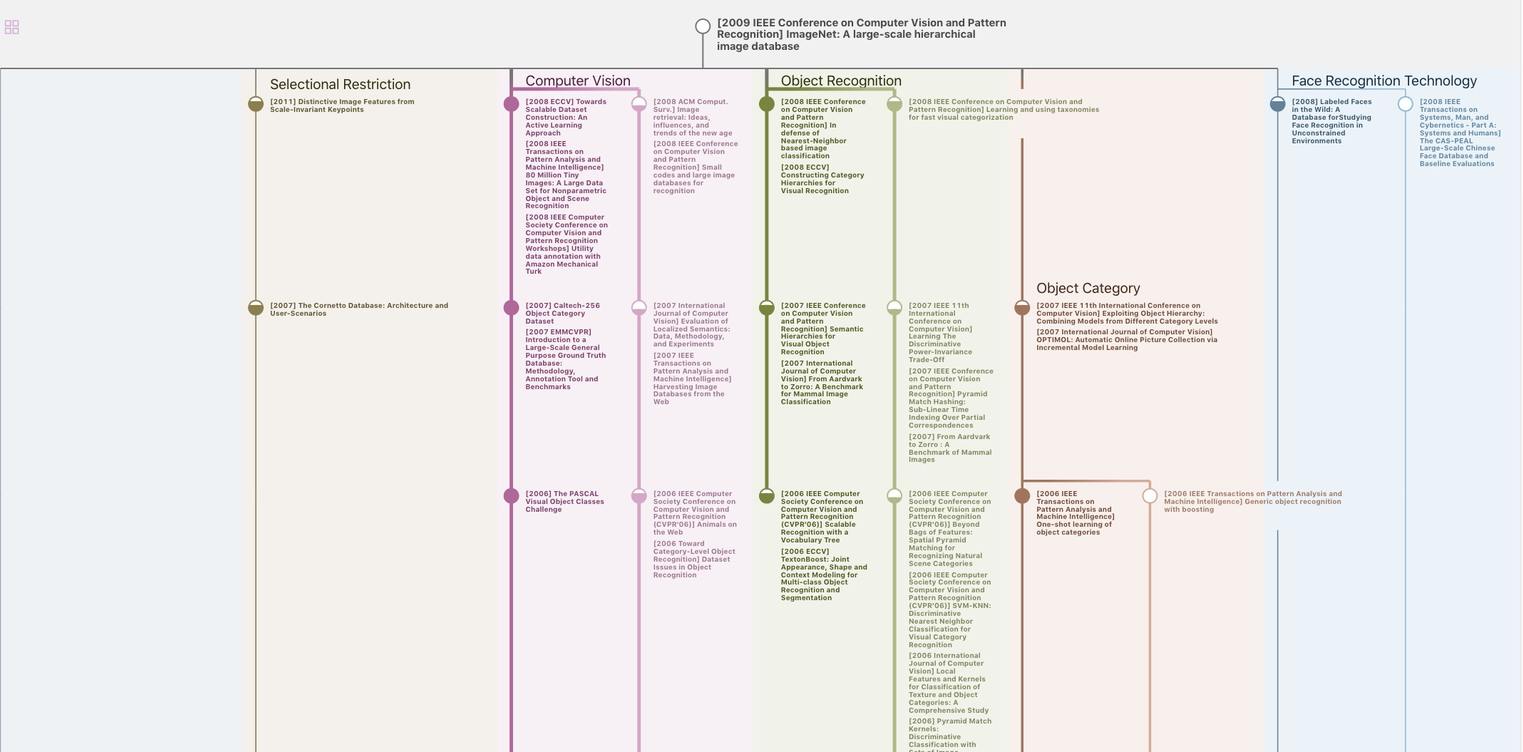
Generate MRT to find the research sequence of this paper
Chat Paper
Summary is being generated by the instructions you defined